Deep learning neural network -guided detection of asbestos bodies in bronchoalveolar lavage samples
Acta Cytologica(2023)
摘要
Introduction: Asbestos is a global occupational health hazard, and exposure to it by inhalation predisposes to interstitial as well as malignant pulmonary morbidity. Over time, asbestos fibers embedded in lung tissue can become coated with iron-rich proteins and mucopolysaccharides, after which they are called asbestos bodies (ABs) and can be detected in light microscopy (LM). Bronchoalveolar lavage, a cytological sample from the lower airways, is one of the methods for diagnosing lung asbestosis and related morbidity. Search for ABs in these samples is generally laborious and time-consuming. We describe a novel diagnostic method, which implements deep learning neural network technology for the detection of ABs in bronchoalveolar lavage samples (BALs). Methods: BALs with suspicion of asbestos exposure were scanned as whole slide images (WSIs) and uploaded to a cloud-based virtual microscopy platform with a neural network training interface. The images were used for training and testing a neural network model capable of recognizing ABs. To prioritize the model’s sensitivity, we allowed it to also make false-positive suggestions. To test the model, we compared its performance to standard LM diagnostic data as well as the ground truth (GT) number of ABs, which we established by a thorough manual search of the WSIs. Results: We were able to reach overall sensitivity of 93.4% (95% CI: 90.3–95.7%) in the detection of ABs in comparison to their GT number. Compared to standard LM diagnostic data, our model showed equal to or higher sensitivity in most cases. Conclusion: Our results indicate that deep learning neural network technology offers promising diagnostic tools for routine assessment of BALs. However, at this stage, a human expert is required to confirm the findings.
更多查看译文
关键词
asbestos bodies,neural network
AI 理解论文
溯源树
样例
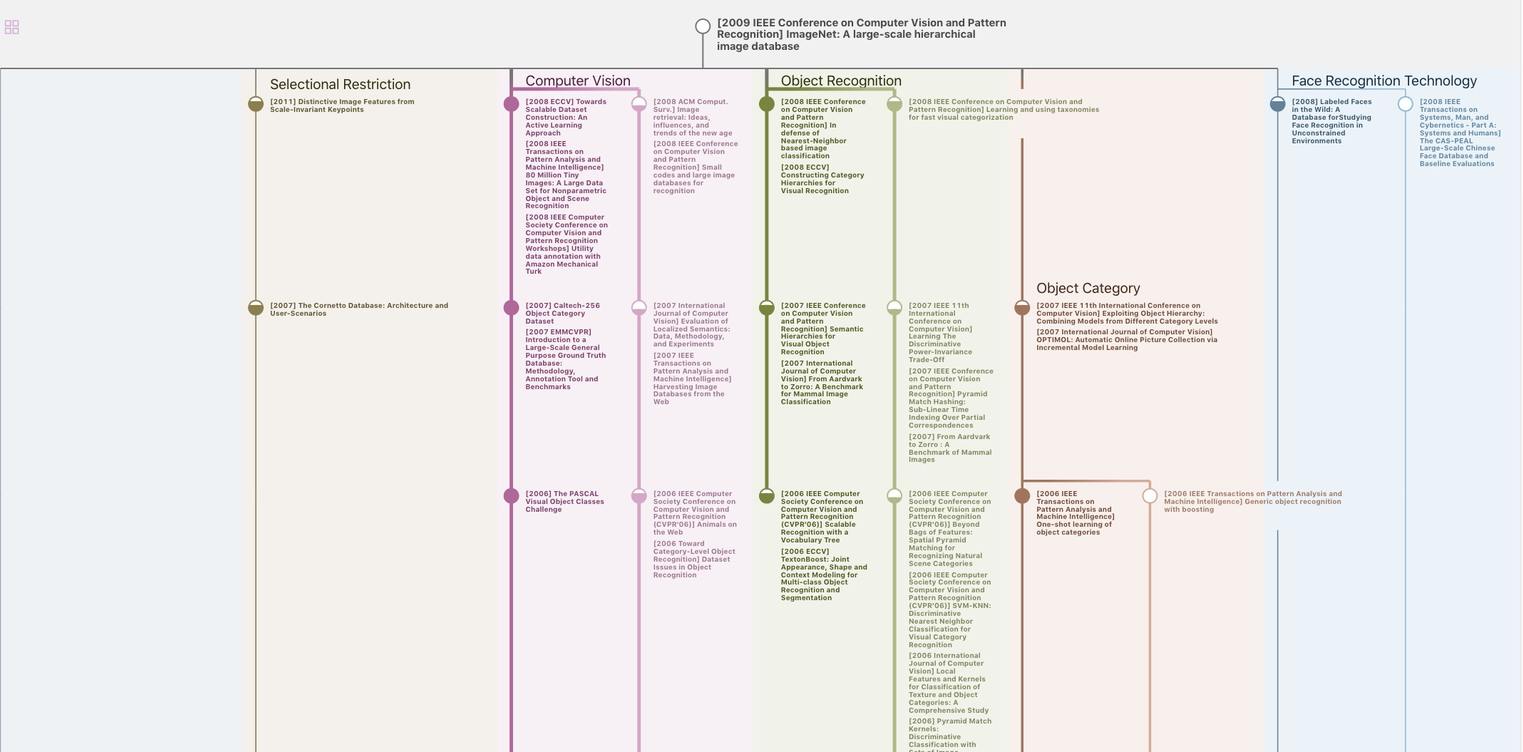
生成溯源树,研究论文发展脉络
Chat Paper
正在生成论文摘要