Mobile Collaborative Learning Over Opportunistic Internet of Vehicles.
IEEE Trans. Mob. Comput.(2024)
摘要
Machine learning models are widely applied for vehicular applications, which are essential to future intelligent transportation system (ITS). Traditional model training methods commonly employ a client-server architecture to perform local training and global iterative aggregations, which can consume significant bandwidth resources that are often absent in vehicular networks, especially in high vehicle density scenarios. Modern vehicle users naturally can collaboratively train machine learning models as they are the data owner and have strong local computing power from the onboard units (OBU). In this paper, we propose a novel collaborative learning scheme for mobile vehicles that can utilize the opportunistic vehicle-to-roadside (V2R) communication to exploit the common priors of vehicular data without interaction with a centralized coordinator. Specifically, vehicles perform local training during the driving journey, and simply upload its local model to roadside unit (RSU) encountered on the way. RSU's model will be updated accordingly and sent back to the vehicle via the V2R communication. We have theoretically shown that RSUs' models can eventually converge without a backhaul connection. Extensive experiments upon various road configurations demonstrate that the proposed scheme can efficiently train models among vehicles without dedicated Internet access and scale well with both the road range and vehicle density.
更多查看译文
关键词
Collaborative learning,Internet of vehicles,distributed learning,opportunistic communication
AI 理解论文
溯源树
样例
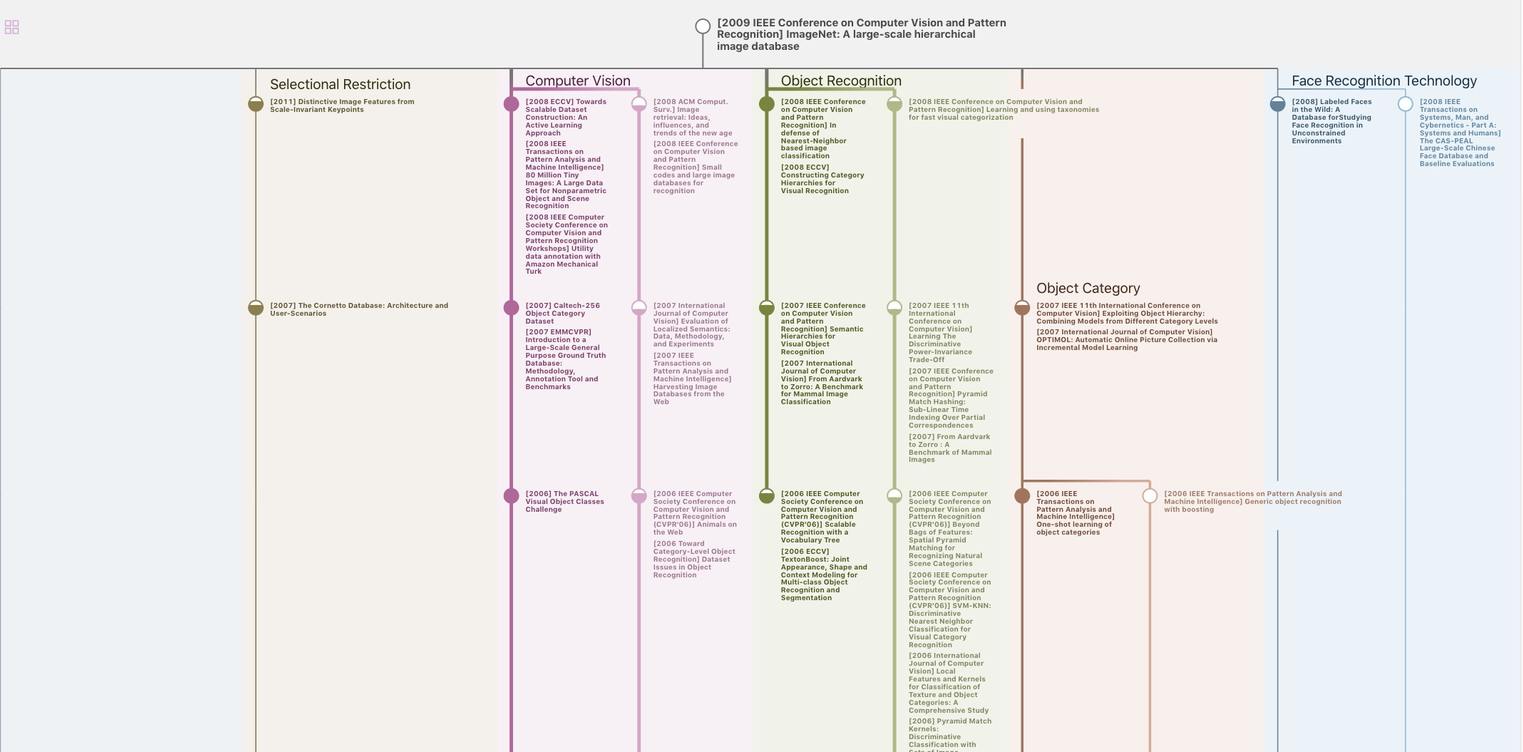
生成溯源树,研究论文发展脉络
Chat Paper
正在生成论文摘要