Imperceptible UAPs for Automatic Modulation Classification Based on Deep Learning
IEEE TRANSACTIONS ON CIRCUITS AND SYSTEMS II-EXPRESS BRIEFS(2024)
摘要
Automatic Modulation Classification (AMC), which based on deep learning has been extensively researched and implemented in wireless communication systems. Universal adversarial perturbation refers to a single perturbation that can cause most samples to be misclassified by deep learning models. In this brief, we aim to achieve imperceptible universal adversarial attacks on AMC models, and thus an imperceptible universal adversarial perturbations (imperceptible UAPs) framework was proposed. Specifically, loss functions were separately designed for target signals and non -target signals, and then a total loss was calculated. This total loss function can be modified by hyperparameters to achieve class -specific universal adversarial attacks (CUAAs), class -discriminative universal adversarial attacks (CD-UAAs), and class -discriminative target universal adversarial attacks (CD-TUAAs). Meanwhile, wavelet reconstruction was applied to the training data, thus further improving the discriminability of the generated UAPs. After CUAAs were implemented, the class dominant in radio signals was visualized and analyzed by confusion matrices. Furthermore, with the confusion matrices of CUAAs, CD-TUAAs were efficiently implemented. The experiments were conducted on two radio signal datasets and models. In most scenarios, CD-UAAs achieved excellent performance with an average Delta A(cc) of 58.20% and CD-TUAAs achieved an average Delta K of 73.78%.
更多查看译文
关键词
Perturbation methods,Modulation,Wireless communication,Training,Signal to noise ratio,Image reconstruction,Deep learning,Automatic modulation classification,class discriminative universal adversarial attack,wireless security
AI 理解论文
溯源树
样例
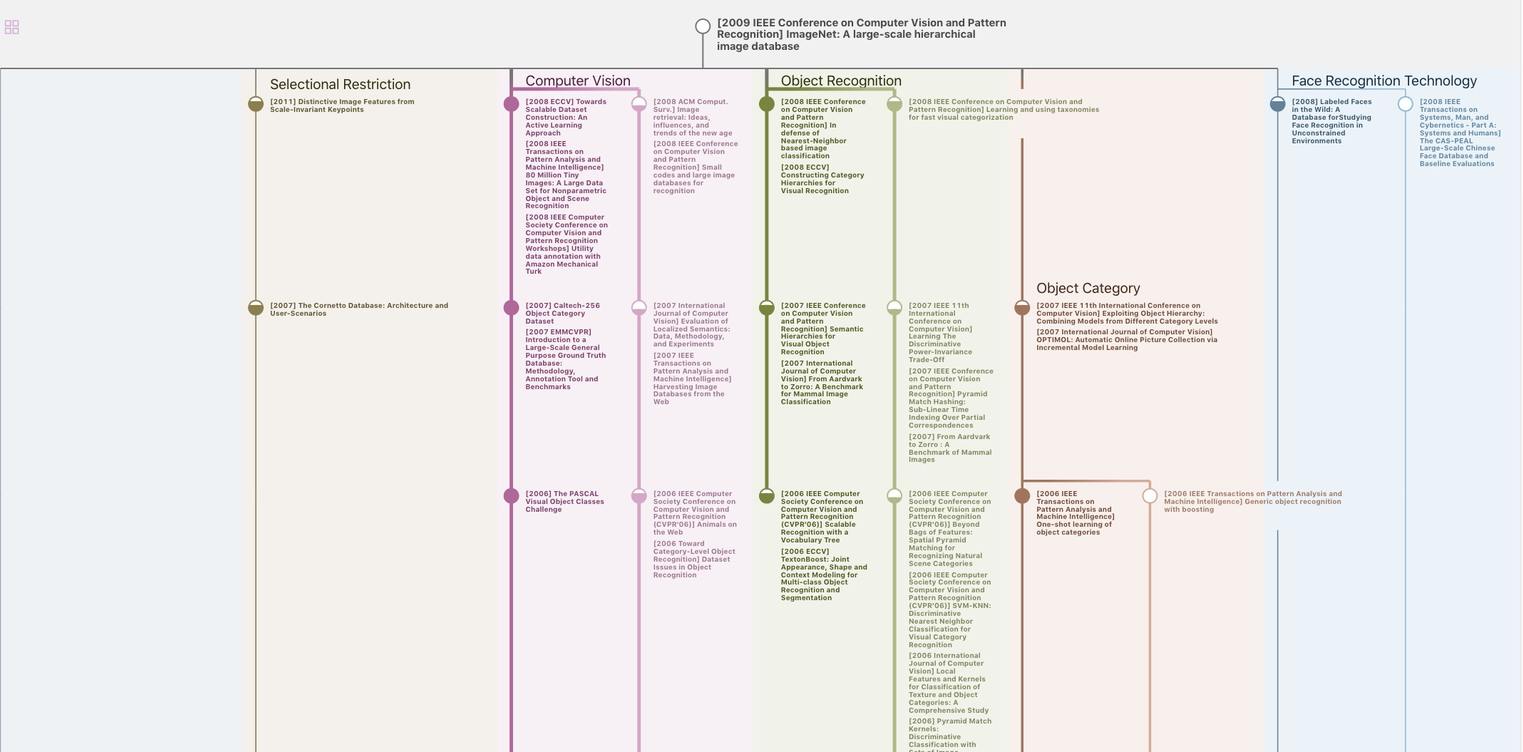
生成溯源树,研究论文发展脉络
Chat Paper
正在生成论文摘要