Regularization and Predictor Selection for Ordinal and Categorical Data
Statistics for social and behavioral sciences(2023)
摘要
Categorical data are quite common in applied statistics, and various regularized fitting procedures have been proposed for appropriate handling of such variables in regression. This chapter gives an overview of some of those techniques. Special emphasis is put on model selection with ordinal explanatory variables if the number of categories and/or the number of predictors is relatively large. In this context, regularized fitting is compared to more classical procedures like forward selection. Structuring of ordinal predictors is considered within the framework of generalized additive models (GAMs), and tools provided within this framework are employed for statistical inference.
更多查看译文
关键词
ordinal,predictor selection,regularization
AI 理解论文
溯源树
样例
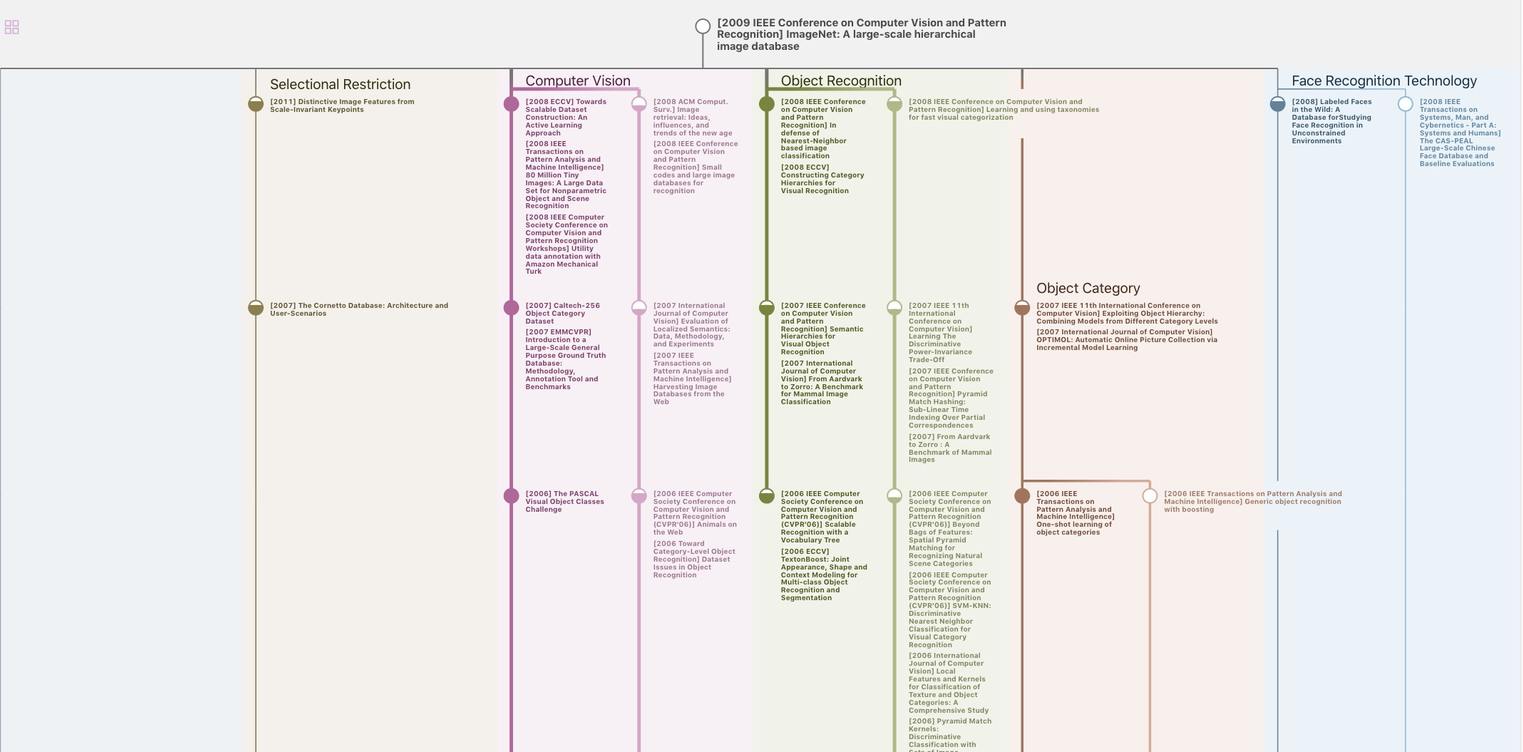
生成溯源树,研究论文发展脉络
Chat Paper
正在生成论文摘要