A cross-attention transformer encoder for paired sequence data
biorxiv(2023)
摘要
Transformer-based sequence encoding architectures are often limited to a single-sequence input while some tasks require a multi-sequence input. For example, the peptide-MHCII binding prediction task where the input consists of two protein sequences. Current workarounds to solve this input-type mismatch lack resemblance with the biological mechanisms behind the task. As a solution, we propose a novel cross-attention transformer encoder that creates a cross-attended embedding of both input sequences. We compare its classification performance on the peptide-MHCII binding prediction task to a baseline logistic regression model and a default transformer encoder. Finally, we make visualizations of the attention layers to show how the different models learn different patterns.
### Competing Interest Statement
KL and PM hold shares in ImmuneWatch BV, an immunoinformatics company.
更多查看译文
AI 理解论文
溯源树
样例
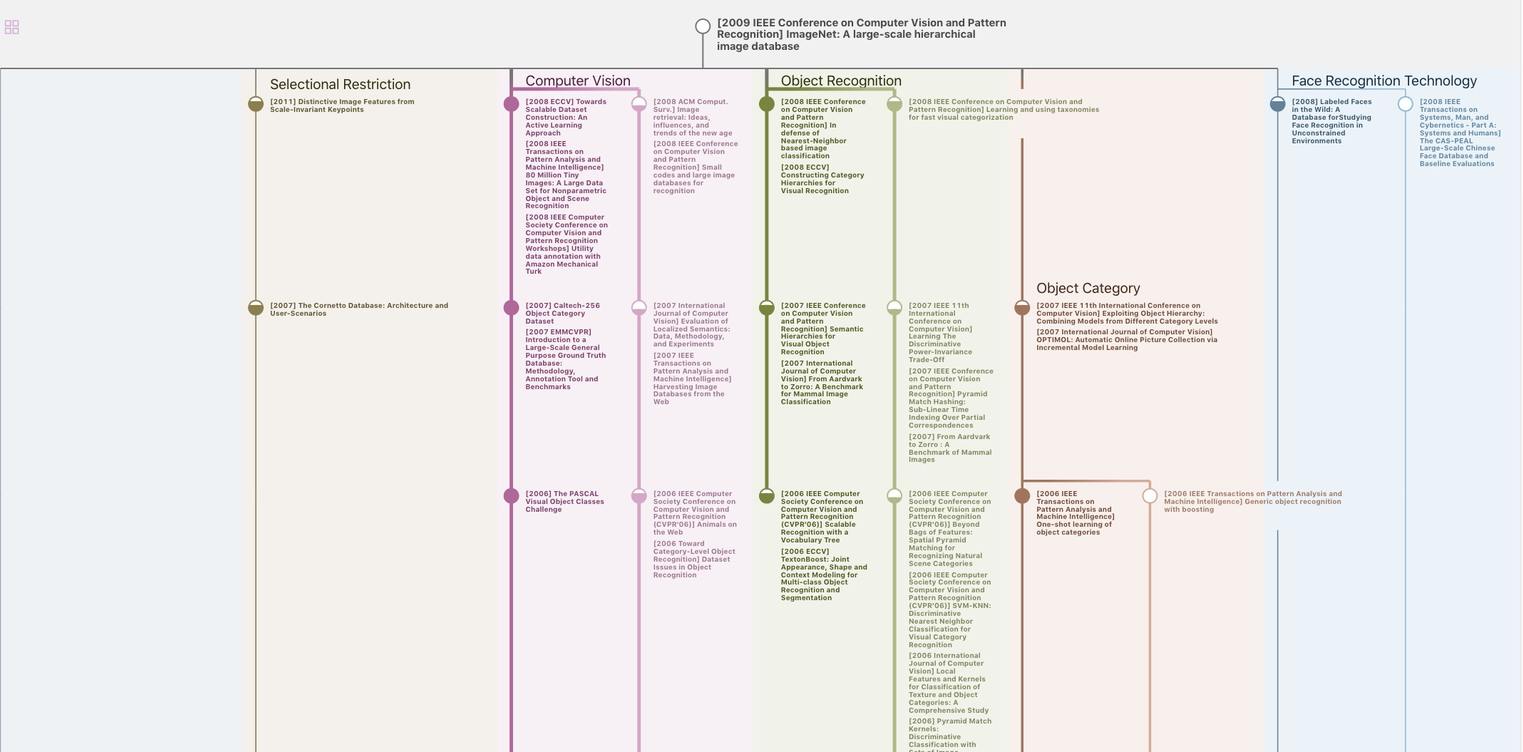
生成溯源树,研究论文发展脉络
Chat Paper
正在生成论文摘要