Deep Q-learning with hybrid quantum neural network on solving maze problems
Quantum Machine Intelligence(2024)
摘要
Quantum computing holds great potential for advancing the limitations of machine learning algorithms to handle higher dimensions of data and reduce overall training parameters in deep learning (DL) models. This study uses a trainable variational quantum circuit (VQC) on a gate-based quantum computing model to investigate the potential for quantum benefit in a model-free reinforcement learning problem. Through a comprehensive investigation and evaluation of the current model and capabilities of quantum computers, we designed and trained a novel hybrid quantum neural network based on the latest Qiskit and PyTorch framework. We compared its performance with a full-classical CNN with and without an incorporated VQC. Our research provides insights into the potential of deep quantum learning to solve a maze problem and, potentially, other reinforcement learning problems. We conclude that reinforcement learning problems can be practical with reasonable training epochs. Moreover, a comparative study of full-classical and hybrid quantum neural networks is discussed to understand these two approaches’ performance, advantages, and disadvantages to deep Q-learning problems, especially on larger-scale maze problems larger than 4 × 4.
更多查看译文
关键词
Quantum machine learning,Hybrid quantum neural networks,Deep reinforcement learning,Quantum deep Q-learning
AI 理解论文
溯源树
样例
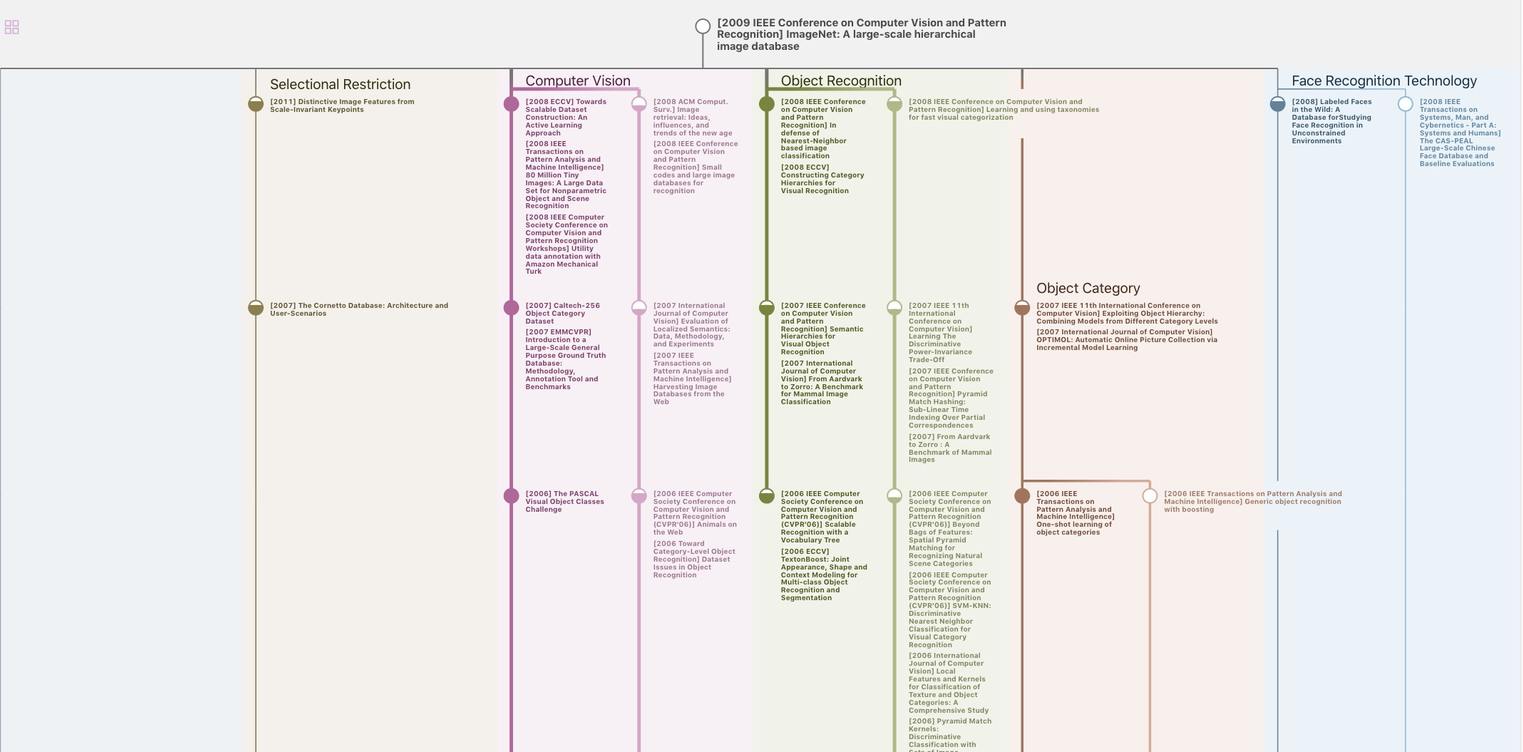
生成溯源树,研究论文发展脉络
Chat Paper
正在生成论文摘要