NashFormer: Leveraging Local Nash Equilibria for Semantically Diverse Trajectory Prediction
arXiv (Cornell University)(2023)
摘要
Interactions between road agents present a significant challenge in trajectory prediction, especially in cases involving multiple agents. Because existing diversity-aware predictors do not account for the interactive nature of multi-agent predictions, they may miss these important interaction outcomes. In this paper, we propose NashFormer, a framework for trajectory prediction that leverages game-theoretic inverse reinforcement learning to improve coverage of multi-modal predictions. We use a training-time game-theoretic analysis as an auxiliary loss resulting in improved coverage and accuracy without presuming a taxonomy of actions for the agents. We demonstrate our approach on the interactive split of the Waymo Open Motion Dataset, including four subsets involving scenarios with high interaction complexity. Experiment results show that our predictor produces accurate predictions while covering $33\%$ more potential interactions versus a baseline model.
更多查看译文
关键词
trajectory
AI 理解论文
溯源树
样例
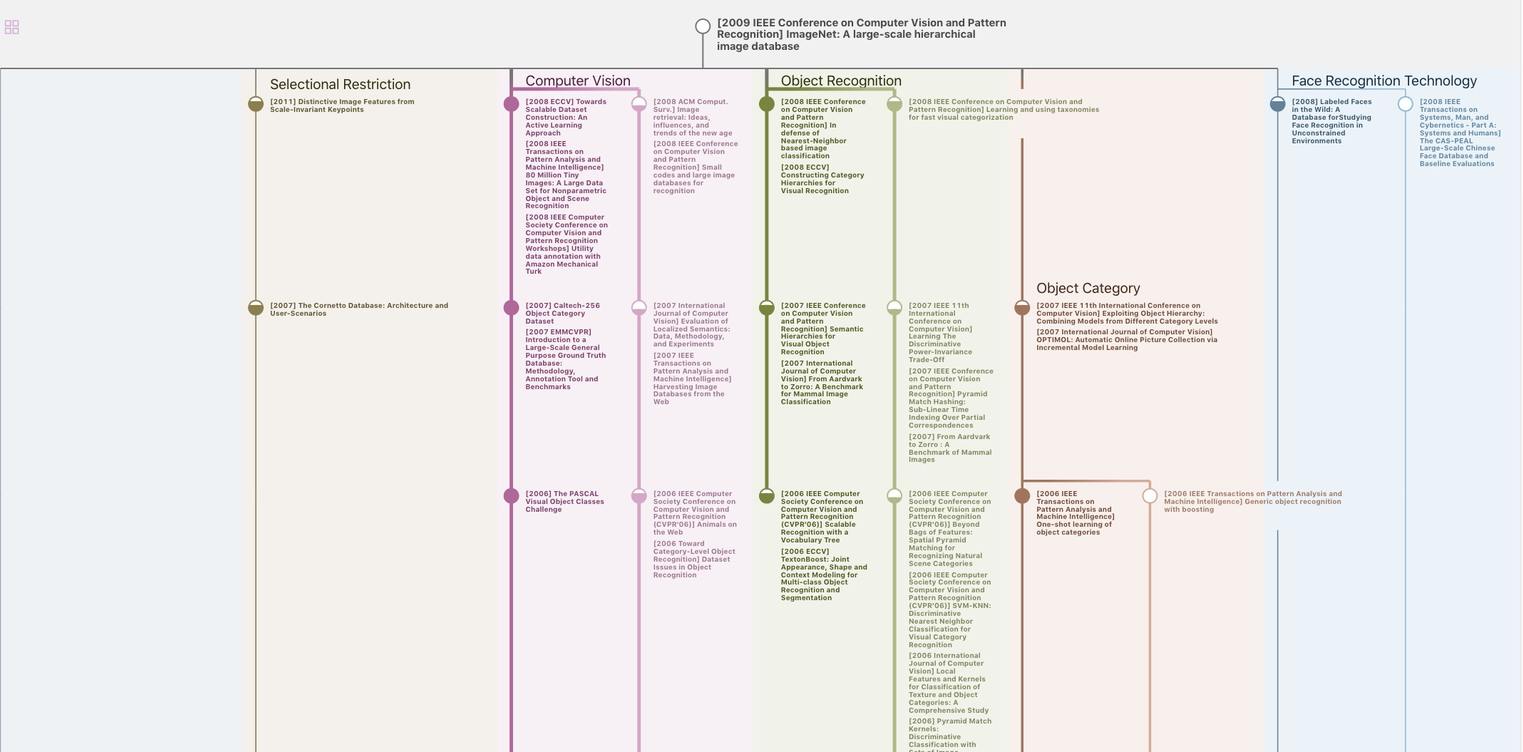
生成溯源树,研究论文发展脉络
Chat Paper
正在生成论文摘要