Abstract 5698: A multicenter study validated an integrated deep learning model for precision malignancy risk assessment and reducing unnecessary biopsies in BI-RADS 4 cases
Cancer Research(2023)
摘要
Introduction: BI-RADS category 4 is associated with a wide variability in probability of malignancy, ranging from 2 to 95% while biopsy-derived positive predictive value (PPV3) for this category’s lesions remains low at 21.1% in the US. A major fallout of these facts is that we have way very high false positive rate leading to too many unnecessary biopsies and their associated costs and emotional burden. We improved our in-house intelligent-augmented Breast cancer RISK calculator (iBRISK), an integrated deep learning (DL) based decision support app and assessed its performance in a multicenter IRB-approved study. Methods: We improved iBRISK by retraining the DL model with an expanded dataset of 9,700 patient records of clinical risk-factors and mammographic descriptors from Houston Methodist Hospital (HMH) and validated using another 1,078 patient records. These patients were all seen between March 2006 and December 2016. We assessed the model using blinded, independent retrospective BI-RADS 4 patients who had biopsies subsequently after mammography and seen January 2015 - June 2019 at three major healthcare institutions in Texas, USA: MD Anderson Cancer Center, the University of Texas Health Sciences Center at San Antonio, and HMH. We dichotomized and trichotomized the data to evaluate precision of risk stratification and probability of malignancy (POM) estimation translated into biopsy decision augmentation. The iBRISK score as a continuous predictor of malignancy and possible cost savings was also analyzed. Results: The multicenter validation dataset had 4,209 women, median age (interquartile) was 56 (45, 65) years. The use of iBRISK score as a continuous predictor of malignancy yielded an AUC of 0.97. Among “low” and “moderate” POM patients, only two out of 1,228 patients (0.16%) and 118 out of 1788 (6.6%) were malignant respectively. This translates to an even better precision when compared to newly introduced BI-RADS 4 subcategories 4A and 4B, with associated PPV3s of 7.6% and 22%, respectively. The “high” POM group had a malignancy rate of 85.9% (1,025/1,193). Estimated potential cost savings in the US was over $260 million. Conclusion: The iBRISK app demonstrated high sensitivity in malignancy prediction and can potentially be used to safely obviate biopsies in up to 50% of patients in low/moderate POM-groups. This would result in significant healthcare quality improvement, cost savings, and help reduce patient anxiety. Citation Format: Chika F. Ezeana, Tiancheng He, Tejal A. Patel, Virginia Kaklamani, Maryam Elmi, Erica Ibarra, Pamela M. Otto, Kenneth A. Kist, Heather Speck, Lin Wang, Joe Ensor, Ya-Chen T. Shih, Bumyang Kim, I-Wen Pan, David Spak, Wei T. Yang, Jenny C. Chang, Stephen T. Wong. A multicenter study validated an integrated deep learning model for precision malignancy risk assessment and reducing unnecessary biopsies in BI-RADS 4 cases. [abstract]. In: Proceedings of the American Association for Cancer Research Annual Meeting 2023; Part 1 (Regular and Invited Abstracts); 2023 Apr 14-19; Orlando, FL. Philadelphia (PA): AACR; Cancer Res 2023;83(7_Suppl):Abstract nr 5698.
更多查看译文
关键词
precision malignancy risk assessment,integrated deep learning model,deep learning,risk assessment,bi-rads
AI 理解论文
溯源树
样例
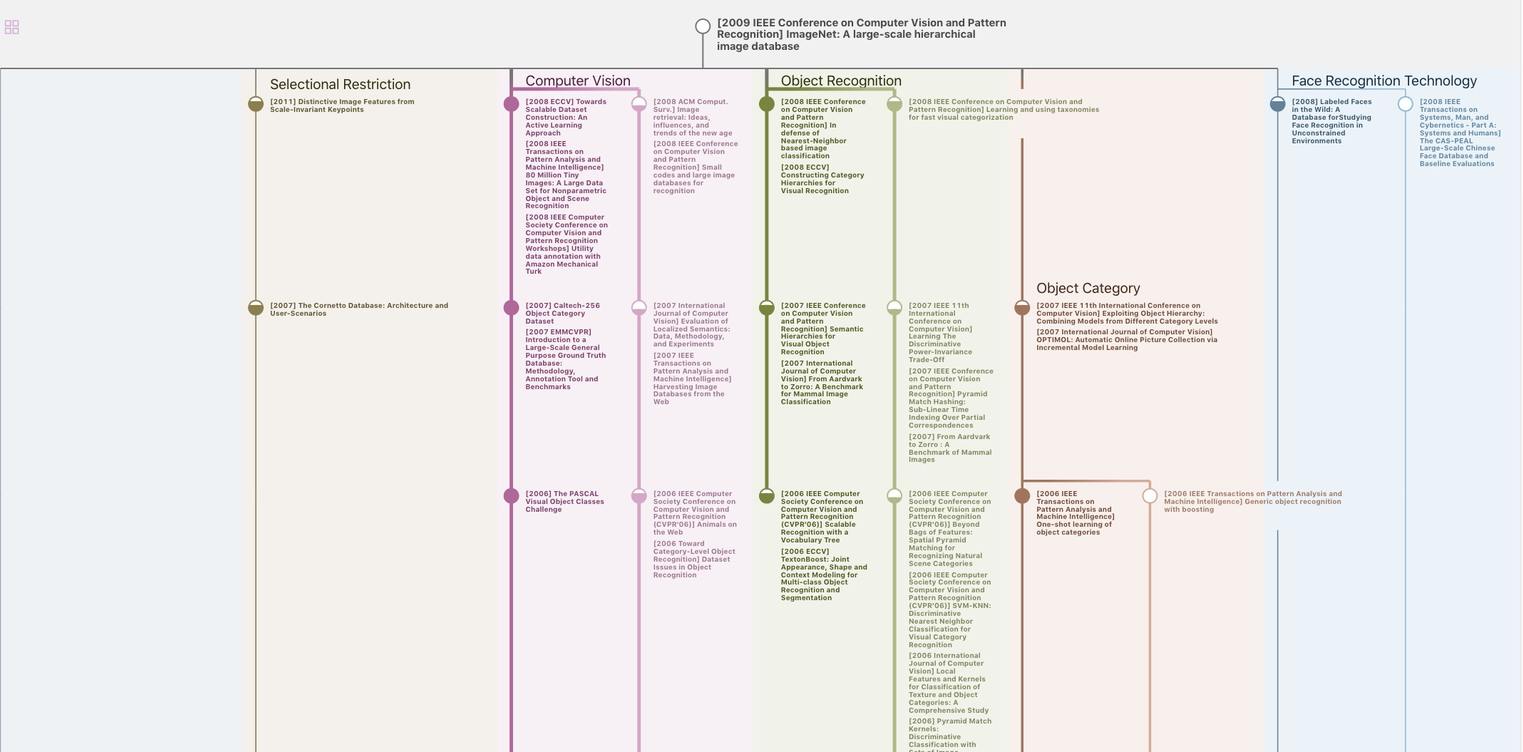
生成溯源树,研究论文发展脉络
Chat Paper
正在生成论文摘要