A Big Data Processing Technique Based on Tikhonov Regularization
Mathematics for industry(2023)
摘要
We propose a big data processing technique that can reconstruct a function and its derivative from scattered data with random noise. The main idea is to make use of the data amount and its statistical property to reduce the random error, while using a relatively small and proper number of nodes for interpolation. Tikhonov regularization is adopted to obtain stable and reliable reconstructions of the function and its derivative. While the noise level remains large, reconstruction error becomes small as sample size increases. When a dataset is given, rigorous error bounds of function and derivative reconstruction in continuous $$L^2$$ norm are obtained using a histogram density estimator as indicator function. When observation points are sampled from a distribution, asymptotic convergence rate in probability is obtained as sample size tends to infinity.
更多查看译文
关键词
big data processing technique,tikhonov regularization,big data
AI 理解论文
溯源树
样例
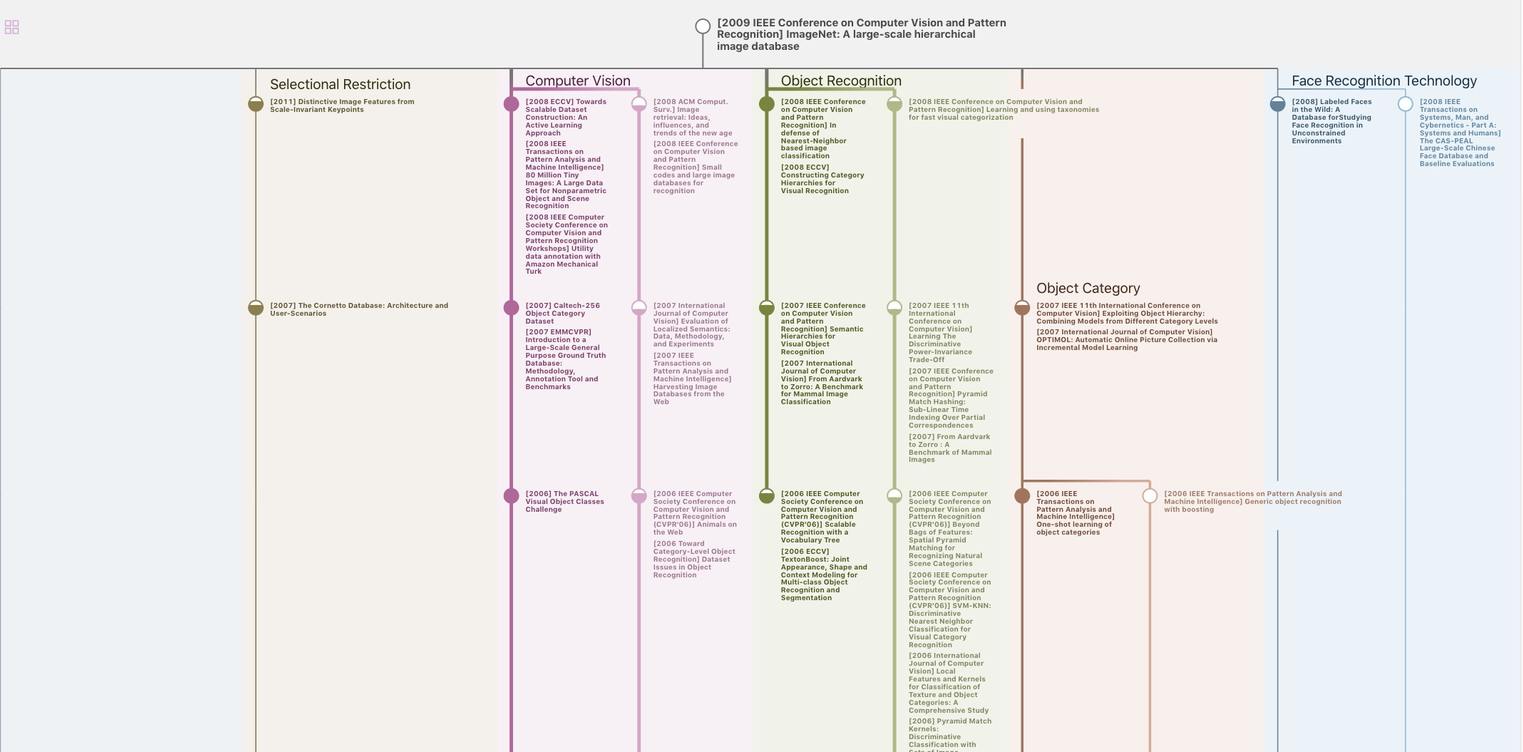
生成溯源树,研究论文发展脉络
Chat Paper
正在生成论文摘要