STUDY: Socially Aware Temporally Causal Decoder Recommender Systems
arXiv (Cornell University)(2023)
摘要
Recommender systems are widely used to help people find items that are tailored to their interests. These interests are often influenced by social networks, making it important to use social network information effectively in recommender systems. This is especially true for demographic groups with interests that differ from the majority. This paper introduces STUDY, a Socially-aware Temporally caUsal Decoder recommender sYstem. STUDY introduces a new socially-aware recommender system architecture that is significantly more efficient to learn and train than existing methods. STUDY performs joint inference over socially connected groups in a single forward pass of a modified transformer decoder network. We demonstrate the benefits of STUDY in the recommendation of books for students who are dyslexic, or struggling readers. Dyslexic students often have difficulty engaging with reading material, making it critical to recommend books that are tailored to their interests. We worked with our non-profit partner Learning Ally to evaluate STUDY on a dataset of struggling readers. STUDY was able to generate recommendations that more accurately predicted student engagement, when compared with existing methods.
更多查看译文
AI 理解论文
溯源树
样例
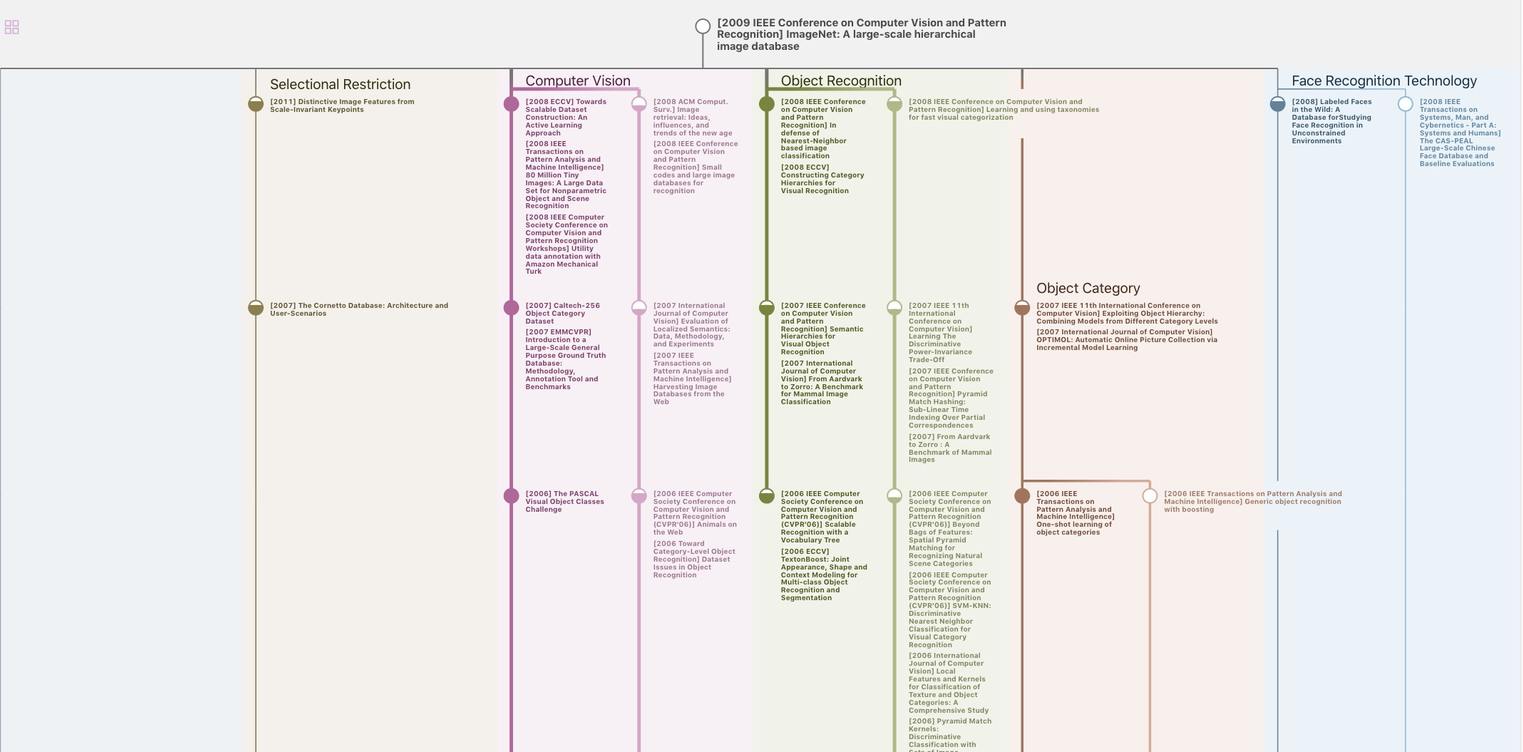
生成溯源树,研究论文发展脉络
Chat Paper
正在生成论文摘要