Local-global methods for generalised solar irradiance forecasting
Applied Intelligence(2024)
摘要
For efficient operation, solar power operators often require generation forecasts for multiple sites with varying data availability. Many proposed methods for forecasting solar irradiance / solar power production formulate the problem as a time-series, using current observations to generate forecasts. This necessitates a real-time data stream and enough historical observations at every location for these methods to be deployed. In this paper, we propose the use of Global methods to train generalised models. Using data from 20 locations distributed throughout the UK, we show that it is possible to learn models without access to data for all locations, enabling them to generate forecasts for unseen locations. We show a single Global model trained on multiple locations can produce more consistent and accurate results across locations. Furthermore, by leveraging weather observations and measurements from other locations we show it is possible to create models capable of accurately forecasting irradiance at locations without any real-time data. We apply our approaches to both classical and state-of-the-art Machine Learning methods, including a Transformer architecture. We compare models using satellite imagery or point observations (temperature, pressure, etc.) as weather data. These methods could facilitate planning and optimisation for both newly deployed solar farms and domestic installations from the moment they come online.
更多查看译文
关键词
Deep learning,Time series forecast,Solar irradiance forecast,Generalised model,Local-global
AI 理解论文
溯源树
样例
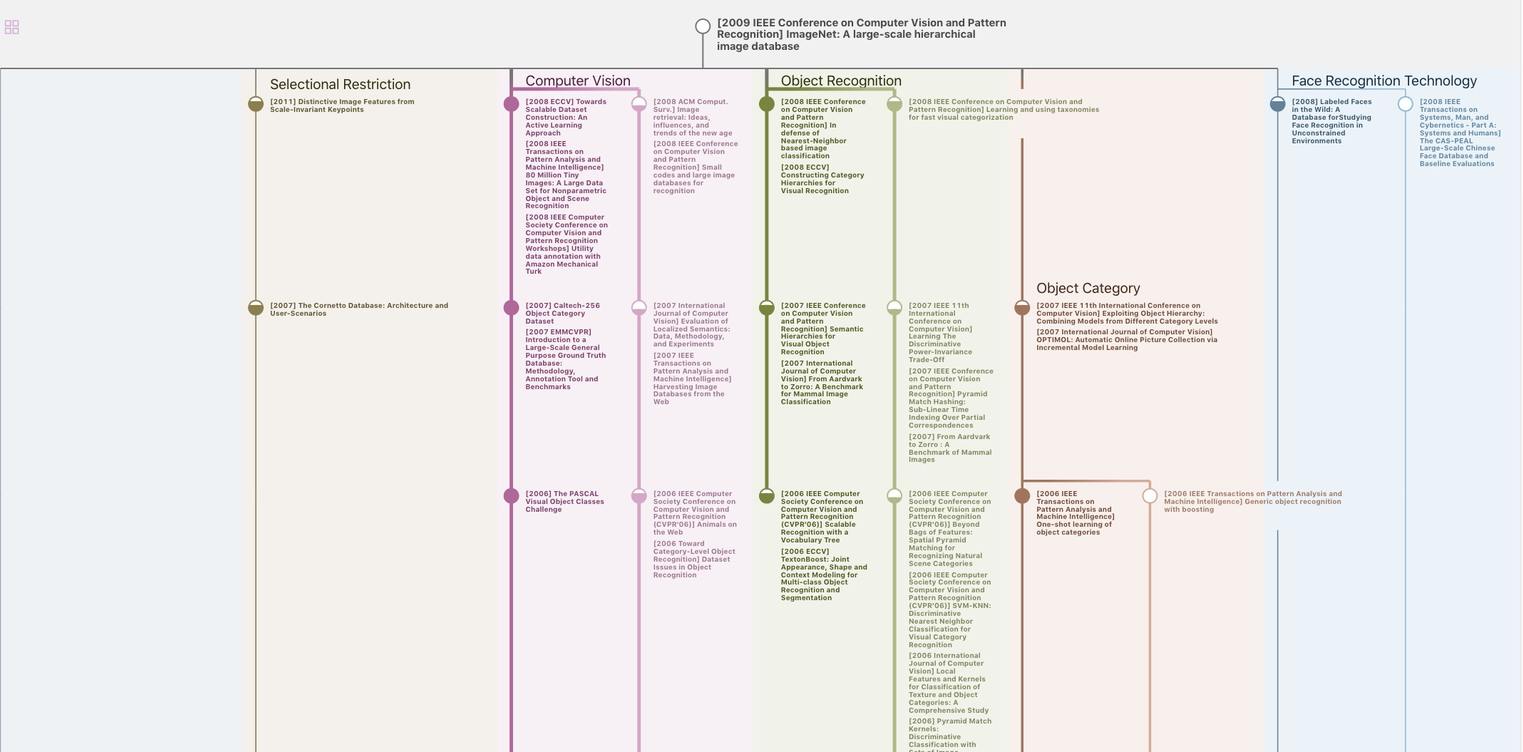
生成溯源树,研究论文发展脉络
Chat Paper
正在生成论文摘要