Aligning Before Aggregating: Enabling Communication Efficient Cross-Domain Federated Learning via Consistent Feature Extraction.
IEEE Trans. Mob. Comput.(2024)
摘要
Cross-domain federated learning (FL), where data on local clients come from different domains, is a common case of FL. In such a cross-domain case, features extracted from the raw data of different clients deviate from each other in the feature space, leading to a so-called feature shift. This phenomenon can reduce feature discrimination and degrade the performance of the learned model. However, most existing FL methods are not specifically designed for the cross-domain setting. In this paper, we propose a novel cross-domain FL method named AlignFed. In AlignFed, each client model consists of a personalized feature extractor and a shared lightweight classifier. The feature extractor maps the features to a consistent space by aligning them to identical global target points. Inspired by recent studies in contrastive learning, AlignFed regards points that are uniformly distributed on the hypersphere as global target points. It then pushes features toward global target points of their corresponding classes and away from those of other classes to improve feature discrimination. The shared classifier aggregates knowledge across clients over the consistent feature space, which can mitigate performance degradation caused by feature shift while reducing communication cost. We conduct convergence analysis and perform extensive experiments to evaluate AlignFed.
更多查看译文
关键词
Federated Learning,Cross-Domain,Feature Alignment,Communication Cost
AI 理解论文
溯源树
样例
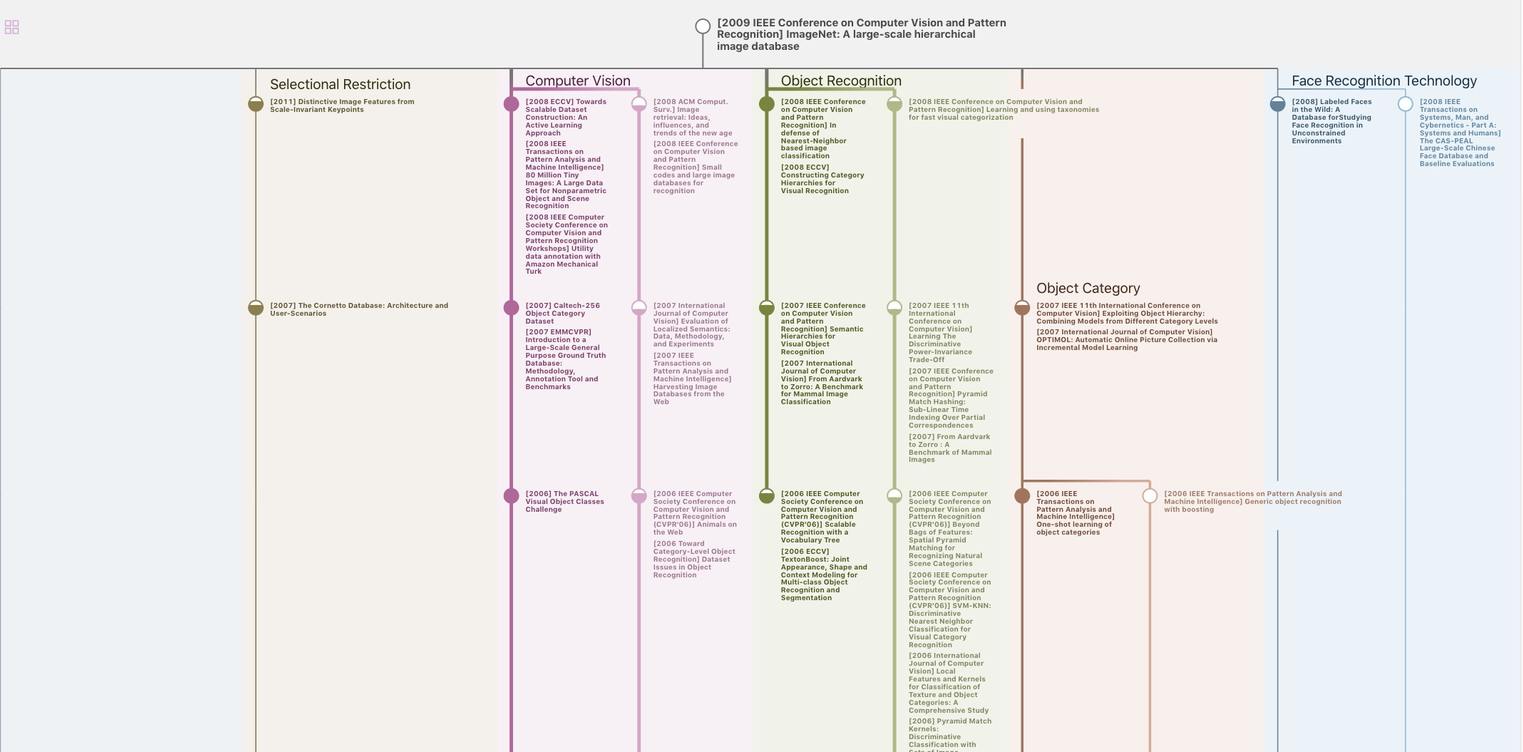
生成溯源树,研究论文发展脉络
Chat Paper
正在生成论文摘要