Multi-type Features Graph Neural Networks for Cocrystals Prediction using API-Coformers Interactions
Research Square (Research Square)(2023)
摘要
Abstract Active pharmaceutical ingredients (APIs) have gained direct pharmaceutical interest, along with their in vitro properties, and thus utilized as auxiliary solid dosage forms upon FDA guidance and approval on pharmaceutical cocrystals as a potential and attractive route for drug substance development. In this study, we implemented graph neural networks to predict the formationof cocrystals using our first created API-co-formers interactions graph dataset. We further compared our work with previous studies that implemented descriptor-based models (e.g., random forest, support vector machine, extreme gradient boosting,and artificial neural networks). All built graph-based models show compelling performance accuracies (i.e., 91.36, 94.60, and 95.95% for GCN, GraphSAGE, and R-GCN respectively). Furthermore, R-GCN prevailed among the built graph-based models due to its capability to learn the topological structure of the graph from the additionally provided information (i.e., non-ionic andnon-covalent interactions or link information) between APIs and coformers.
更多查看译文
关键词
cocrystals prediction,neural networks,multi-type,api-coformers
AI 理解论文
溯源树
样例
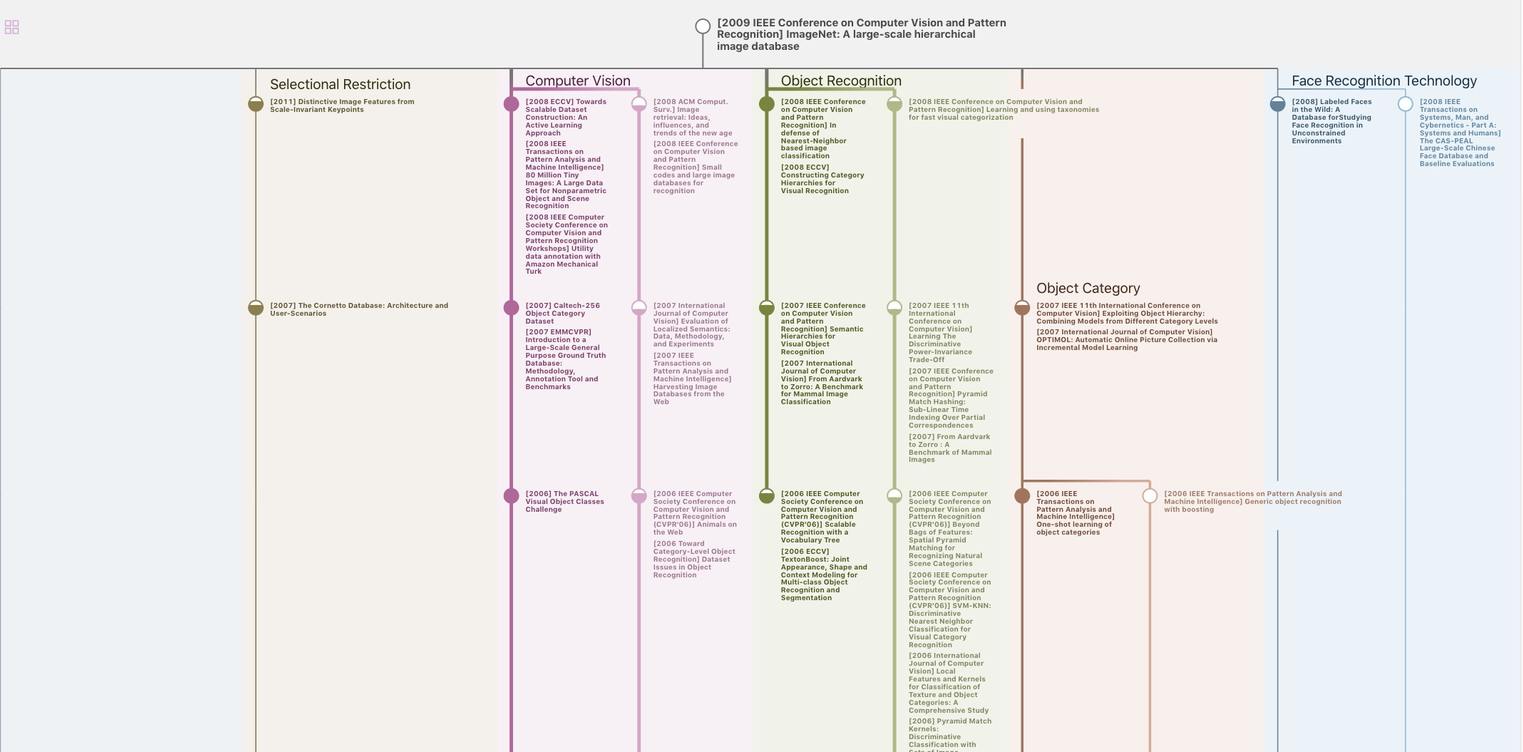
生成溯源树,研究论文发展脉络
Chat Paper
正在生成论文摘要