PTSTEP: Prompt Tuning for Semantic Typing of Event Processes
ARTIFICIAL NEURAL NETWORKS AND MACHINE LEARNING, ICANN 2023, PT III(2023)
摘要
Giving machines the ability to understand the intent of human actions is a basic goal of Natural Language Understanding. In the context of that, a task called the Multi-axis Event Processes Typing is proposed, which aims to comprehend the overall goal of an event sequence from the aspect of action and object. Existing works utilize fine-tuning to mine the semantic information of the event processes in the pre-trained language models and achieve good performance. Prompt tuning is effective in fully exploiting the capabilities of pre-trained language models. To mine more sufficient semantic information of the event process, it is crucial to utilize appropriate prompts to guide the pre-trained language models. Moreover, most existing prompt tuning methods use unified prompt encodings. Due to the complex correlations between events of event processes, it is hard to capture context-sensitive semantic information of the event processes. In this paper, we propose PTSTEP, an encoder-decoder based method with continuous prompts. Specifically, we propose a context-aware prompt encoder to obtain a more expressive continuous prompt. Parameters in the pre-trained language model are fixed. On the encoder, the continuous prompt guide the model to mine more semantic information of the event process. On the decoder, the context-aware continuous prompt guide the model to better understand the event processes. PTSTEP outperforms the state-of-the-art method by 0.82% and 3.74% respectively on action MRR and object MRR. The significant improvements prove the effectiveness of our method.
更多查看译文
关键词
Event processes understanding,Semantic Typing,Prompt Tuning
AI 理解论文
溯源树
样例
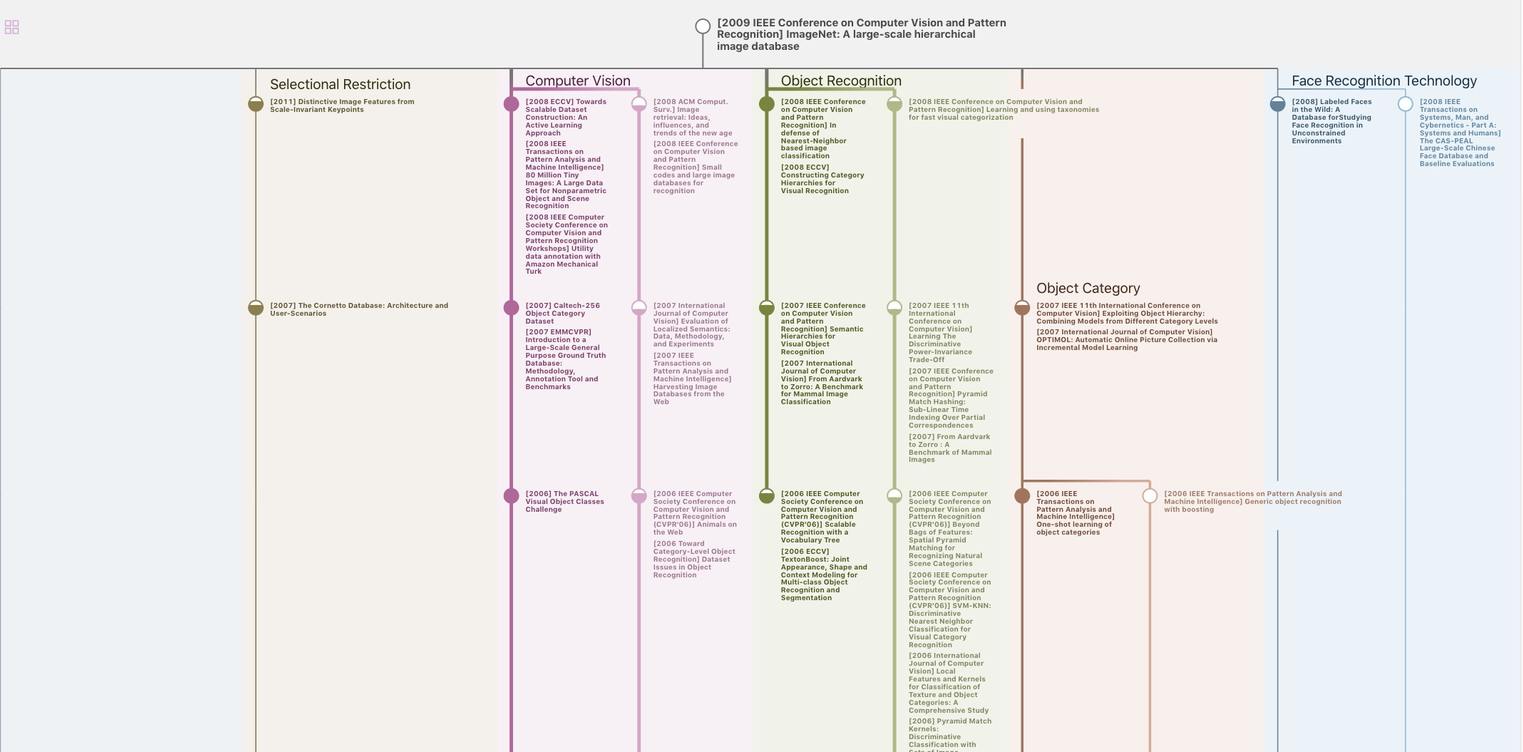
生成溯源树,研究论文发展脉络
Chat Paper
正在生成论文摘要