ECMT Framework for Internet of Things: An Integrative Approach Employing In-Memory Attribute Examination and Sophisticated Neural Network Architectures in Conjunction With Hybridized Machine Learning Methodologies.
IEEE Internet Things J.(2024)
摘要
With the proliferation of connected devices in the Internet of Things (IoT), cybersecurity threats have increased. Identifying malicious attacks in IoT requires advanced techniques tailored to this ecosystem. Existing algorithms have limited effectiveness in detecting obfuscated IoT malware. This study proposes the Elucidating Cybersecurity-promulgated Malware Taxonomy (ECMT) framework, combining memory analysis and ensemble machine learning, to enhance IoT malware categorization. ECMT integrates Support Vector Classification, Quadratic Discriminant Analysis, and AdaBoost on forensic artifacts from memory dumps to improve detection across families like ransomware, spyware, and trojans. ECMT can enable intrusion prevention, information protection, and cybercrime deterrence in IoT environments. Experiments on a balanced dataset indicate AdaBoost achieved 96% accuracy, demonstrating ECMT’s capabilities against complex IoT threats. The integrated approach provides automated, adaptable detection scalable to large IoT deployments through efficient linear models and robust ensemble learning. ECMT addresses concept drift and interpretability via retraining and explanation techniques. Results highlight advanced memory analysis and optimized machine learning classifiers as a promising solution for robust IoT malware detection despite adversaries’ evolving tactics. Further research can extend platform support, harden models against attacks, and refine streaming input. ECMT establishes a foundation for IoT security by unifying memory forensics, optimized neural architectures, and tailored ensemble learning.
更多查看译文
关键词
Machine Learning,Malware Classification,Feature Engineering,Cyber Security,Neural Networks
AI 理解论文
溯源树
样例
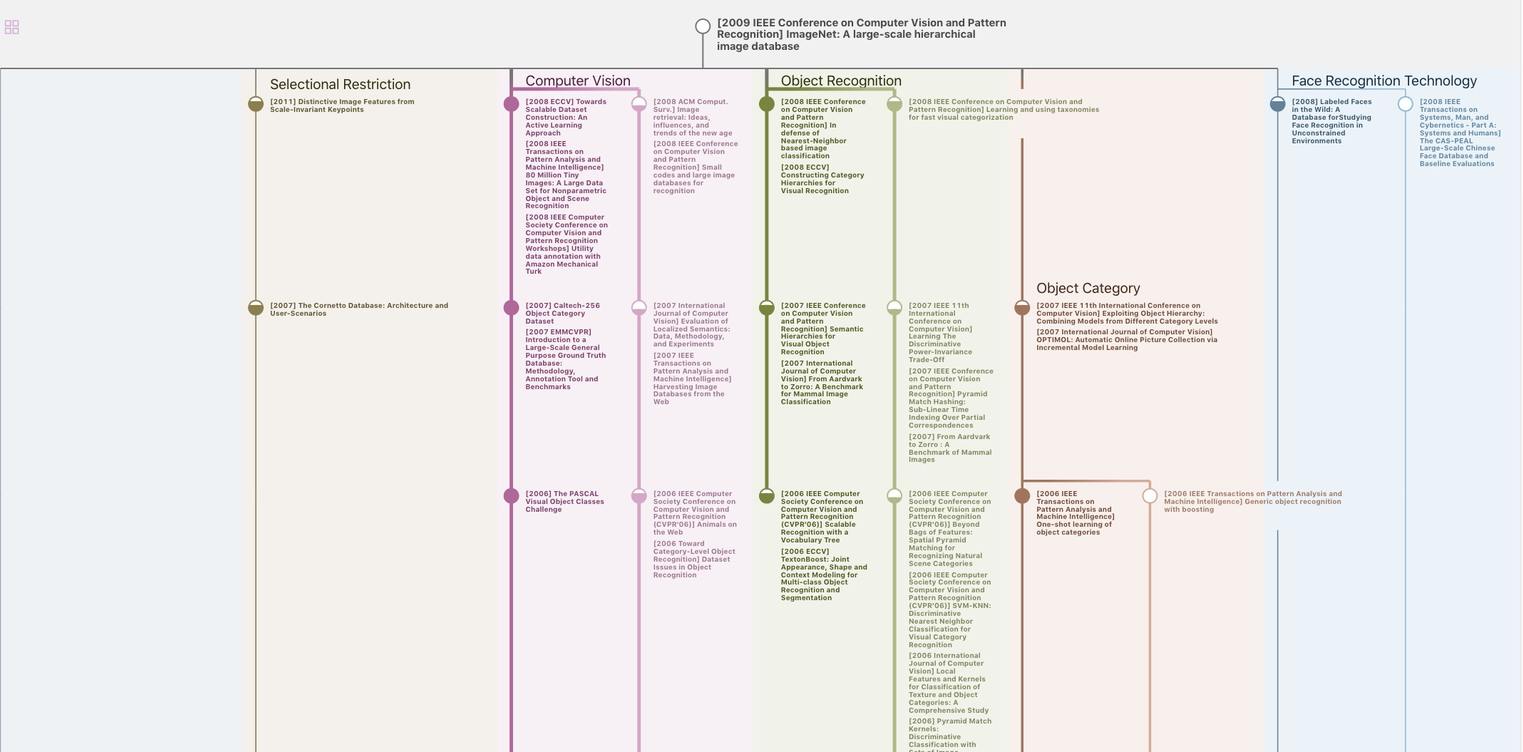
生成溯源树,研究论文发展脉络
Chat Paper
正在生成论文摘要