Rethinking Robust Contrastive Learning from the Adversarial Perspective
arXiv (Cornell University)(2023)
摘要
To advance the understanding of robust deep learning, we delve into the effects of adversarial training on self-supervised and supervised contrastive learning alongside supervised learning. Our analysis uncovers significant disparities between adversarial and clean representations in standard-trained networks across various learning algorithms. Remarkably, adversarial training mitigates these disparities and fosters the convergence of representations toward a universal set, regardless of the learning scheme used. Additionally, increasing the similarity between adversarial and clean representations, particularly near the end of the network, enhances network robustness. These findings offer valuable insights for designing and training effective and robust deep learning networks. Our code is released at \textcolor{magenta}{\url{https://github.com/softsys4ai/CL-Robustness}}.
更多查看译文
关键词
robust contrastive learning,perspective
AI 理解论文
溯源树
样例
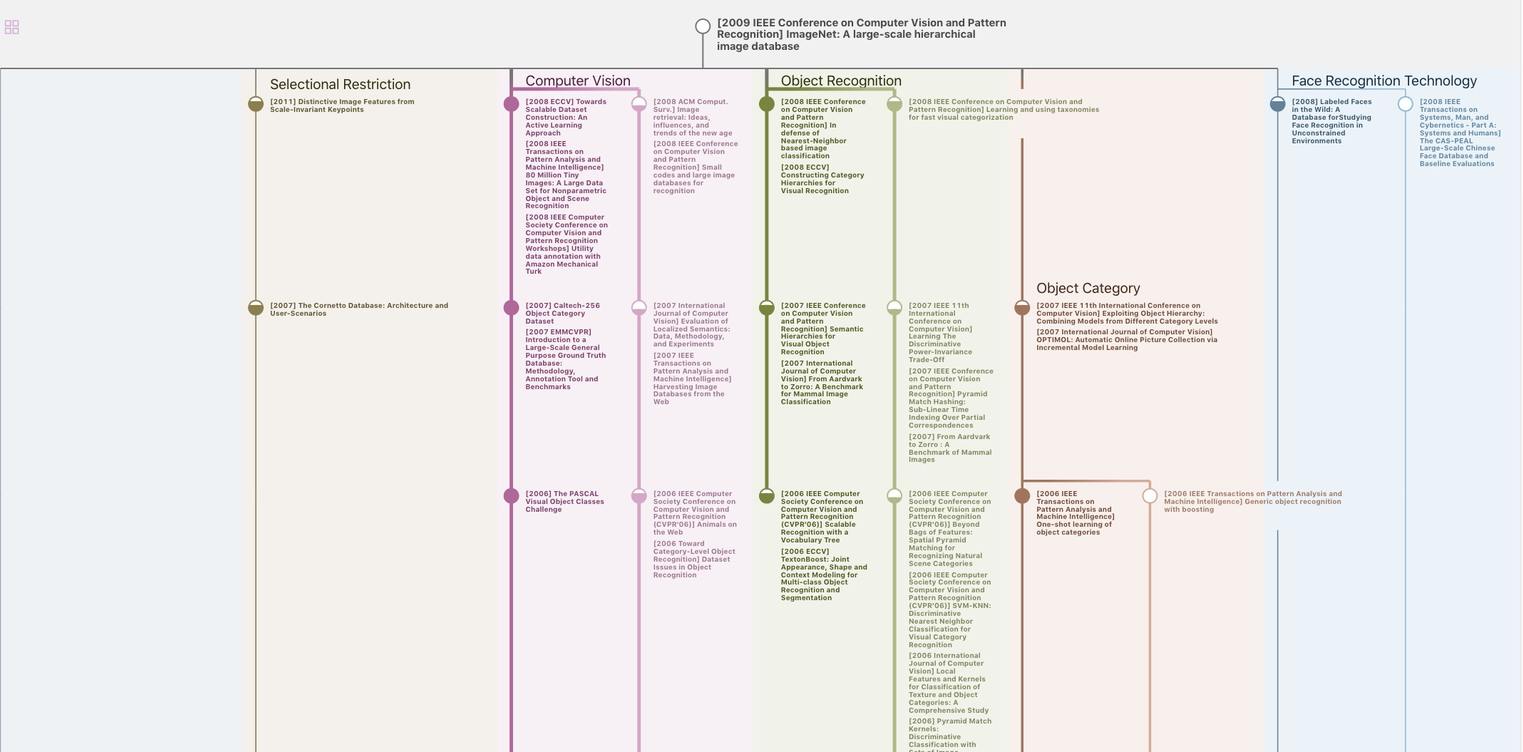
生成溯源树,研究论文发展脉络
Chat Paper
正在生成论文摘要