The Deep Method: Towards Computational Modeling of the Social Emotion Shame driven by Theory, Introspection, and Social Signals
IEEE Transactions on Affective Computing(2023)
摘要
Understanding emotions is key to Affective Computing. Emotion recognition focuses on the communicative component of emotions encoded in social signals. This view alone is insufficient for a deeper understanding and computational representation of the internal, subjectively experienced component of emotions. This paper presents a cognition-based method called
Deep
as a starting point for deeper computational modeling of the internal component of emotions.
Deep
incorporates an approach to query individual internal emotional experiences and to represent such information computationally. It combines social signals, verbalized introspection information, context information, and theory-driven knowledge. We apply the
Deep
method to the emotion of shame as an example and compare it to a typical emotion recognition model, highlighting the differences and advantages.
更多查看译文
关键词
Emotion Modeling,Methods,Empirical Study,Bayesian Network
AI 理解论文
溯源树
样例
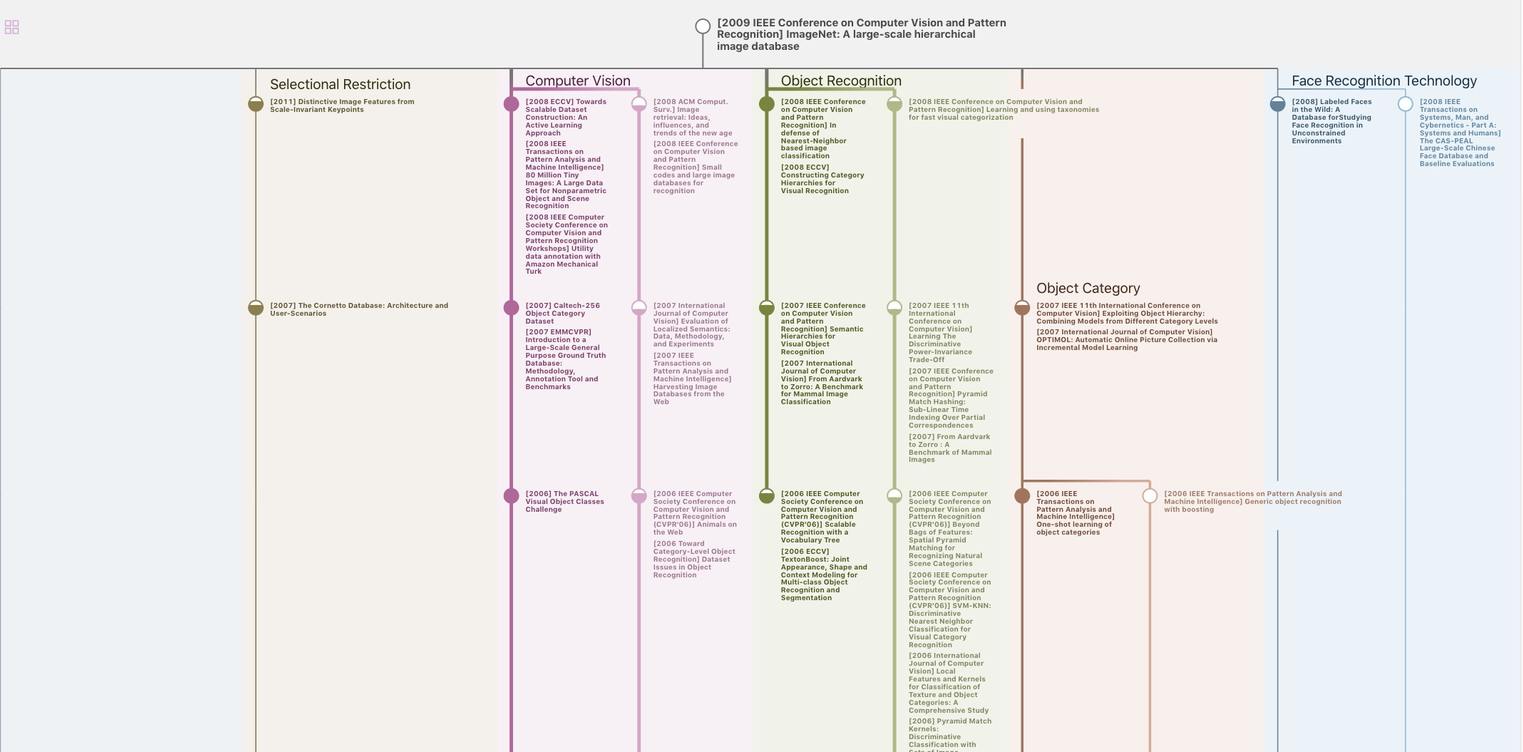
生成溯源树,研究论文发展脉络
Chat Paper
正在生成论文摘要