Prototype Contrastive Learning for Personalized Federated Learning
ARTIFICIAL NEURAL NETWORKS AND MACHINE LEARNING, ICANN 2023, PT III(2023)
摘要
Federated learning (FL) is a decentralized learning paradigm in which multiple clients collaborate to train the global model. However, the generalization of a global model is often affected by data heterogeneity. The goal of Personalized Federated Learning (PFL) is to develop models tailored to local tasks that overcomes data heterogeneity from the clients' perspective. In this paper, we introduce Prototype Contrastive Learning into FL (FedPCL) to learn a global base encoder, which aggregates knowledge learned by local models not only in the parameter space but also in the embedding space. Furthermore, given that some client resources are limited, we employ two prototype settings: multiple prototypes and a single prototype. The federated process combines with the Expectation Maximization (EM) algorithm. During the iterative process, clients perform the E-step to compute prototypes and the M-step to update model parameters by minimizing the ProtoNCE-M (ProtoNCE-S) loss. This process leads to achieving convergence of the global model. Subsequently, the global base encoder that extracts more compact representations is customized according to the local task to ensure personalization. Experimental results demonstrate the consistent increase in performance as well as its effective personalization ability.
更多查看译文
关键词
Contrastive Learning,Prototype,Embedding Space
AI 理解论文
溯源树
样例
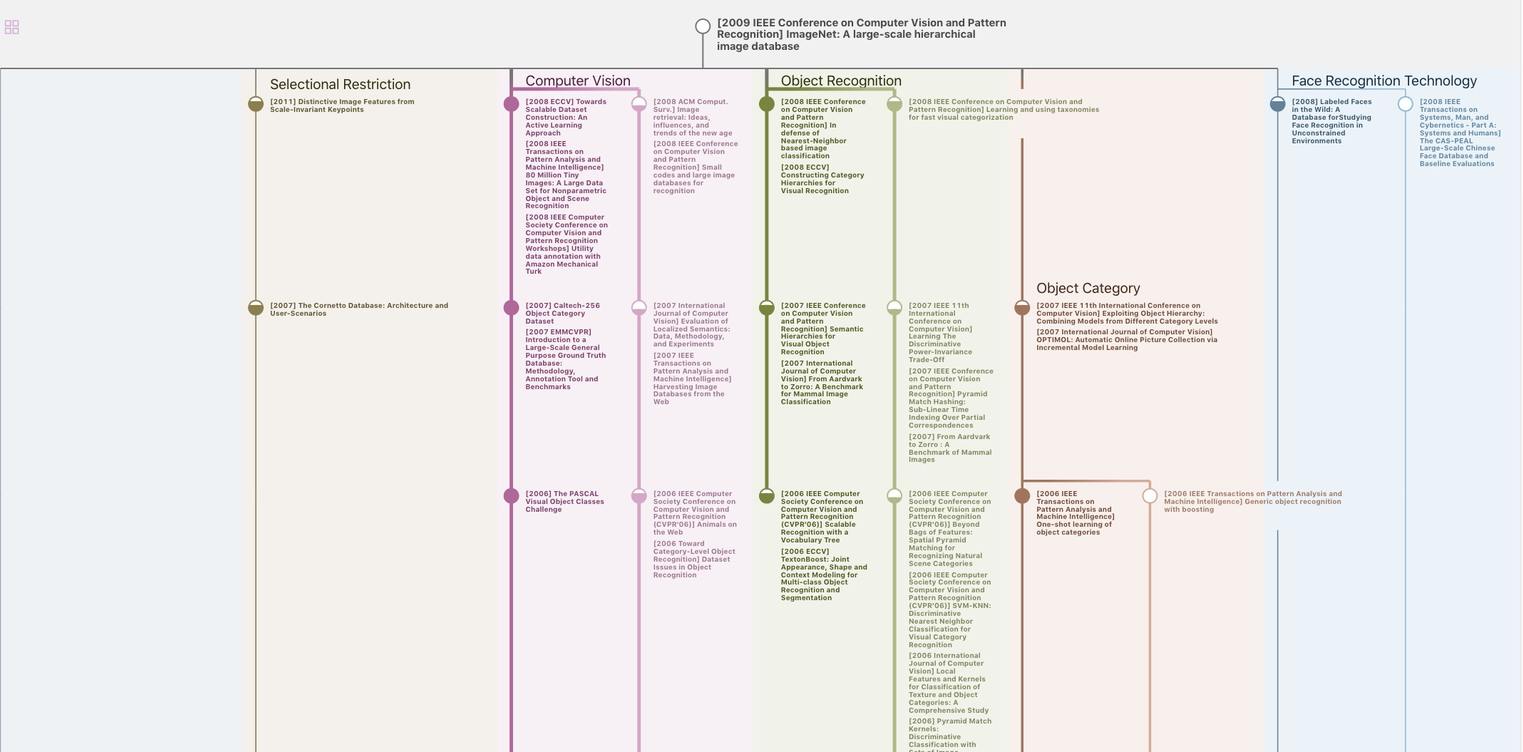
生成溯源树,研究论文发展脉络
Chat Paper
正在生成论文摘要