An INT8 Charge-Digital Hybrid Compute-In-Memory Macro With CNN-Friendly Shift-Feed Register Design.
IEEE Trans. Circuits Syst. II Express Briefs(2024)
摘要
As a novel computing architecture for Artificial Intelligence (AI), Compute-In-Memory (CIM) faces several challenges. On one hand, different computational paradigms pose a challenge in achieving both high area efficiency and inference accuracy. On the other hand, numerous on-chip buffers limit the potential for further reduction in inference energy. This brief presents a Charge-Digital Hybrid CIM macro with a near-CIM CNN-friendly shift-feed register design aiming to enhance energy and area efficiency while maintaining high inference accuracy. The macro is silicon-verified on 22nm FDSOI process, achieving 38.6 52.3 TOPS/W energy efficiency and 21.7 TOPS/mm2 throughput with an accuracy of 93.27% for CIFAR-10 and 71.92% for CIFAR-100. The shift-feed register is also evaluated demonstrating a 66% inference energy reduction on ResNet18.conv2 layer with a small area overhead.
更多查看译文
关键词
Artificial Intelligence (AI),Compute-In-Memory (CIM),charge sharing,Convolutional Neural Network (CNN)
AI 理解论文
溯源树
样例
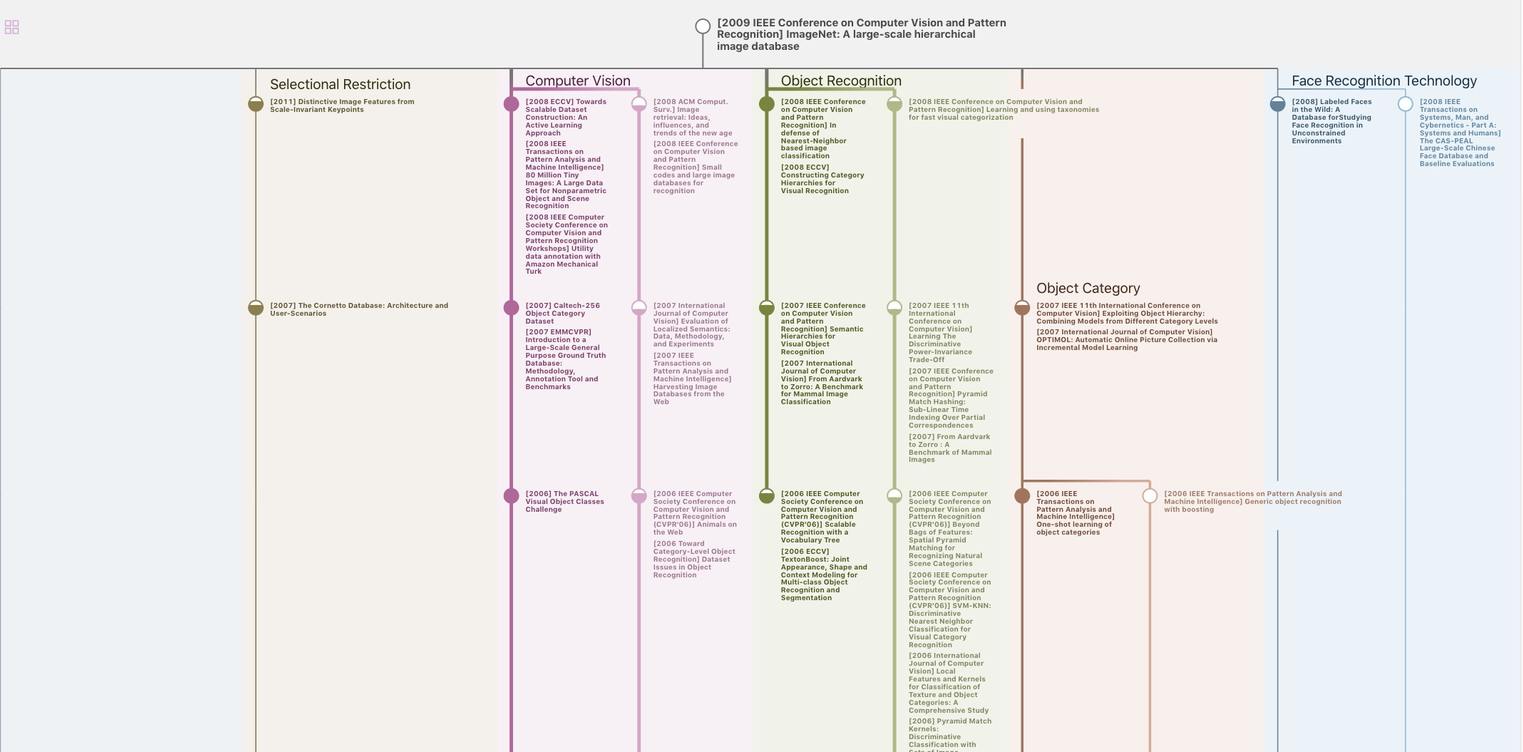
生成溯源树,研究论文发展脉络
Chat Paper
正在生成论文摘要