One Hip Wonder: 1D-CNNs Reduce Sensor Requirements for Everyday Gait Analysis
ARTIFICIAL NEURAL NETWORKS AND MACHINE LEARNING, ICANN 2023, PART X(2023)
摘要
Wearable inertial measurement units (IMU) enable largescale multicenter studies of everyday gait analysis in patients with rare neurodegenerative diseases such as cerebellar ataxia. To date, the quantity of sensors used in such studies has involved a trade-off between data quality and clinical feasibility. Here, we apply machine learning techniques to potentially reduce the number of sensors required for real-life gait analysis from three sensors to a single sensor on the hip. We trained 1D-CNNs on constrained walking data from individuals with cerebellar ataxia and healthy controls to generate synthetic foot data and predict gait features from a single sensor and tested them in free walking conditions, including the everyday life of unseen subjects. We compare 14 stride-based gait features (e.g. stride length) with three sensors (two on the feet and one on the hip) with our approach estimating the same features based on raw IMU-data from a single sensor placed on the hip. Leveraging layer-wise relevance propagation (LRP) and transfer learning, we determine driving elements of the input signals to predict individuals' gait features. Our approach achieved a relative error (< 5%) similar to the state of the art three-sensor approach. Thus, machine learning-assisted one-sensor systems can reduce the complexity and cost of gait analysis in upcoming clinical studies while maintaining clinical meaningful effect sizes.
更多查看译文
关键词
Clinical gait analysis,time-series,CNN,explainability,cerebellar ataxia
AI 理解论文
溯源树
样例
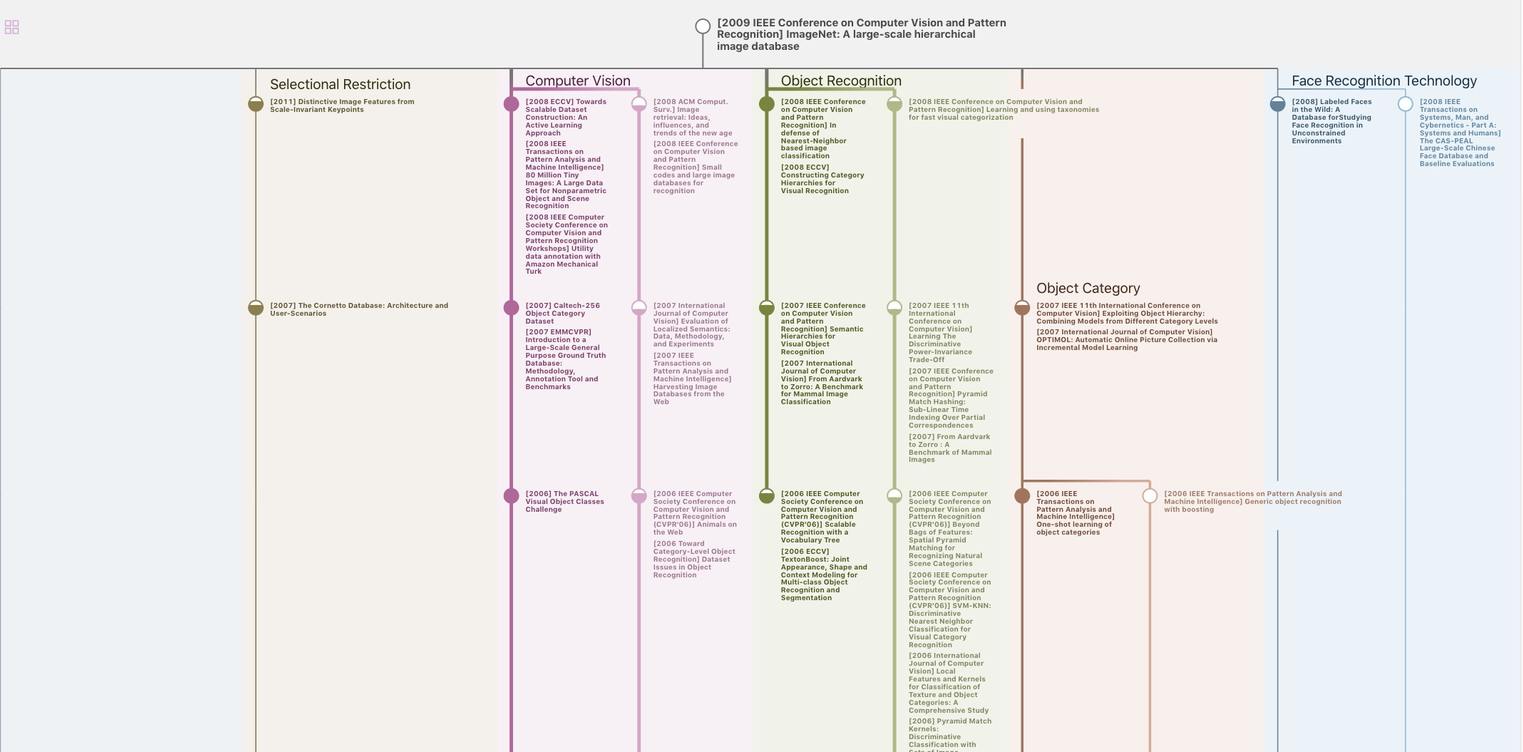
生成溯源树,研究论文发展脉络
Chat Paper
正在生成论文摘要