A Reduced-Memory Multi-layer Perceptron with Systematic Network Weights Generated and Trained Through Distribution Hyper-parameters
Lecture notes in networks and systems(2023)
摘要
In this paper, we propose an alternate way to represent and train the weights of a Multi-Layer Perceptron. The individual weights of all connections from a node are represented by a statistical distribution for which the hyper-parameters are trained. Although the proposed distribution-based neural network was difficult to train using standard backpropagation and gradient descent algorithms, we successfully used a genetic algorithm-based training strategy on experimental datasets. On a number of binary classification datasets in the public domain, contemporary state-of-the-art algorithms were used for comparative benchmarking of the model efficiency. Distribution-based neural network was ranked two out of the six training methods in final accuracy (behind backpropagation) but achieved substantial memory and time complexity gains of 20 and more than 100 folds, respectively. We believe that the model offers a promising alternative training method for large networks with small performance trade-offs.
更多查看译文
关键词
systematic network weights generated,reduced-memory,multi-layer,hyper-parameters
AI 理解论文
溯源树
样例
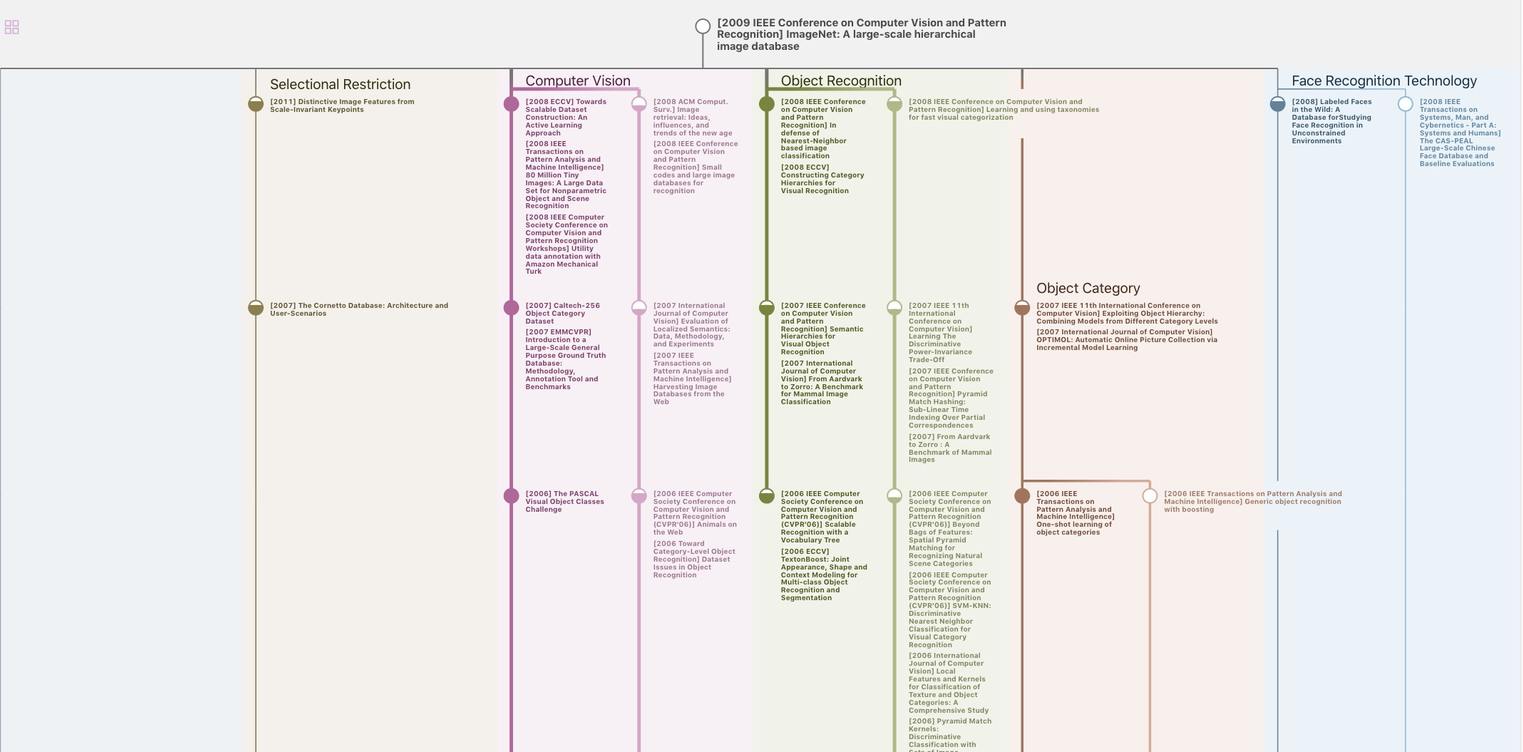
生成溯源树,研究论文发展脉络
Chat Paper
正在生成论文摘要