Leveraging Prior Knowledge in Reinforcement Learning via Double-Sided Bounds on the Value Function
arXiv (Cornell University)(2023)
摘要
An agent's ability to leverage past experience is critical for efficiently solving new tasks. Approximate solutions for new tasks can be obtained from previously derived value functions, as demonstrated by research on transfer learning, curriculum learning, and compositionality. However, prior work has primarily focused on using value functions to obtain zero-shot approximations for solutions to a new task. In this work, we show how an arbitrary approximation for the value function can be used to derive double-sided bounds on the optimal value function of interest. We further extend the framework with error analysis for continuous state and action spaces. The derived results lead to new approaches for clipping during training which we validate numerically in simple domains.
更多查看译文
关键词
reinforcement learning,prior knowledge,value function,double-sided
AI 理解论文
溯源树
样例
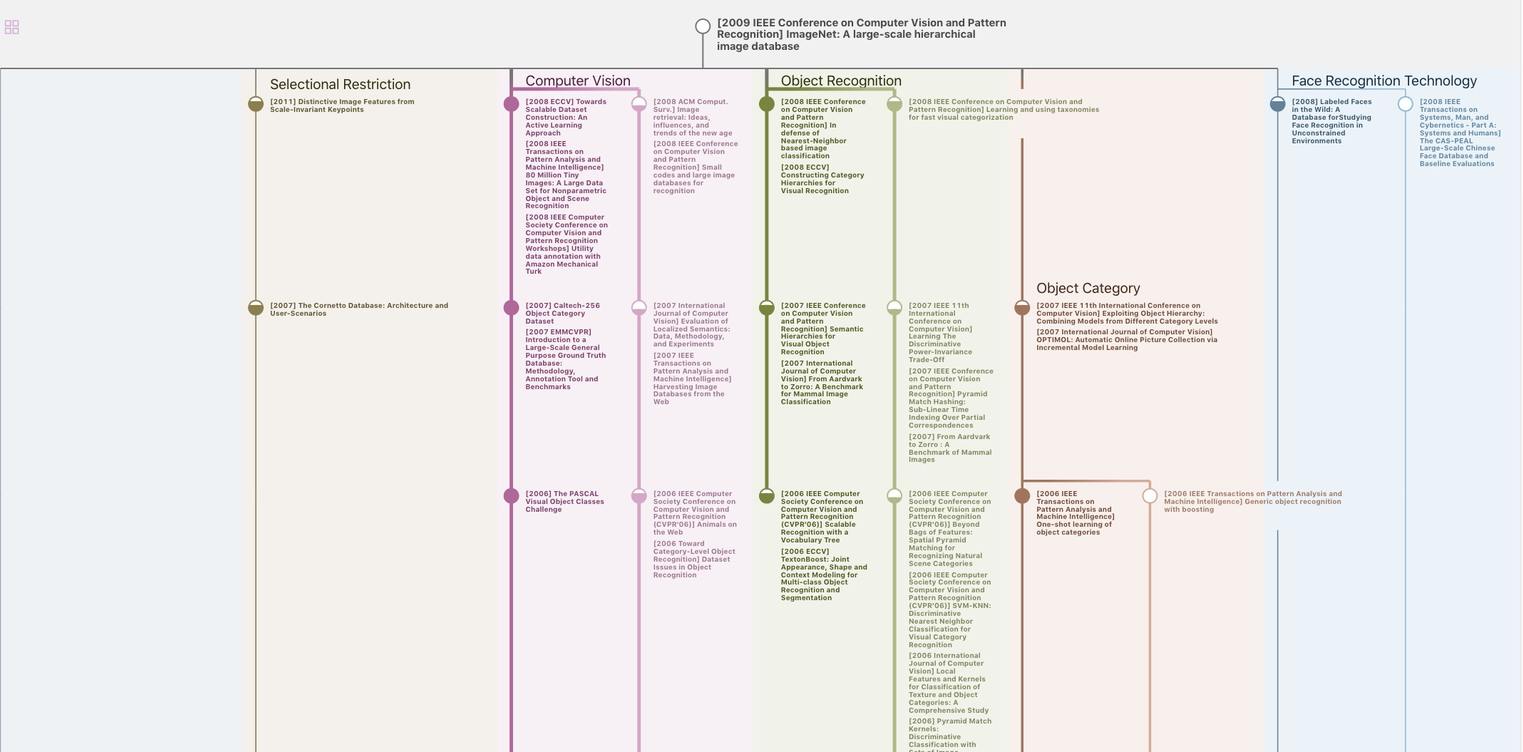
生成溯源树,研究论文发展脉络
Chat Paper
正在生成论文摘要