Taper Residual Dense Network for Audio Super-Resolution
Lecture Notes in Computer Science(2023)
摘要
Audio Super-Resolution (Audio SR) aims to seek a mapping method from low-resolution audio to high-resolution audio, in which deep-learning-based approaches have achieved encouraging results in recent years. However, most Audio SR models do not break out of the limitations of the framework of UNet, which only have skip connections between the corresponding encoders and decoders. In this paper, we propose a taper residual dense network (TNet) for Audio SR. Specifically, 1) we introduce Residual Dense Block (RDB) and modify it to make it suitable for processing audio signals, which can fully utilize the features extracted by the network at different levels; 2) we design a taper network architecture that models and extracts features based on frequency-domain amplitude, frequency-domain phase as well as time-domain waveform in different lower branches, and then performs feature fusion in the upper branch. Experimental results show that our proposed TNet outperforms other baselines with fewer parameters.
更多查看译文
关键词
audio super resolution,deep learning,residual dense network
AI 理解论文
溯源树
样例
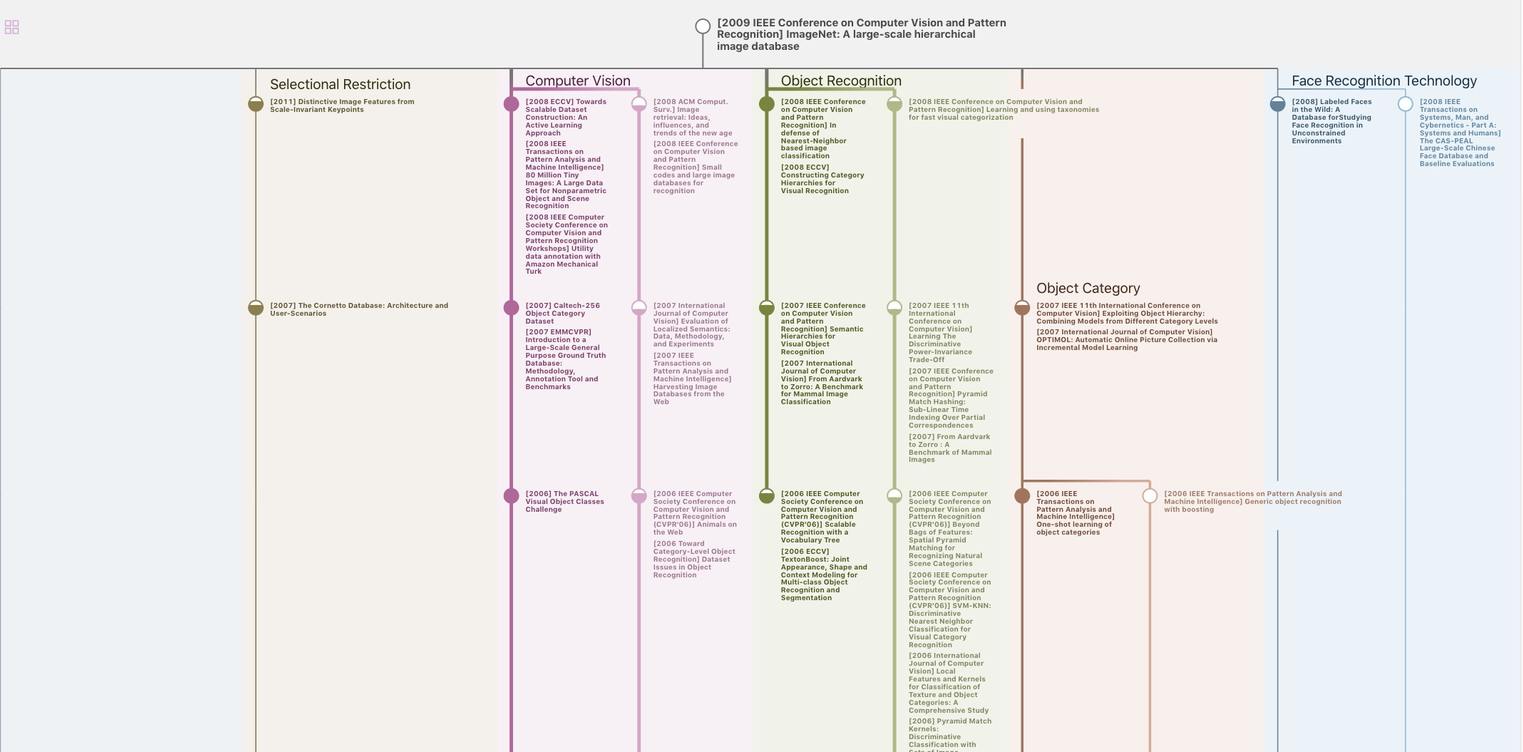
生成溯源树,研究论文发展脉络
Chat Paper
正在生成论文摘要