Driver intention prediction based on multi-dimensional cross-modality information interaction
Multimedia Systems(2024)
摘要
Driver intention prediction allows drivers to perceive possible dangers in the fastest time and has become one of the most important research topics in the field of self-driving in recent years. In this study, we propose a driver intention prediction method based on multi-dimensional cross-modality information interaction. First, an efficient video recognition network is designed to extract channel-temporal features of in-side (driver) and out-side (road) videos, respectively, in which we design a cross-modality channel-spatial weight mechanism to achieve information interaction between the two feature extraction networks corresponding, respectively, to the two modalities, and we also introduce a contrastive learning module by which we force the two feature extraction networks to enhance structural knowledge interaction. Then, the obtained representations of in- and outside videos are fused using a ResLayer-based module to get a preliminary prediction which is then corrected by incorporating the GPS information to obtain a final decision. Besides, we employ a multi-task framework to train the entire network. We validate the proposed method on the public dataset Brain4Car, and the results show that the proposed method achieves competitive results in accuracy while balancing performance and computation.
更多查看译文
关键词
Driver intention prediction,Self-driving,Multimodal learning,Contrastive learning,Deep neural networks
AI 理解论文
溯源树
样例
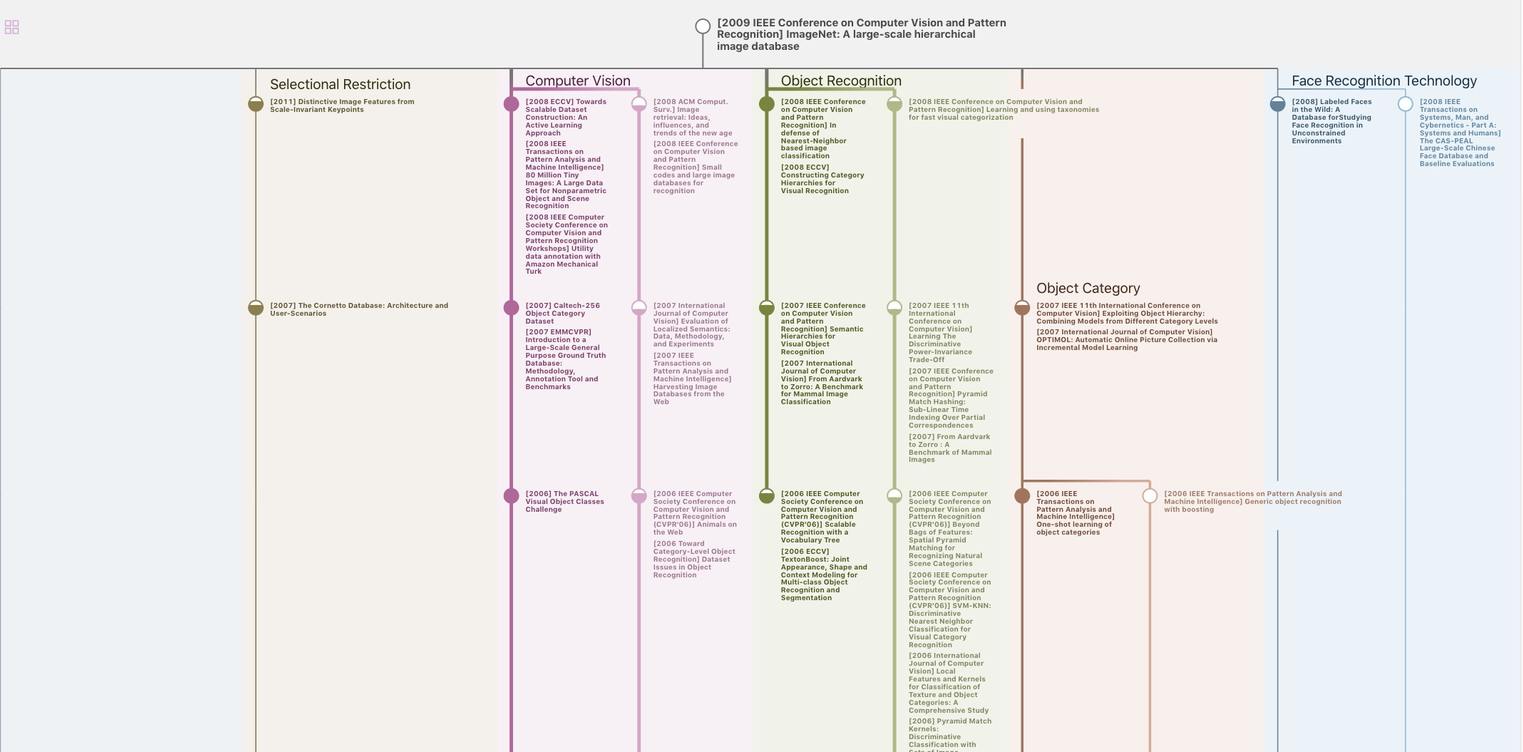
生成溯源树,研究论文发展脉络
Chat Paper
正在生成论文摘要