Stratified principal component analysis
arXiv (Cornell University)(2023)
摘要
This paper investigates a general family of covariance models with repeated eigenvalues extending probabilistic principal component analysis (PPCA). A geometric interpretation shows that these models are parameterised by flag manifolds and stratify the space of covariance matrices according to the sequence of eigenvalue multiplicities. The subsequent analysis sheds light on PPCA and answers an important question on the practical identifiability of individual eigenvectors. It notably shows that one rarely has enough samples to fit a covariance model with distinct eigenvalues and that block-averaging the adjacent sample eigenvalues with small gaps achieves a better complexity/goodness-of-fit tradeoff.
更多查看译文
关键词
stratified principal component analysis,principal component analysis
AI 理解论文
溯源树
样例
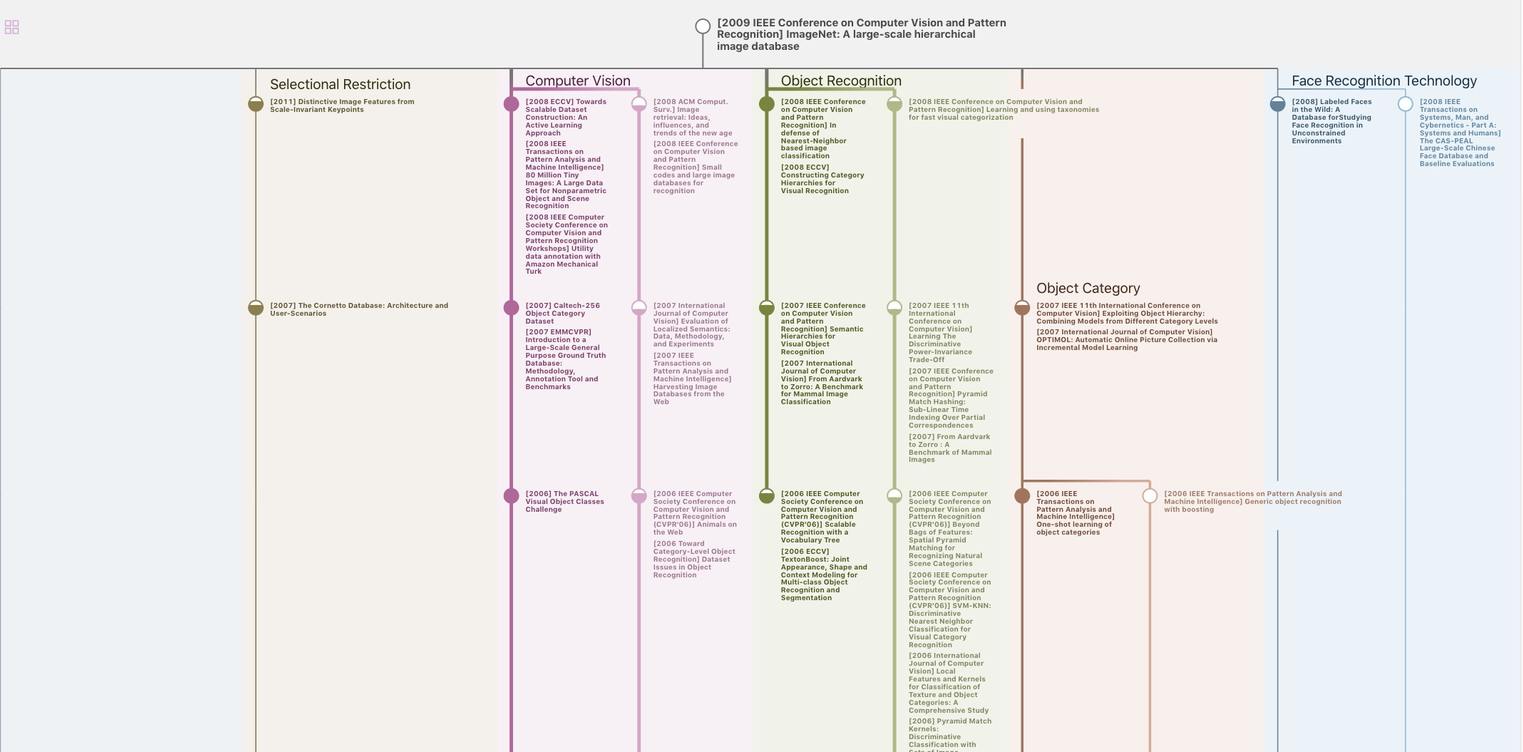
生成溯源树,研究论文发展脉络
Chat Paper
正在生成论文摘要