A Novel Autoencoder for Task-Driven Object Segmentation
Communications in computer and information science(2023)
摘要
Salient object segmentation has attracted increasing attention in recent years. Though a lot of works based on autoencoder have been made, these methods can only recognize and segment one class of objects, but cannot segment the other classes of objects in the same image. To address this issue, this paper proposes a novel autoencoder to perform task-driven object segmentation, in which a control signal is added to the decoder to determine which class of objects need to be segmented. What’s more, we establish a Bayesian rule in the decoder, in which the control signal is set as the prior, and the latent features learned in encoder is transferred to corresponding layer of decoder as observation, thus the posterior probability of each object with respect to the specific-class can be calculated, and the objects belonging to this class can be segmented. This proposed method is evaluated for task-driven salient object segmentation on a several benchmark datasets, including DUT-OMRON, ECSSD, DUTS-TE, etc. Experimental results show that our approach tends to segment accurate, detailed and complete objects, and improves the performance compared with the previous state-of-the-arts.
更多查看译文
关键词
novel autoencoder,segmentation,object,task-driven
AI 理解论文
溯源树
样例
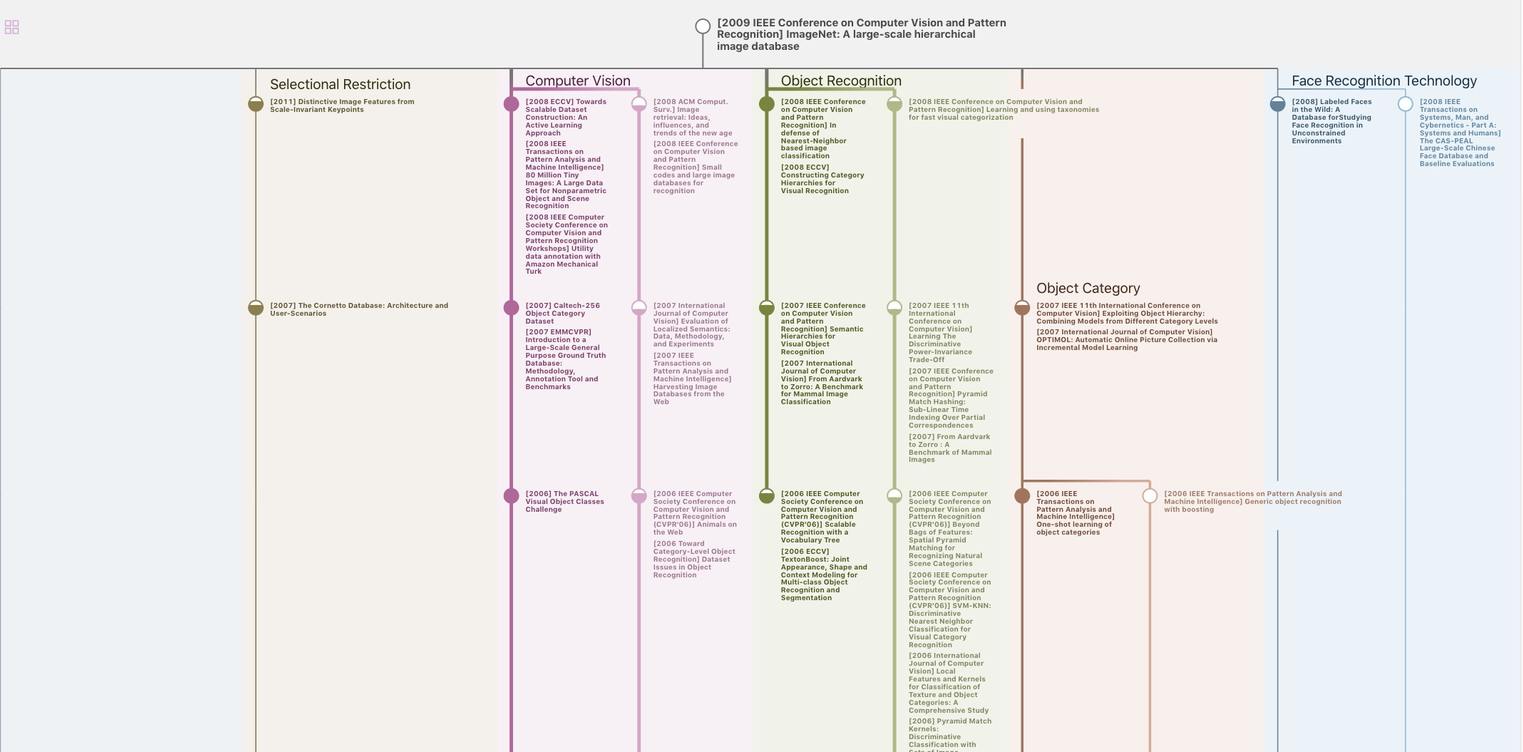
生成溯源树,研究论文发展脉络
Chat Paper
正在生成论文摘要