Self-supervised Video Representation Learning via Capturing Semantic Changes Indicated by Saccades
IEEE Transactions on Circuits and Systems for Video Technology(2023)
摘要
In this paper, we propose a self-supervised video representation learning (video SSL) method by taking inspiration from cognitive science and neuroscience on human visual perception. Different from previous methods that focus on the inherent properties of videos, we argue that humans learn to perceive the world through the self-awareness of the semantic changes or consistency in the input stimuli in the absence of labels, accompanied by representation reorganization during the post-learning rest periods. To this end, we first exploit the presence of saccades as an indicator of semantic changes in a contrastive learning framework, mimicking self-awareness in human representation learning. The saccades are generated by alternating the fixations following the predicted scanpath. Second, we model the semantic consistency in eye fixation by minimizing the prediction error between the predicted and the true state of another time point. Finally, we incorporate prototypical contrastive learning to reorganize the learned representations to enhance the associations among perceptually similar ones. Compared to previous video SSL solutions, our method can capture finer-grained semantics from video instances and further associate similar ones together. Experiments show that the proposed bio-inspired video SSL method significantly improves the Top-1 video retrieval accuracy on UCF101 and achieves superior performance on downstream tasks such as action recognition under comparable settings.
更多查看译文
关键词
Self-supervised learning,video self-supervised learning,representation learning,bio-inspired
AI 理解论文
溯源树
样例
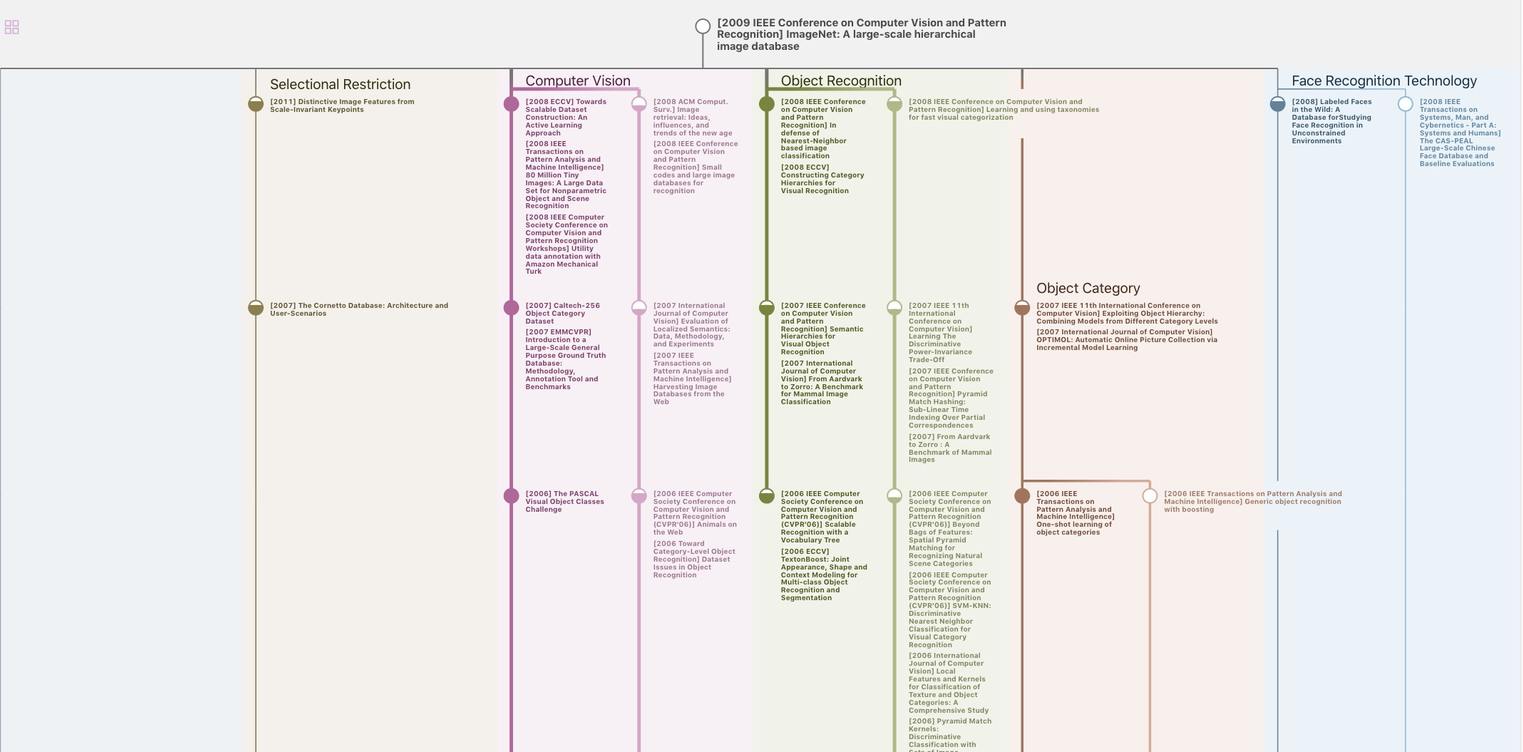
生成溯源树,研究论文发展脉络
Chat Paper
正在生成论文摘要