An Evaluation of Memory Optimization Methods for Training Neural Networks
arXiv (Cornell University)(2023)
摘要
As models continue to grow in size, the development of memory optimization methods (MOMs) has emerged as a solution to address the memory bottleneck encountered when training large models. To comprehensively examine the practical value of various MOMs, we have conducted a thorough analysis of existing literature from a systems perspective. Our analysis has revealed a notable challenge within the research community: the absence of standardized metrics for effectively evaluating the efficacy of MOMs. The scarcity of informative evaluation metrics hinders the ability of researchers and practitioners to compare and benchmark different approaches reliably. Consequently, drawing definitive conclusions and making informed decisions regarding the selection and application of MOMs becomes a challenging endeavor. To address the challenge, this paper summarizes the scenarios in which MOMs prove advantageous for model training. We propose the use of distinct evaluation metrics under different scenarios. By employing these metrics, we evaluate the prevailing MOMs and find that their benefits are not universal. We present insights derived from experiments and discuss the circumstances in which they can be advantageous.
更多查看译文
关键词
memory optimization methods,optimization methods,training neural,neural
networks
AI 理解论文
溯源树
样例
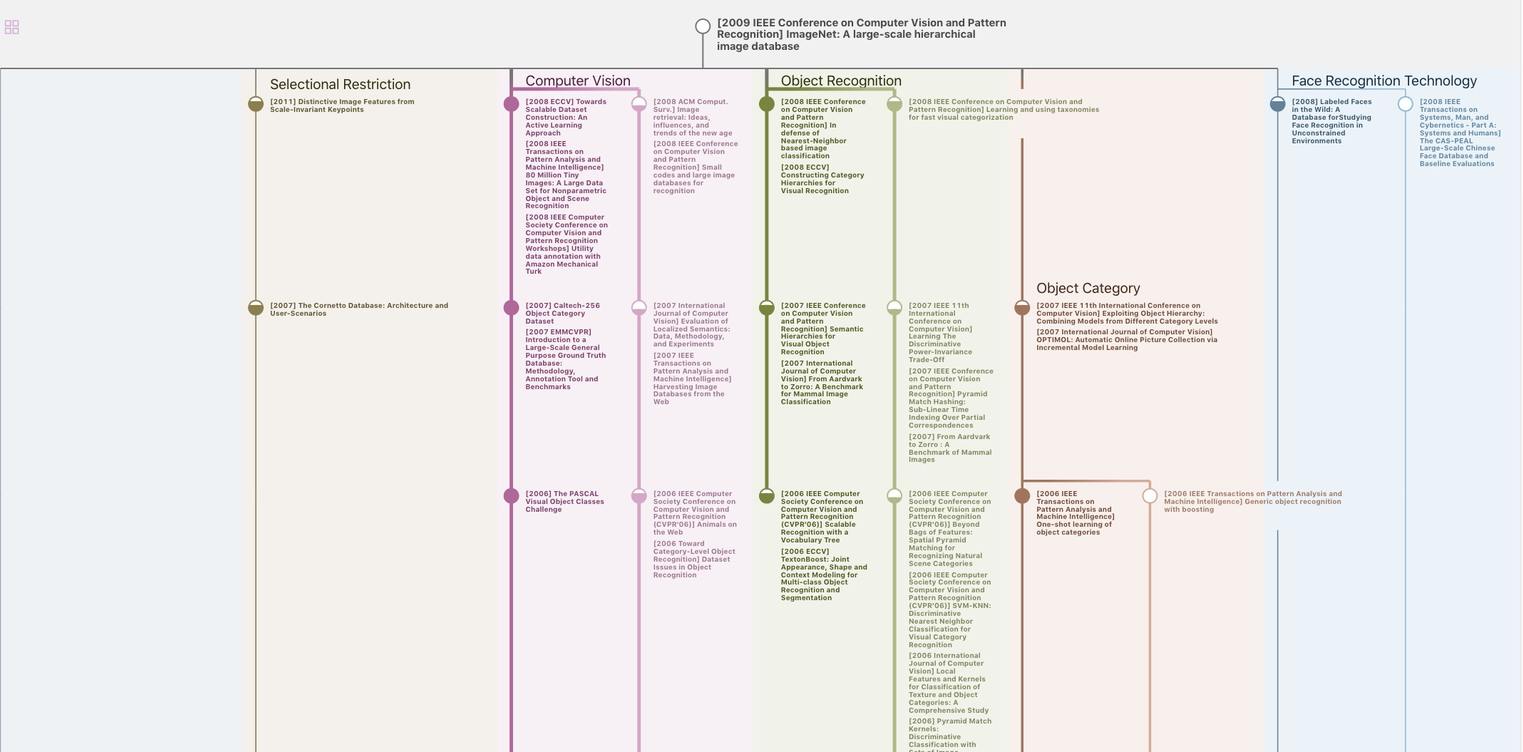
生成溯源树,研究论文发展脉络
Chat Paper
正在生成论文摘要