Byzantine-robust decentralized stochastic optimization with stochastic gradient noise-independent learning error
SIGNAL PROCESSING(2024)
摘要
This paper studies Byzantine-robust stochastic optimization over a decentralized network, where every agent periodically communicates with its neighbors to exchange local models, and then updates its own local model with one or a mini-batch of local samples. The performance of such a method is affected by an unknown number of Byzantine agents, which conduct adversarially during the optimization process. To the best of our knowledge, there is no existing work that simultaneously achieves a linear convergence speed and a small learning error. We observe that the unsatisfactory trade -off between convergence speed and learning error is due to the intrinsic stochastic gradient noise. Motivated by this observation, we introduce two variance reduction methods, stochastic average gradient algorithm (SAGA) and loopless stochastic variance-reduced gradient (LSVRG), to Byzantine-robust decentralized stochastic optimization for eliminating the negative effect of the stochastic gradient noise. The two resulting methods, BRAVO-SAGA and BRAVO-LSVRG, enjoy both linear convergence speeds and stochastic gradient noise-independent learning errors. Such learning errors are optimal for a class of methods based on total variation (TV)-norm regularization and stochastic subgradient update. We conduct extensive numerical experiments to show their effectiveness under various Byzantine attacks.
更多查看译文
关键词
Decentralized stochastic optimization,Variance reduction,Byzantine-robustness
AI 理解论文
溯源树
样例
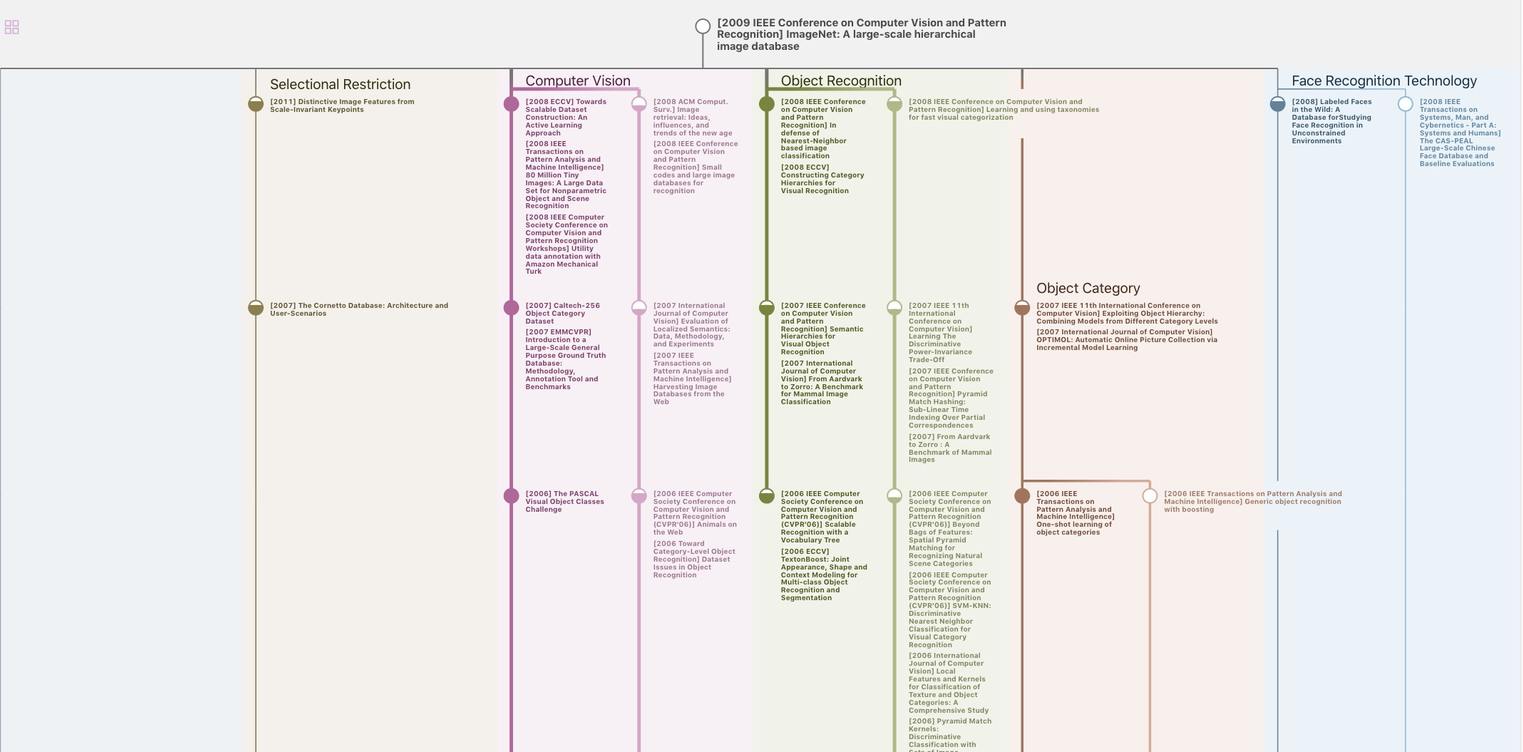
生成溯源树,研究论文发展脉络
Chat Paper
正在生成论文摘要