Friday, June 23, 202310:00 AM - 11:00 AMPP01 Presentation Time: 10:00 AM
Brachytherapy(2023)
摘要
Purpose Brachytherapy treatment planning for cervical cancer is performed real-time while patients are sedated and requires expertise to optimally tailor the radiation to patients’ anatomy. While numerous tools have been developed to increase the efficiency of treatment planning, these methods have not achieved widespread adoption. As a result, many centers are still performing treatment planning manually, which can take 95 minutes on average1. The goal of this work was to automate treatment planning for both intracavitary and hybrid interstitial brachytherapy of cervical cancer using a single neural network model for dose prediction. Materials and Methods The automated planning pipeline takes 3D dose predictions from a neural network model and feeds them into an optimizer, which iteratively determines the dwell times required to reproduce the predicted dose distribution. 907 treatment plans from 273 patients treated with HDR brachytherapy for cervical cancer from 2010 onwards were used to develop the model. The train/test/validation split was 70%/15%/15%, with approximately 49% tandem-and-ovoid (TO), 11% TO with 1-7 needles, 18% tandem-and-ring (TR) and 21% TR with 1-4 needles in each dataset. Model inputs included 4 channels for anatomical masks (high-risk CTV (HRCTV), bladder, rectum and sigmoid), a mask representing the location of digitized dwell positions, and 4 applicator channels (1 for each of tandem, ovoids, ring and needles). Applicator channels were created by mapping a 3D dose kernel to each dwell position within that applicator type (if any) and summing over all dwell positions with equal weighting. A 3D Cascade U-Net architecture2 and a mean squared error (MSE) loss function were used. To convert model dose predictions into deliverable treatment plans, a COBYLA optimizer was used to minimize the MSE between the 3D dose calculated for a given set of dwell times and the predicted dose. Automated plans were produced for 60 test cases (15 for each applicator type). Automated plans were compared to clinical plans using mean absolute differences (MAD) in dose over all voxels and dwell times. HRCTV D90 and organ D2cc were compared with mean differences (MD) and paired t-tests (p value significance threshold 0.013 after Bonferroni correction). Results Figure 1 shows a comparison of automated and clinical plan dose and dwell times for a randomly selected patient from each applicator type. MAD in dose was 32cGy, or 4.6% relative to prescription. MAD in dwell times was 10.2s, or 2.0% relative to total plan dwell time. On average, automated plans had slightly reduced HRCTV D90 (MD 22cGy, or 3.2% relative to prescription, p=0.02), rectal D2cc (MD 8cGy or 1.1%, p=0.3) and sigmoid D2cc (MD 25cGy or 3.6%, p<0.001). Bladder D2cc averaged 11cGy (1.6%) higher for automated plans (p=0.1). Qualitatively, the shape of automated dose distributions matched those of clinical plans (e.g. pear-shaped dose for intracavitary cases) and dwell time weighting between applicator types was acceptable (e.g. reduced dwell times within needles). Automated plans were produced in around 3 minutes, which includes the time to produce inputs (2 min), 20s to run model predictions and 25s for optimization. Conclusions Automated planning with neural network dose predictions produced treatment plans with significantly lower sigmoid dose and otherwise similar dose distributions and metrics as clinically treated plans, in under 3 minutes. Automated plans could be further manually optimized if necessary, but with far less time. The major time savings and standardization across practitioners, regardless of experience, brings value to the brachytherapy treatment planning process. References: 1Michaud et al. Brachytherapy 2016;15(5):578-83. 2Liu et al. Med. Phys. 2021;48(9):5574-82. Brachytherapy treatment planning for cervical cancer is performed real-time while patients are sedated and requires expertise to optimally tailor the radiation to patients’ anatomy. While numerous tools have been developed to increase the efficiency of treatment planning, these methods have not achieved widespread adoption. As a result, many centers are still performing treatment planning manually, which can take 95 minutes on average1. The goal of this work was to automate treatment planning for both intracavitary and hybrid interstitial brachytherapy of cervical cancer using a single neural network model for dose prediction. The automated planning pipeline takes 3D dose predictions from a neural network model and feeds them into an optimizer, which iteratively determines the dwell times required to reproduce the predicted dose distribution. 907 treatment plans from 273 patients treated with HDR brachytherapy for cervical cancer from 2010 onwards were used to develop the model. The train/test/validation split was 70%/15%/15%, with approximately 49% tandem-and-ovoid (TO), 11% TO with 1-7 needles, 18% tandem-and-ring (TR) and 21% TR with 1-4 needles in each dataset. Model inputs included 4 channels for anatomical masks (high-risk CTV (HRCTV), bladder, rectum and sigmoid), a mask representing the location of digitized dwell positions, and 4 applicator channels (1 for each of tandem, ovoids, ring and needles). Applicator channels were created by mapping a 3D dose kernel to each dwell position within that applicator type (if any) and summing over all dwell positions with equal weighting. A 3D Cascade U-Net architecture2 and a mean squared error (MSE) loss function were used. To convert model dose predictions into deliverable treatment plans, a COBYLA optimizer was used to minimize the MSE between the 3D dose calculated for a given set of dwell times and the predicted dose. Automated plans were produced for 60 test cases (15 for each applicator type). Automated plans were compared to clinical plans using mean absolute differences (MAD) in dose over all voxels and dwell times. HRCTV D90 and organ D2cc were compared with mean differences (MD) and paired t-tests (p value significance threshold 0.013 after Bonferroni correction). Figure 1 shows a comparison of automated and clinical plan dose and dwell times for a randomly selected patient from each applicator type. MAD in dose was 32cGy, or 4.6% relative to prescription. MAD in dwell times was 10.2s, or 2.0% relative to total plan dwell time. On average, automated plans had slightly reduced HRCTV D90 (MD 22cGy, or 3.2% relative to prescription, p=0.02), rectal D2cc (MD 8cGy or 1.1%, p=0.3) and sigmoid D2cc (MD 25cGy or 3.6%, p<0.001). Bladder D2cc averaged 11cGy (1.6%) higher for automated plans (p=0.1). Qualitatively, the shape of automated dose distributions matched those of clinical plans (e.g. pear-shaped dose for intracavitary cases) and dwell time weighting between applicator types was acceptable (e.g. reduced dwell times within needles). Automated plans were produced in around 3 minutes, which includes the time to produce inputs (2 min), 20s to run model predictions and 25s for optimization. Automated planning with neural network dose predictions produced treatment plans with significantly lower sigmoid dose and otherwise similar dose distributions and metrics as clinically treated plans, in under 3 minutes. Automated plans could be further manually optimized if necessary, but with far less time. The major time savings and standardization across practitioners, regardless of experience, brings value to the brachytherapy treatment planning process. References: 1Michaud et al. Brachytherapy 2016;15(5):578-83. 2Liu et al. Med. Phys. 2021;48(9):5574-82.
