FAIR-ification of structured Head and Neck Cancer clinical data for multi-institutional collaboration and federated learning
Research Square (Research Square)(2023)
摘要
Abstract Federated learning has been demonstrated as an acceptable clinical research methodology for producing analyses and models on dispersed datasets, without the need for exchanging individual patient-level data. Attention needs to be given to making repositories of clinical data Findable, Accessible, Interoperable and Reusable (FAIR) in order to realize the potential of such clinical data in federated learning applications. This work draws attention to FAIR-ification structured clinical data of Head and Neck cancer patients, generated in different parts of the world with incompatible terminologies. We began with an “open world” approach by converting the native datasets into the Resource Descriptor Framework format, and then applying a customized local annotation for each dataset to map the data fields to open access ontologies. This approach allows interactive data exploration by means of a federated SPARQL query-based dashboard. The annotations and dashboard visualizations were constructed without using the individual patient-level data. It is feasible to develop and validate multi-institutional statistical models with federated learning on top of the annotations that make the data FAIR. Findings are robust and potentially scalable to a larger number of participating institutions. The annotation methodology proposed here supports multiple simultaneous mappings (such as the data being re-used in multiple different projects) while keeping the native data the same. Future work may be to include certain rules and requirements for classes and predicates, and using the Shapes Constraint Language for checking the validity of the data.
更多查看译文
关键词
neck cancer,clinical data,collaboration,structured head,fair-ification,multi-institutional
AI 理解论文
溯源树
样例
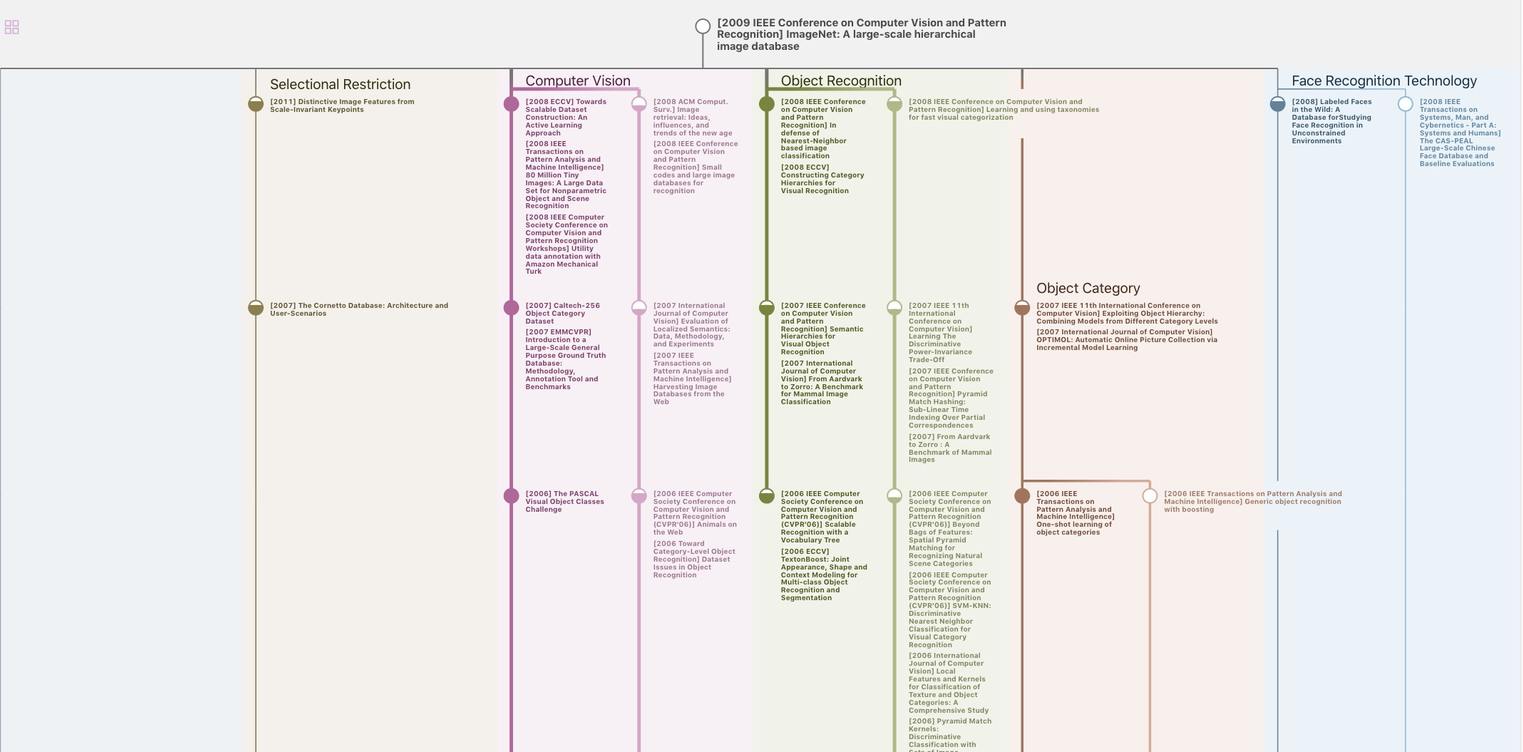
生成溯源树,研究论文发展脉络
Chat Paper
正在生成论文摘要