DSPDet3D: Dynamic Spatial Pruning for 3D Small Object Detection
arXiv (Cornell University)(2023)
摘要
Fine-grained 3D object detection is a core ability for agents to understand their 3D environment and interact with surrounding objects. However, current methods and benchmarks mainly focus on relatively large stuff. 3D object detectors still struggle on small objects due to weak geometric information. With in-depth study, we find increasing the spatial resolution of the feature maps significantly boosts the performance of 3D small object detection. And more interestingly, though the computational overhead increases dramatically with resolution, the growth mainly comes from the upsampling operation of the decoder. Inspired by this, we present a high-resolution multi-level detector with dynamic spatial pruning named DSPDet3D, which detects objects from large to small by iterative upsampling and meanwhile prunes the spatial representation of the scene at regions where there is no smaller object to be detected in higher resolution. We organize two benchmarks on ScanNet and TO-SCENE dataset to evaluate the ability of fine-grained 3D object detection, where our DSPDet3D improves the detection performance of small objects to a new level while achieving leading inference speed compared with existing 3D object detection methods. Moreover, DSPDet3D trained with only ScanNet rooms can generalize well to scenes in larger scale. It takes less than 2s for DSPDet3D to directly process a whole house or building consisting of dozens of rooms while detecting out almost all objects, ranging from bottles to beds, on a single RTX 3090 GPU. Project page: https://xuxw98.github.io/DSPDet3D/.
更多查看译文
关键词
dynamic spatial pruning,detection,object
AI 理解论文
溯源树
样例
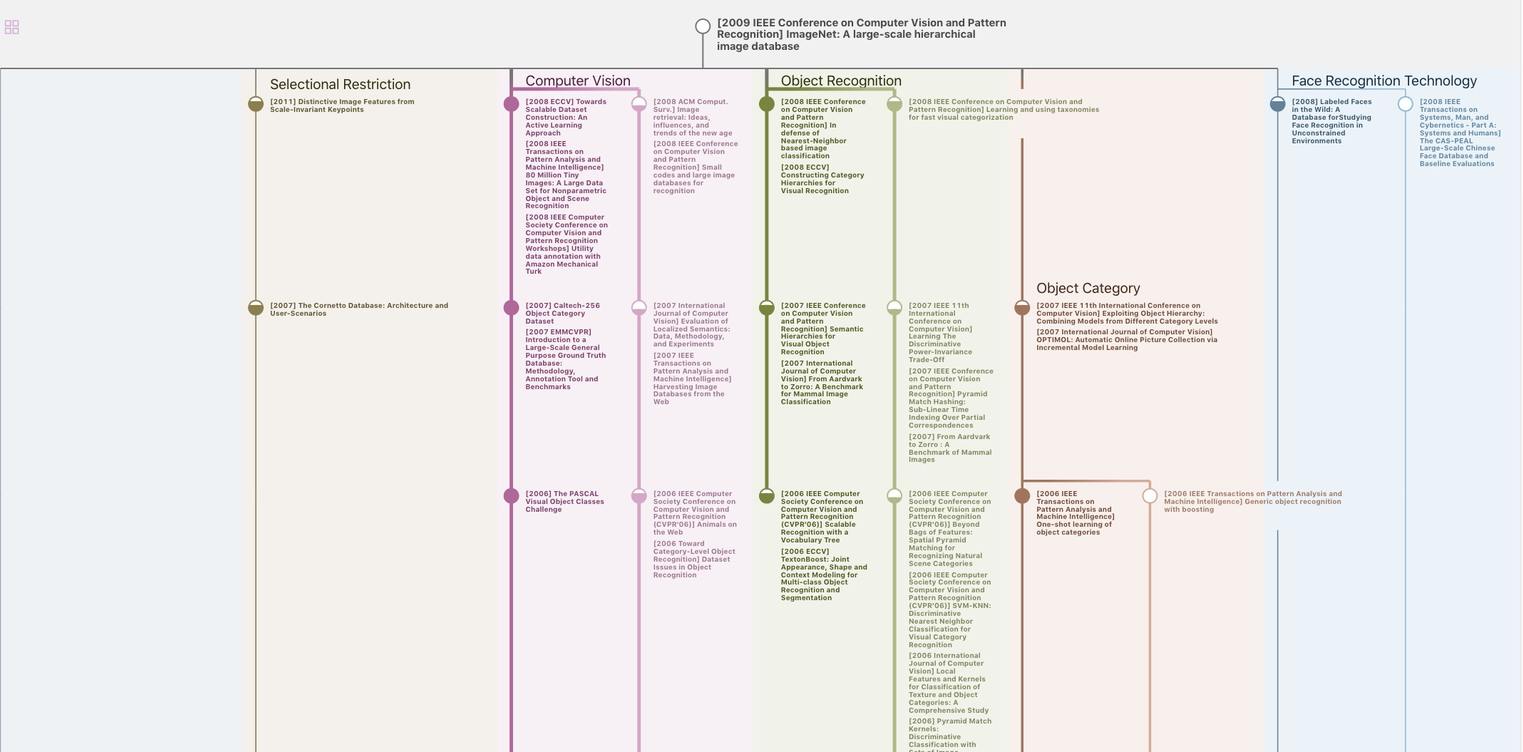
生成溯源树,研究论文发展脉络
Chat Paper
正在生成论文摘要