Deep Reinforcement Learning for Robust VNF Reconfigurations in O-RAN.
IEEE Trans. Netw. Serv. Manag.(2024)
摘要
Open Radio Access Networks (O-RANs) have revolutionized the telecom ecosystem by bringing intelligence into disaggregated RAN and implementing functionalities as Virtual Network Functions (VNF) through open interfaces. However, dynamic traffic conditions in real-life O-RAN environments may require necessary VNF reconfigurations during run-time, which introduce additional overhead costs and traffic instability. To address this challenge, we propose a multi-objective optimization problem that minimizes VNF computational costs and overhead of periodical reconfigurations simultaneously. Our solution uses constrained combinatorial optimization with deep reinforcement learning, where an agent minimizes a penalized cost function calculated by the proposed optimization problem. The evaluation of our proposed solution demonstrates significant enhancements, achieving up to 76% reduction in VNF reconfiguration overhead, with only a slight increase of up to 23% in computational costs. In addition, when compared to the most robust O-RAN system that doesn’t require VNF reconfigurations, which is Centralized RAN (C-RAN), our solution offers up to 76% savings in bandwidth while showing up to 27% overprovisioning of CPU.
更多查看译文
关键词
Radio Access Network (RAN),Open RAN (O-RAN),Constrained Combinatorial Optimization,Deep Reinforcement Learning (DRL)
AI 理解论文
溯源树
样例
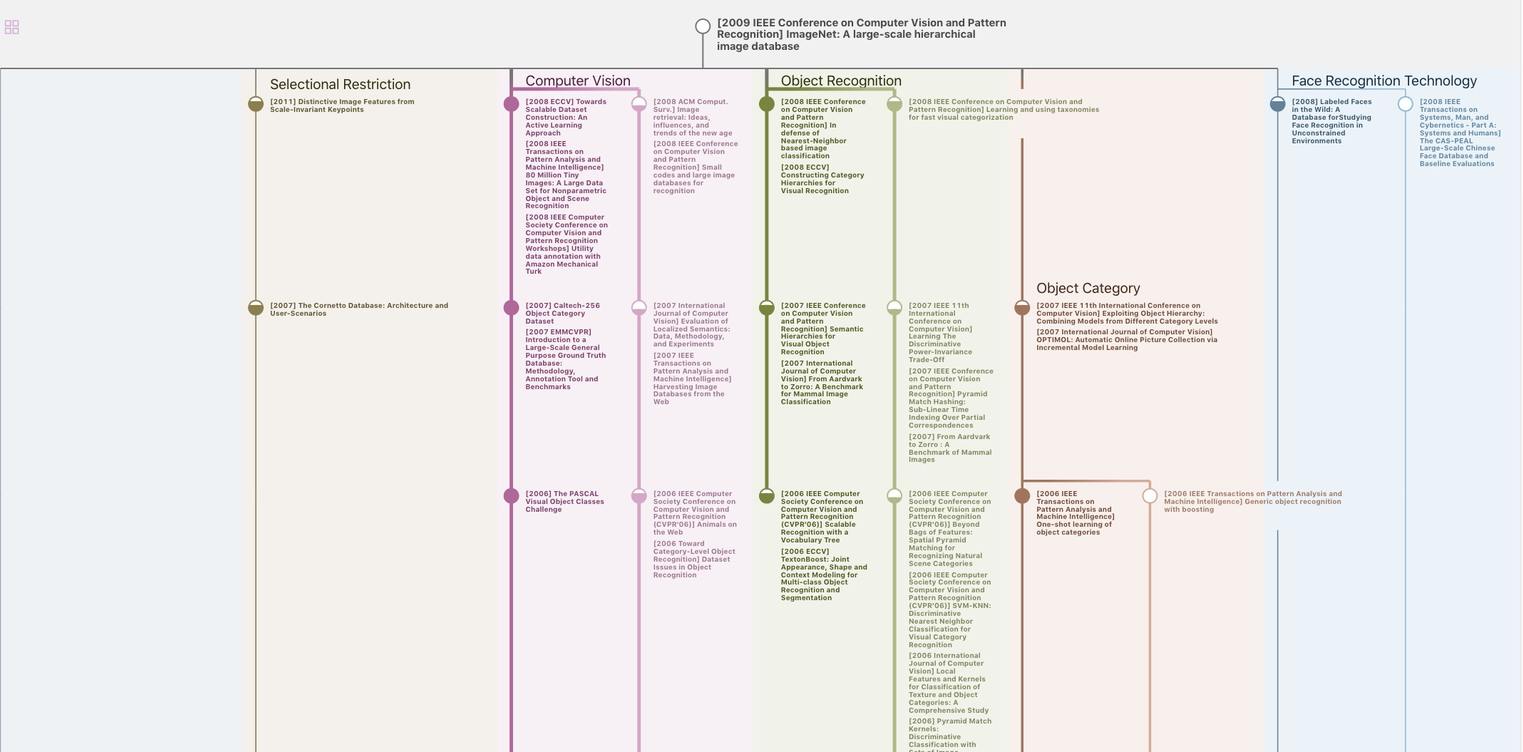
生成溯源树,研究论文发展脉络
Chat Paper
正在生成论文摘要