Abstract 5445: Segmentation-free analysis of multiplexed images with unbiased spatial analytics and explainable AI for predicting disease outcomes
Cancer Research(2023)
摘要
Abstract Background: The spatial intratumor heterogeneity (ITH) is widely acknowledged as driving therapeutic response and providing fuel for drug resistance. Currently, patient selection for immunotherapy is driven mostly by PD-1/PD-L1 based IHC tests and mutational analysis. These oversimplified approaches fail to predict the risk of recurrence, therapeutic response and drug resistance with high accuracy. We hypothesize that functional responses of heterogeneous non-random spatial arrangements of tumor, stromal and immune cells in the tumor microenvironment are determined by distinct combinations of their internal states and spatial interactions within neighborhoods. The interactions generate distinct cell-cell communication networks forming functional spatial configurations of cells, termed microdomains, as organizational units of spatial ITH. Deriving the spatial networks within each microdomain with unbiased spatial analytics and the underlying network biology through explainable AI, is key to understanding tumor initiation, tumor progression, and response to therapy. Problem: There has been an explosion of spatial imaging technologies using immunofluorescence and/or mass spectrometry for intact tissues measuring protein expressions, DNA and RNA probes. To extract high-value knowledge from these multiplexed datasets, a key first step is to segment cells accurately. Despite decades of research, this step remains elusive due to imaging artifacts arising from issues with tile stitching, incorrect registration, signal oversaturation, non-uniform illumination, poor or high-background nuclei signal, defocus, varying cell and nuclear shapes, fluorescence emission efficiency variation, overlapping and/or superimposed nuclei and low resolution (e.g., mass cytometry). Those artifacts may lead to incorrect cell phenotypes, incomplete cell phenotype atlases, and missing rare cell, fusion cell or transition cell types. Solution: We present TumorMapr™, a segmentation-free, unbiased spatial analytics and explainable AI platform that extracts information and creates knowledge from patient disease pathology samples imaged with any technology including fluorescence, mass spectrometry etc. Results: We apply TumorMapr on hyperplexed immunofluorescence dataset of colorectal cancer and hyperplexed imaging mass cytometry dataset of triple negative breast cancer to discover functional cell prototypes involving spatial collections of neighboring pixels highly predictive of disease progression and response to therapy, tumor promoting and tumor restraining microdomains and microdomain-specific network biology predictive of disease outcomes. We further compare these results with segmentation-based approaches to showcase the discovery of novel cell prototypes. Citation Format: Filippo Pullara, Brian Falkenstein, Bruce Campbell, Samantha Panakkal, Akif Burak Tosun, Jeffrey Fine, S. Chakra Chennubhotla. Segmentation-free analysis of multiplexed images with unbiased spatial analytics and explainable AI for predicting disease outcomes. [abstract]. In: Proceedings of the American Association for Cancer Research Annual Meeting 2023; Part 1 (Regular and Invited Abstracts); 2023 Apr 14-19; Orlando, FL. Philadelphia (PA): AACR; Cancer Res 2023;83(7_Suppl):Abstract nr 5445.
更多查看译文
关键词
unbiased spatial analytics,multiplexed images,explainable explainable,segmentation-free
AI 理解论文
溯源树
样例
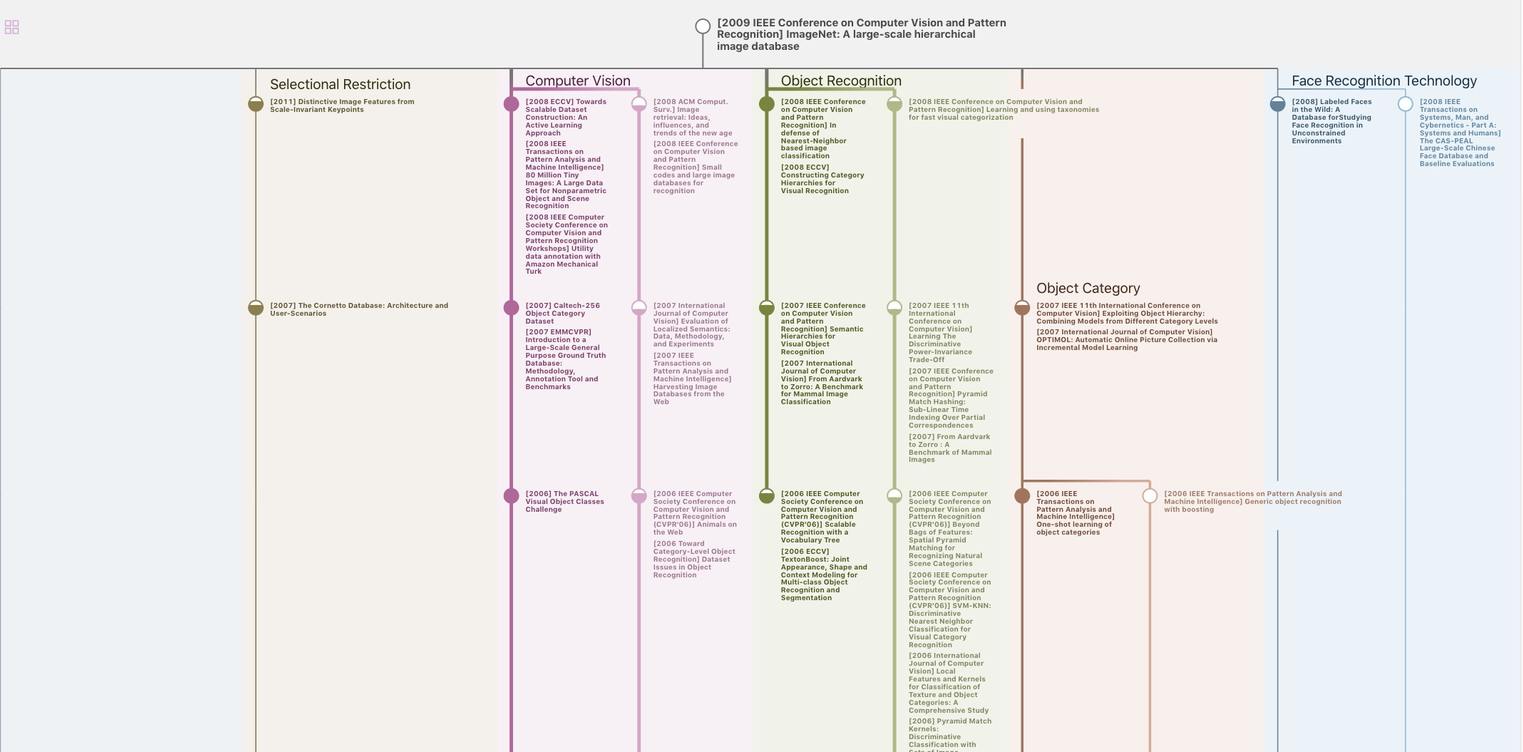
生成溯源树,研究论文发展脉络
Chat Paper
正在生成论文摘要