P629: the cll treatment infection model – clinical prospective validation as part of the prevent-acall trial
HemaSphere(2023)
摘要
Background: Infections are the major concern for patients with Chronic Lymphocytic Leukemia (CLL). Serious infections (1-month mortality rate: 10%) occur more frequently than progression to CLL treatment. We therefore developed the machine learning-based CLL Treatment Infection Model (CLL-TIM) to identify newly diagnosed patients with high risk of serious infections and/or early treatment (Agius et al., Nat Comm, 2020). Aims: To clinically validate the predictive performance of CLL-TIM identifying newly diagnosed patients with high risk of infection and/or treatment as part of the investigator-initiated phase 2 PreVent-ACaLL trial (NCT03868722). Methods: CLL patients were pre-screened by CLL-TIM after obtaining informed consent. CLL-TIM assessed risk based on +70 variables. Patients were predicted by CLL-TIM as high risk or low risk for the combined endpoint of serious infection and/or CLL treatment. The algorithm provided a confidence estimate (low/high), thus providing four predictive groups: (1) High risk, high confidence, (2) high risk, low confidence, (3) low risk, low confidence and (4) low risk, high confidence. Outcome in terms of infections (all grades, ≥grade 3, +/- COVID-19), CLL treatment or death was registered. Results for patients not included for the randomized part due to patient’s preference or being assessed as one of the non-high risk, high confidence groups are reported. Patients were followed from time of CLL-TIM assessment if they had at least one follow-up visit prior to 16JAN2023 until the event of interest, last follow-up visit or death. Kaplan Meier plots and Cox proportional hazards models are reported. Results: For the 118 patients with data available, 12 (10%), 8 (7%), 62 (53%) and 36 (31 %) were assessed as high risk, high confidence, high risk, low confidence, low risk, low confidence and low risk, high confidence, respectively. High risk was more prevalent among male patients, IGHV unmutated patients, patients with del(17p) and patients with Binet stage B/C. The high-risk group had a lower hemoglobin level, lower platelet count and higher leukocyte count as compared to the low-risk patients. For the combined outcome of infections (any grade), CLL treatment or death within one year, the hazard ratio was 2.3 (95% CI 0.83-6.57) for the high risk, high confidence group as compared to the low risk, high confidence group (see figure). 29 out of 56 infections were categorized as being COVID related. Restricting the analysis to ≥ grade 3 infections (as defined for the trial) and/or to non-COVID infections, the hazard ratio for the high risk, high confidence group increased to 8.62 (95% CI 1.57-47.3) and 25.0 (95% CI 3.00-208) as compared to the low risk, high confidence group, respectively. Summary/Conclusion: Here the predictive performance of CLL-TIM is prospectively, clinically validated in the setting of the PreVent-ACaLL trial. To our knowledge, this is the first prospective clinical validation of a machine-learning based predictive model in hematology. CLL-TIM performed well during COVID despite being trained prior to the pandemic. Improved performance was demonstrated when restricting the outcome to ≥grade 3, non-COVID-19 infections. Thus, robustness of CLL-TIM in different clinical settings was demonstrated. CLL-TIM has been implemented into the electronic health record system of Eastern Denmark. The results of the ongoing PreVent-ACaLL trial, testing whether short-term acalabrutinib + venetoclax can improve the infection-free, treatment-free survival for these high-risk patients, are awaited.Keywords: Machine learning, Chronic lymphocytic leukemia, B cell chronic lymphocytic leukemia, B-CLL
更多查看译文
关键词
cll treatment infection model,clinical,prevent-acall
AI 理解论文
溯源树
样例
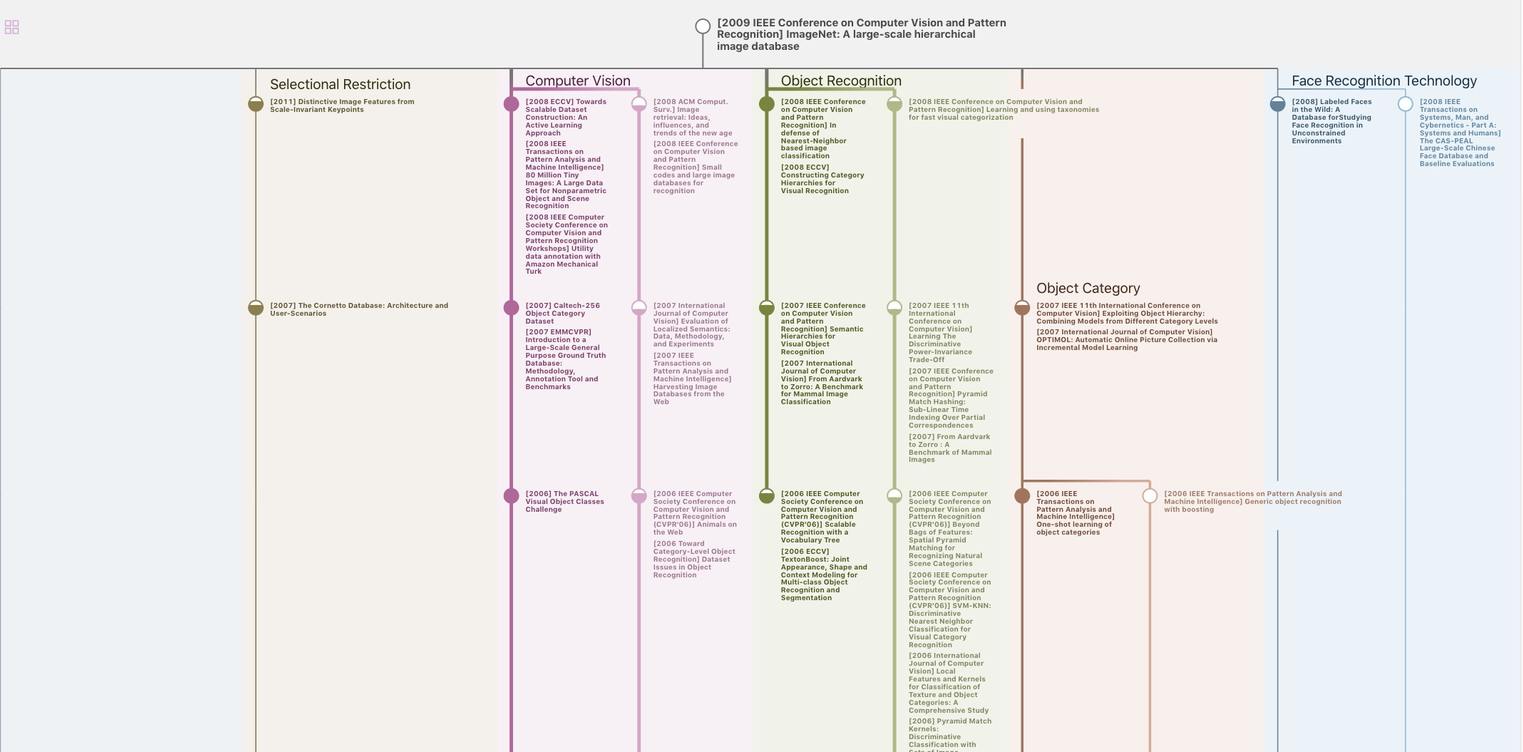
生成溯源树,研究论文发展脉络
Chat Paper
正在生成论文摘要