Efficient Model Extraction by Data Set Stealing, Balancing, and Filtering
IEEE INTERNET OF THINGS JOURNAL(2023)
摘要
Model extraction replicates the functionality of machine learning models deployed as a service. Recently, generative adversarial networks (GANs)-based methods have achieved remarkable performance in data-free model extraction. However, previous methods generate random data in every training batch, resulting in slow convergence and redundant queries. We propose to tackle the task with a much simpler paradigm. Specifically, we steal a data set with GAN before training the clone model rather than during every training batch. Benefiting from full use of the generated data, the proposed paradigm needs less training time and query cost. To improve the class distribution of data, a balancing strategy is applied. Furthermore, the balanced data set is filtered based on adversarial robustness for better quality. Combining the above strategies, we propose an efficient model extraction by data set stealing, balancing, and filtering (DSBF). Experiments on three widely used data sets show that DSBF outperforms previous methods while converging faster and costing fewer queries.
更多查看译文
关键词
Black-box,data-free,efficient,hard-label,model extraction
AI 理解论文
溯源树
样例
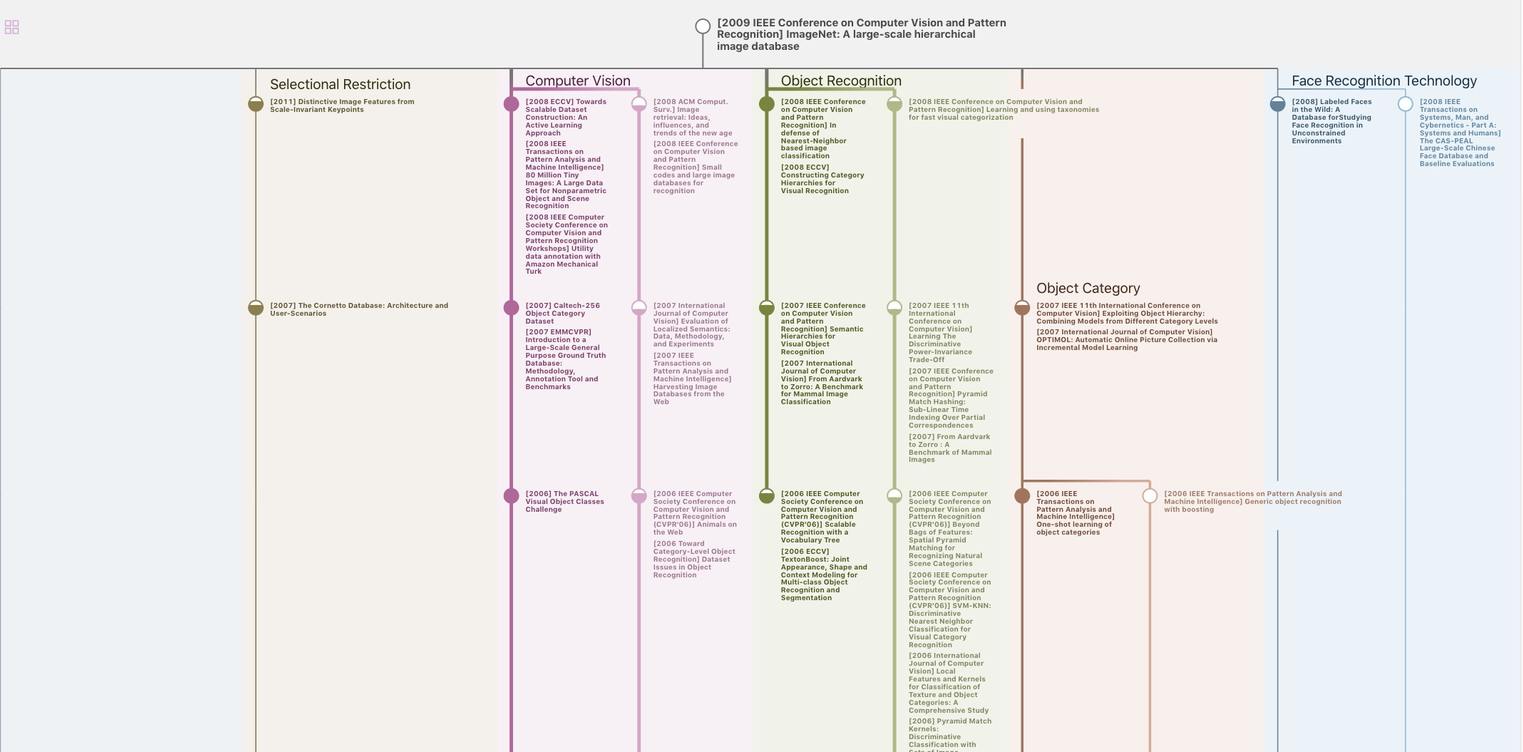
生成溯源树,研究论文发展脉络
Chat Paper
正在生成论文摘要