Exploration of Bitflip’s Effect on DNN Accuracy in Plaintext and Ciphertext
IEEE Micro(2023)
摘要
Neural Networks (NNs) are increasingly deployed to solve complex classification problems and produce accurate results on reliable systems. However, its accuracy quickly degrades in the presence of bit flips from memory errors or targeted attacks on DRAM main memory. Prior work has shown that a few bit errors significantly reduce NN accuracies, but it is unclear which bits have an outsized impact on network accuracy and why. This paper first investigates the relationship of the number representation for NN parameters with the impacts of bit flips on NN accuracy. We then explore the Bit Flip Detection (BFD) framework— four software-based error detectors that detect bit flips independent of NN topology. We discuss exciting findings and evaluate the various detectors’ efficacy, characteristics, and trade-offs.
更多查看译文
关键词
dnn accuracy,bitflips,plaintext
AI 理解论文
溯源树
样例
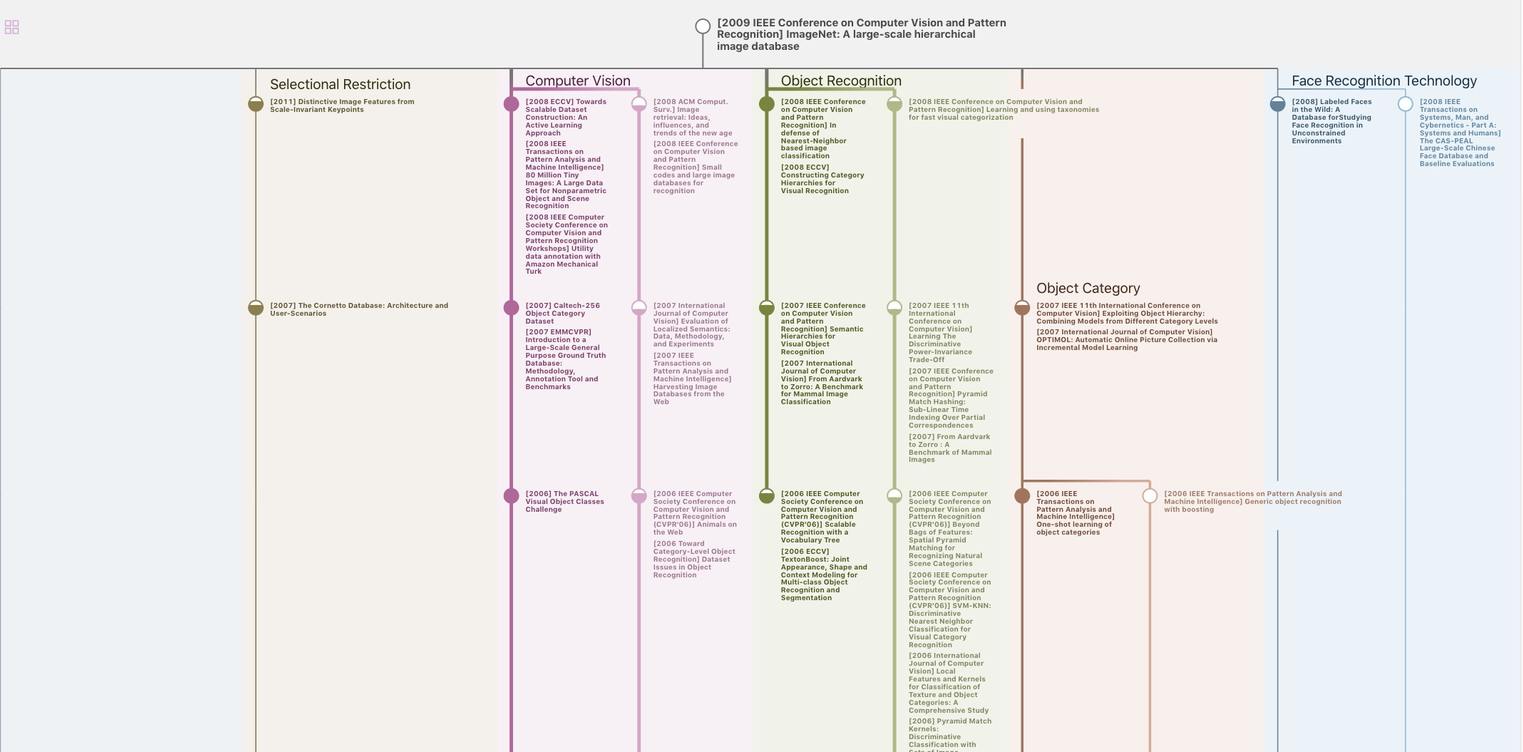
生成溯源树,研究论文发展脉络
Chat Paper
正在生成论文摘要