Low-Latency Hierarchical Federated Learning in Wireless Edge Networks.
IEEE Internet Things J.(2024)
摘要
Hierarchical federated learning (HFL) has recently emerged as a more practical machine learning paradigm, which enables edge servers (ESs) in close proximity to conduct partial model aggregation. Despite its utility, local training and model aggregation incur considerable computation and communication time. Client selection (CS) has proven effective for minimizing latency. However, CS faces the following challenges in HFL. First, the accessible clients, computation resources and network bandwidth are time-varying and unpredictable. Second, certain dynamics can only be observed after the decisions are made. Third, multiple ESs face different unknown clients, increasing the difficulty of selecting clients in an online manner. Last, resource usage may be excessively violated during the training process. Existing HFL researches are insufficient to tackle these challenges. This work proposes a multi-ESs CS framework (MCS), which is based on multi-armed bandit technique. MCS aims to reduce the cumulative computation and communication time, using two algorithms: i) an online learning-based CS algorithm (OCA) makes the CS decisions for each ES, based on empirical learning results; ii) a randomized rounding algorithm (RRA) converts fractional decisions obtained by OCA into binary solutions. Theoretically, MCS can enjoy the sub-linear regret and violation compared to the optimal strategy. Practically, extensive experiments on real-world datasets demonstrate the empirical superiority of MCS over multiple state-of-the-art algorithms in minimizing cumulative latency.
更多查看译文
关键词
hierarchical federated learning,client selection,online learning,multi-armed bandit,constraint violation
AI 理解论文
溯源树
样例
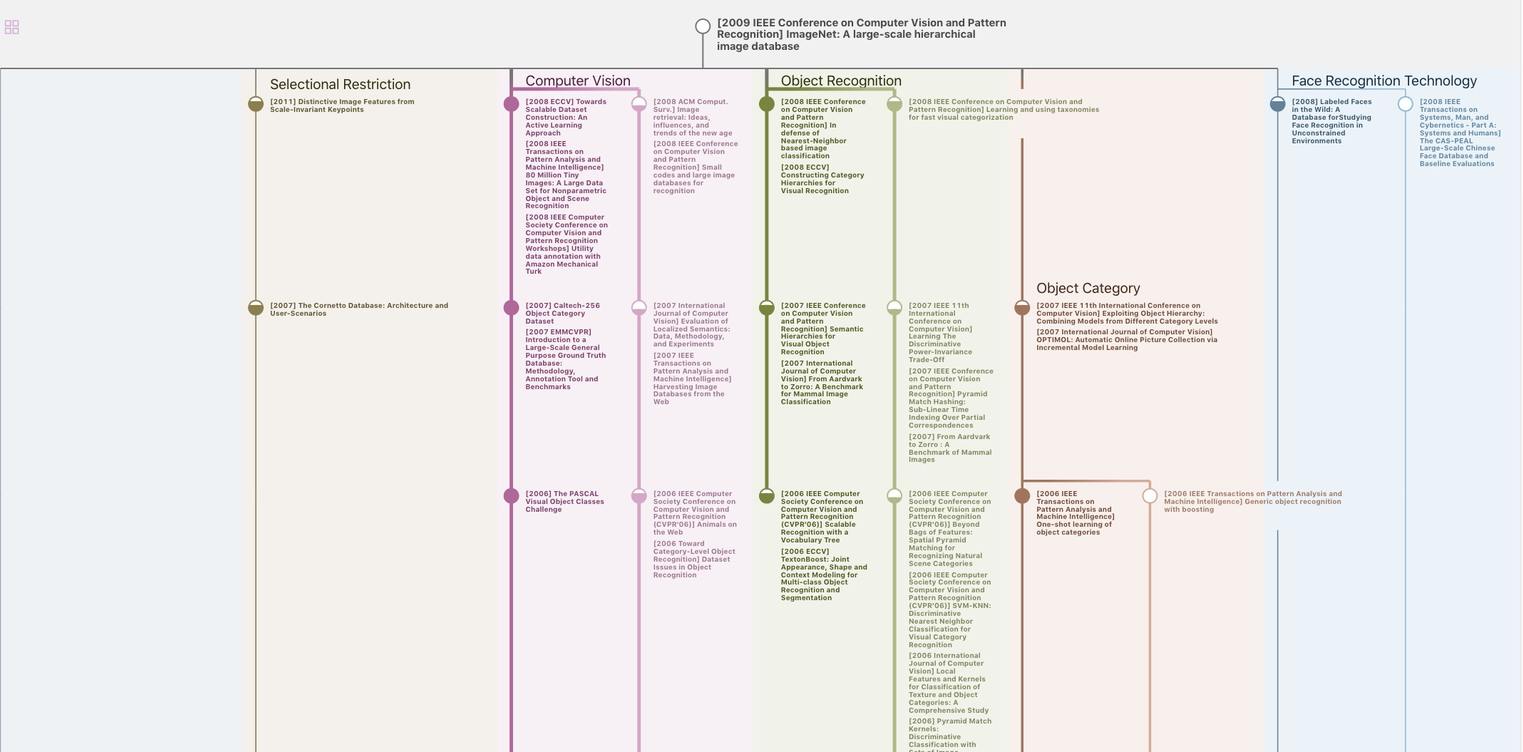
生成溯源树,研究论文发展脉络
Chat Paper
正在生成论文摘要