更多查看译文
关键词
presentation time,friday,june
AI 理解论文
溯源树
样例
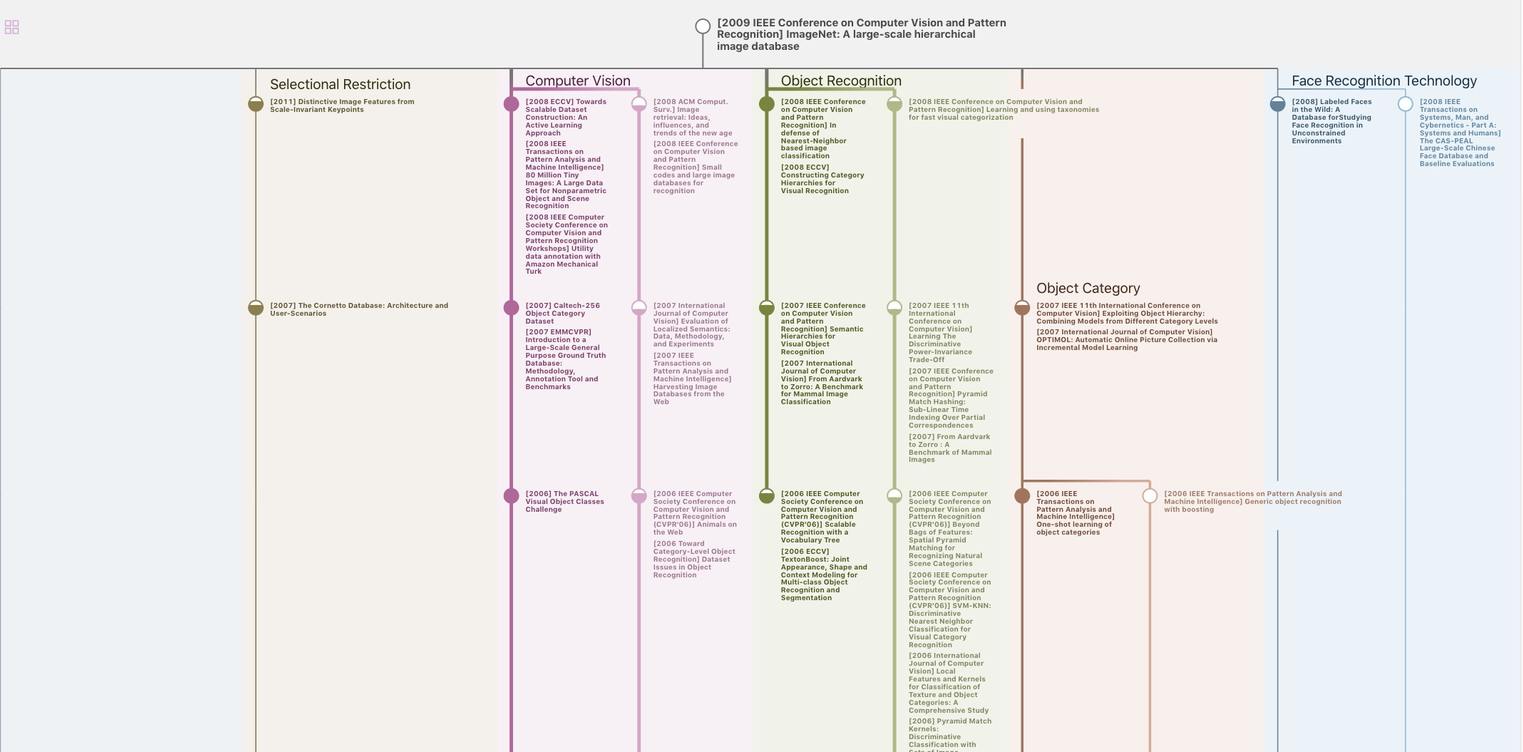
生成溯源树,研究论文发展脉络
Chat Paper
正在生成论文摘要