Hyperspectral Blind Unmixing Using a Double Deep Image Prior
IEEE transactions on neural networks and learning systems(2023)
摘要
With the rise of machine learning, hyperspectral image (HSI) unmixing problems have been tackled using learning-based methods. However, physically meaningful unmixing results are not guaranteed without proper guidance. In this work, we propose an unsupervised framework inspired by deep image prior (DIP) that can be used for both linear and nonlinear blind unmixing models. The framework consists of three modules: 1) an Endmember estimation module using DIP (EDIP); 2) an Abundance estimation module using DIP (ADIP); and 3) a mixing module (MM). EDIP and ADIP modules generate endmembers and abundances, respectively, while MM produces a reconstruction of the HSI observations based on the postulated unmixing model. We introduce a composite loss function that applies to both linear and nonlinear unmixing models to generate meaningful unmixing results. In addition, we propose an adaptive loss weight strategy for better unmixing results in nonlinear mixing scenarios. The proposed methods outperform state-of-the-art unmixing algorithms in extensive experiments conducted on both synthetic and real datasets.
更多查看译文
关键词
Convolutional neural networks (CNNs),deep image prior (DIP),hyperspectral image (HSI),linear unmixing,nonlinear unmixing
AI 理解论文
溯源树
样例
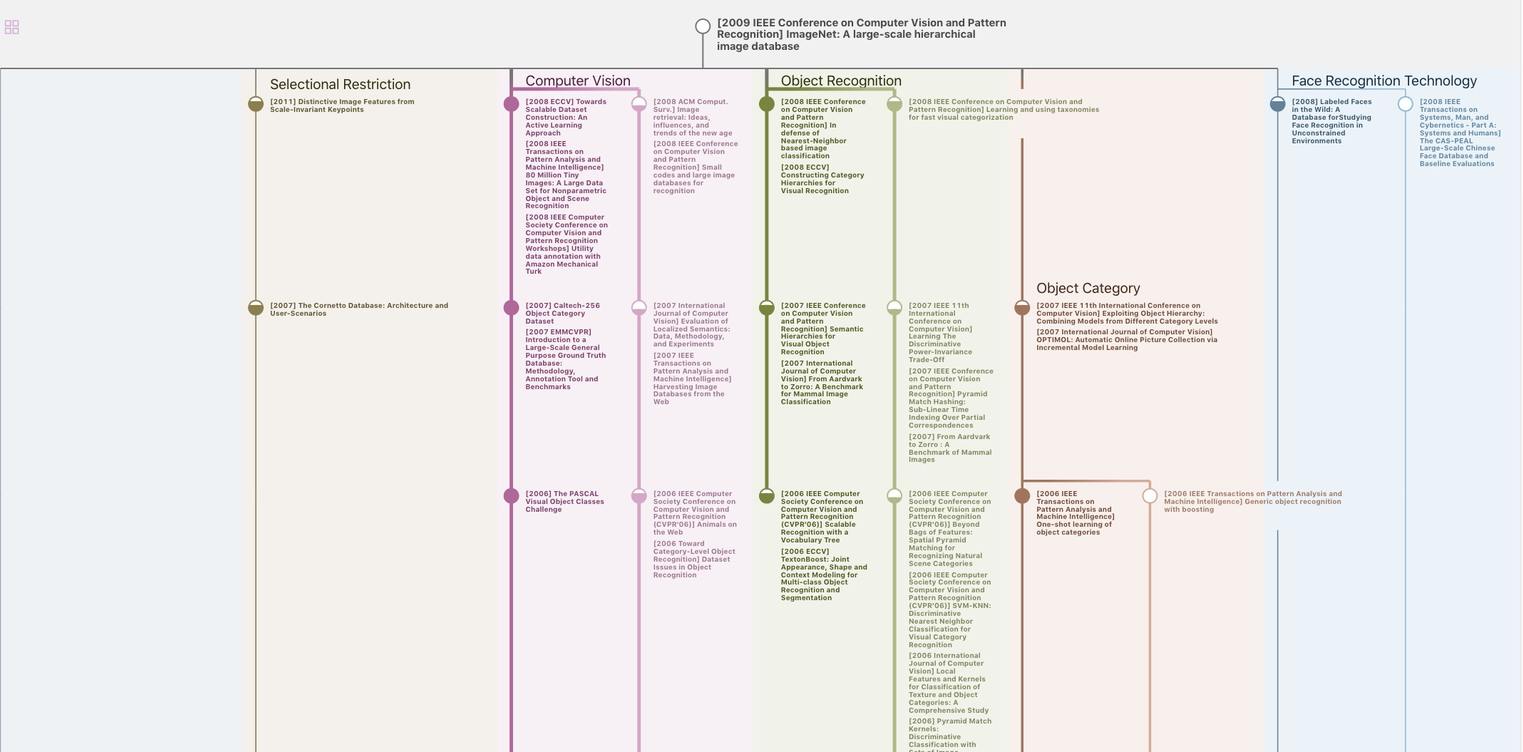
生成溯源树,研究论文发展脉络
Chat Paper
正在生成论文摘要