Efficient indoor localization with wireless sensor networks via learnable sparse coding
MEASUREMENT SCIENCE AND TECHNOLOGY(2023)
摘要
In this study, the indoor localization problem for human movement is built as a sparse representation, and a learnable sparse coding algorithm is proposed by introducing a nonconvex penalty function as the sparse constraints. The good properties of the penalty are able to help reduce the iteration cost to find a sparse solution indicating the target locations. The sparse code can achieve its optimal value from the trained neural network model with parametrization. It is instead of a piece of algorithmic processing in the conventional methods requiring a large iteration. A real-world experiment using a Bluetooth low energy wireless sensor network validates the proposed method and shows the improvement of the error reduction at the first five iteration points on average compared to iterative shrinkage and thresholding algorithm (ISTA), and achieves competitive performance in the localization tasks for learned-ISTA-based algorithms.
更多查看译文
关键词
wireless sensor network,non-convex optimization,sparse coding,learnable algorithm,neural network
AI 理解论文
溯源树
样例
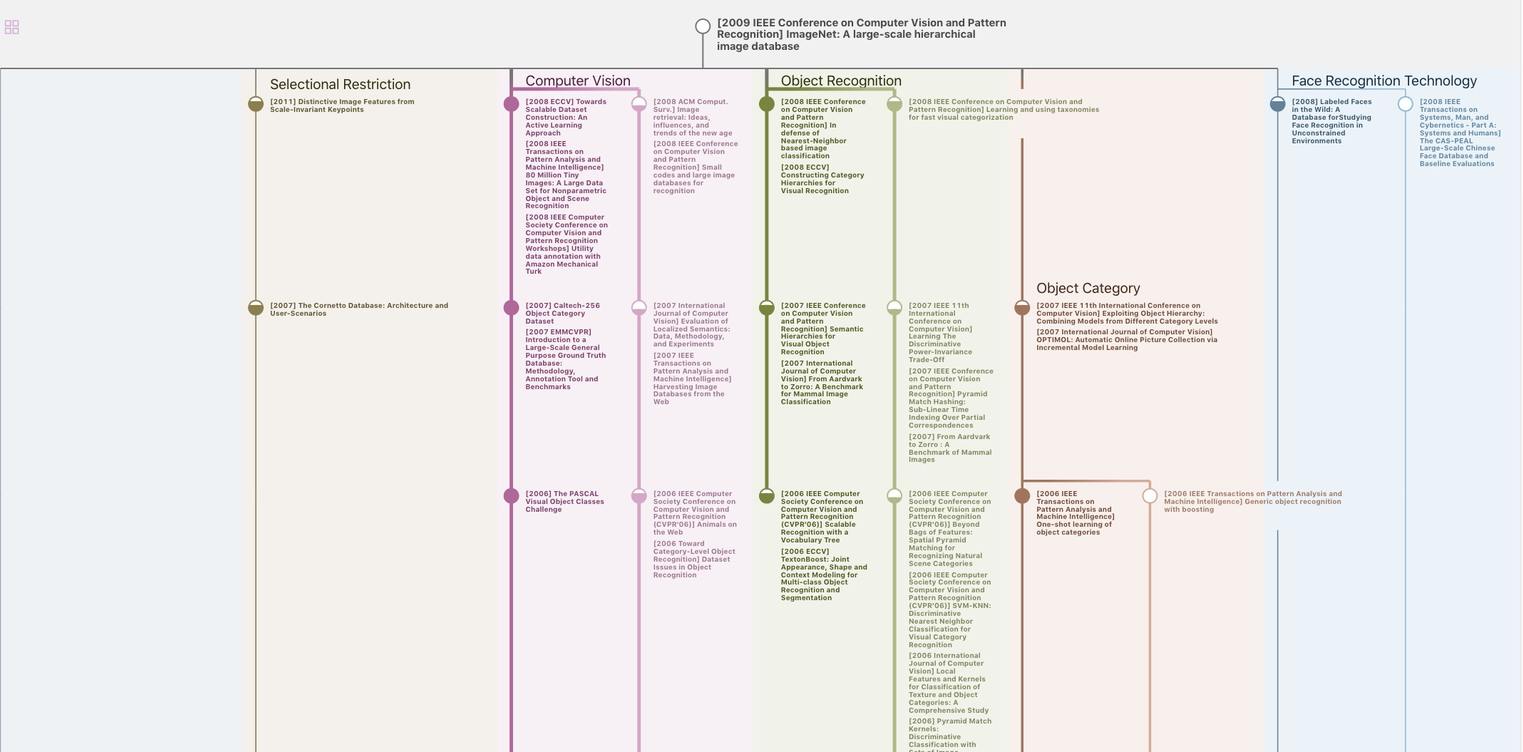
生成溯源树,研究论文发展脉络
Chat Paper
正在生成论文摘要