Unit Correlation With Interactive Feature for Robust and Effective Tracking
IEEE TRANSACTIONS ON MULTIMEDIA(2024)
摘要
For robust and effective tracking, most efforts strive to design a powerful representation target model, while we are inspired by the idea of "knowing oneself and knowing others" to major in both the target and non-target features. In this work, we propose a unit correlation with interactive feature tracker (UCIF), which utilizes feature interaction and independent correlation operation to improve robustness and effectiveness. Specifically, we first propose a feature integration network, in which the feature enhancement module concentrates on enhancing the tracker's representation ability for both target and non-target. The feature interaction module is in charge of completing the interactive learning between target and non-target features. Then, considering the potential risk of blurring spatial information in regular correlation operation, a unit correlation network is presented, where the convolution sampling strategy can integrate the target features as well as reduce the computation costs. The unit kernel for correlation operation can protect the target spatial information. The channel ranking module suppresses background interference via weight assignment. Extensive experiments are conducted on both the short-term and long-term challenging benchmarks, including OTB2015, NFS, UAV123, TrackingNet, GOT-10 k, TLP, LaSOT and VOT-LT2019. Our tracker achieves remarkable performance in robustness and effectiveness.
更多查看译文
关键词
Target tracking,Correlation,Feature extraction,Robustness,Transformers,Visualization,Finite element analysis,Visual object tracking,feature enhancement,feature interaction,convolution sampling,unit correlation
AI 理解论文
溯源树
样例
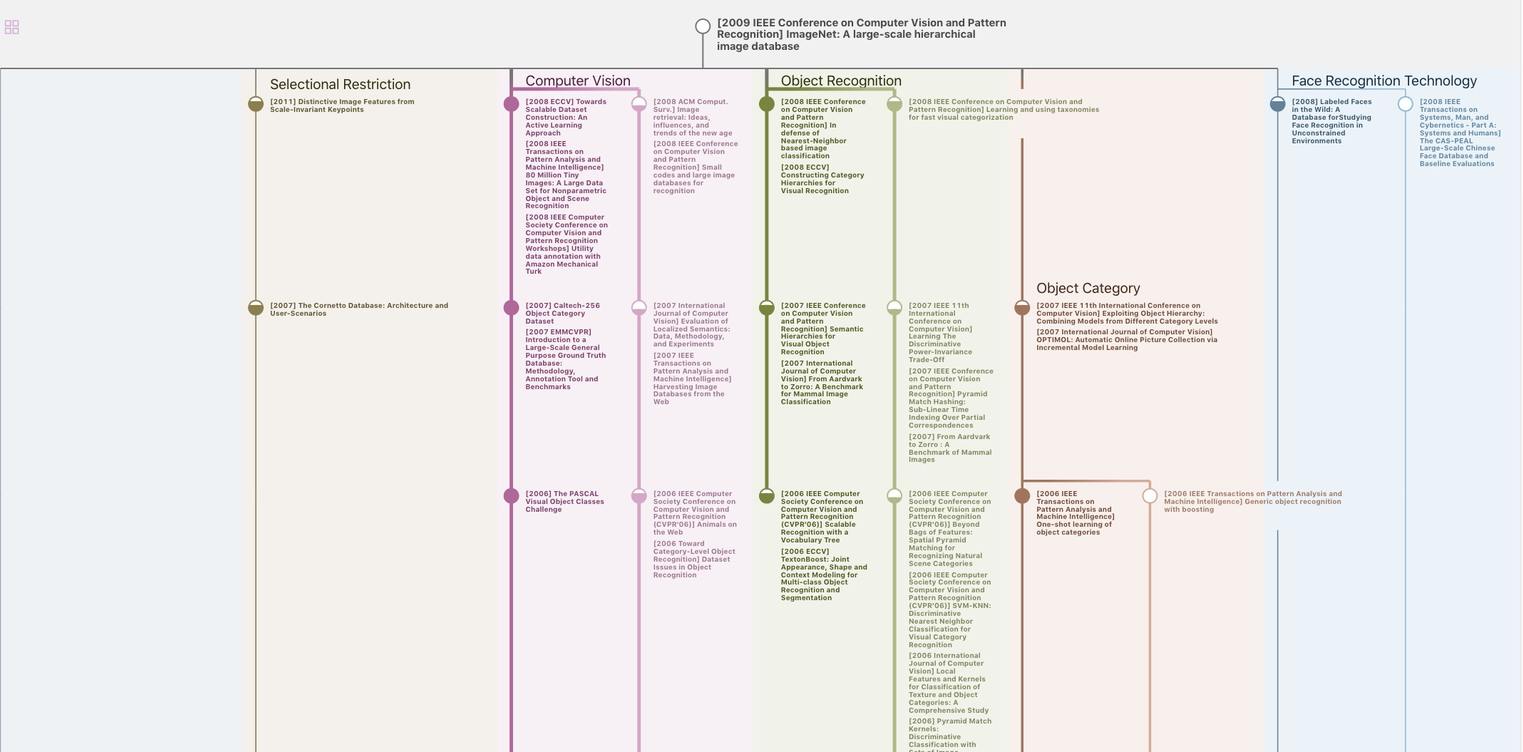
生成溯源树,研究论文发展脉络
Chat Paper
正在生成论文摘要