Prospective validation of a dynamic prognostic model for identifying COVID ‐19 patients at high risk of rapid deterioration
Pharmacoepidemiology and Drug Safety(2022)
摘要
We sought to develop and prospectively validate a dynamic model that incorporates changes in biomarkers to predict rapid clinical deterioration in patients hospitalized for COVID-19.We established a retrospective cohort of hospitalized patients aged ≥18 years with laboratory-confirmed COVID-19 using electronic health records (EHR) from a large integrated care delivery network in Massachusetts including >40 facilities from March to November 2020. A total of 71 factors, including time-varying vital signs and laboratory findings during hospitalization were screened. We used elastic net regression and tree-based scan statistics for variable selection to predict rapid deterioration, defined as progression by two levels of a published severity scale in the next 24 h. The development cohort included the first 70% of patients identified chronologically in calendar time; the latter 30% served as the validation cohort. A cut-off point was estimated to alert clinicians of high risk of imminent clinical deterioration.Overall, 3706 patients (2587 in the development and 1119 in the validation cohort) met the eligibility criteria with a median of 6 days of follow-up. Twenty-four variables were selected in the final model, including 16 dynamic changes of laboratory results or vital signs. Area under the ROC curve was 0.81 (95% CI, 0.79-0.82) in the development set and 0.74 (95% CI, 0.71-0.78) in the validation set. The model was well calibrated (slope = 0.84 and intercept = -0.07 on the calibration plot in the validation set). The estimated cut-off point, with a positive predictive value of 83%, was 0.78.Our prospectively validated dynamic prognostic model demonstrated temporal generalizability in a rapidly evolving pandemic and can be used to inform day-to-day treatment and resource allocation decisions based on dynamic changes in biophysiological factors.
更多查看译文
关键词
dynamic prognostic model,<scp>covid</scp>
AI 理解论文
溯源树
样例
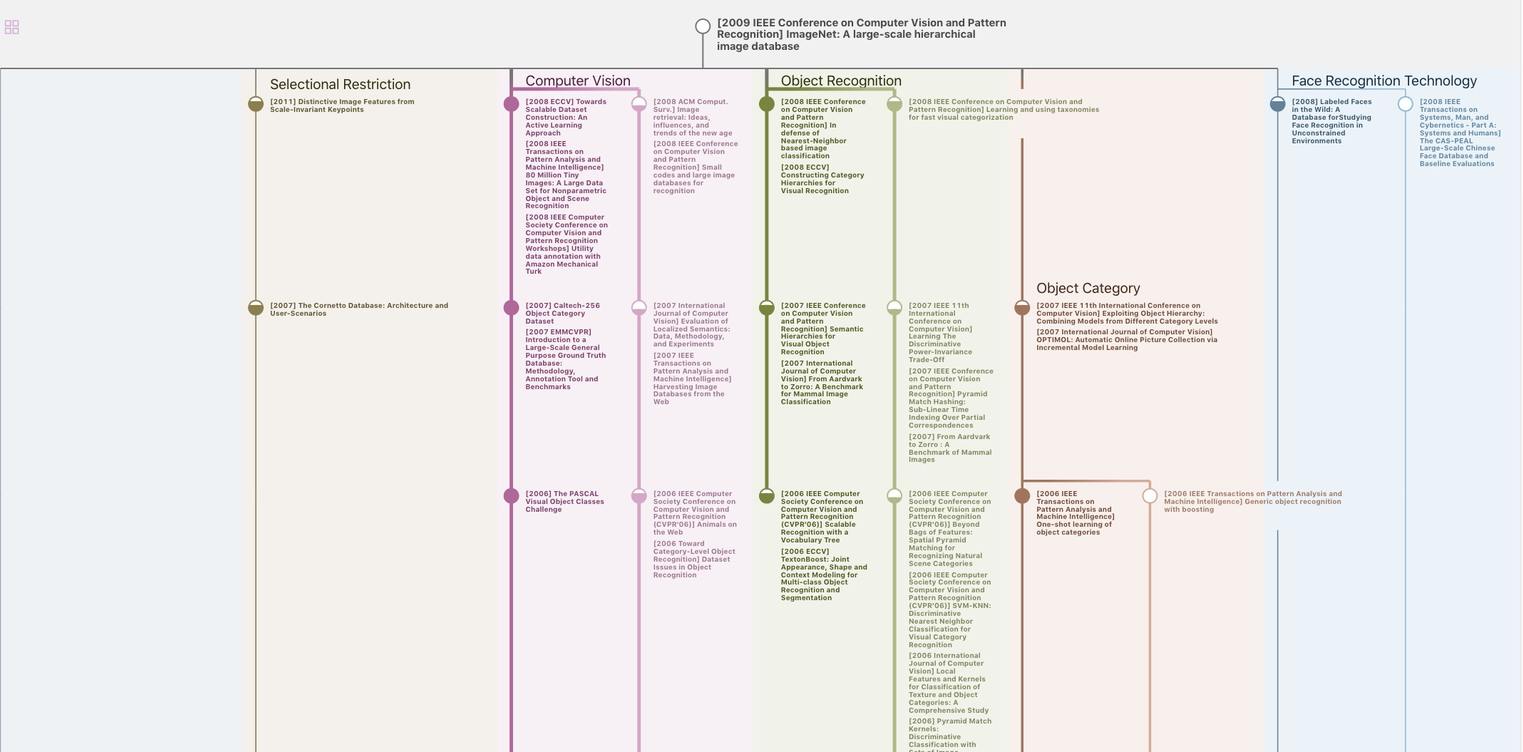
生成溯源树,研究论文发展脉络
Chat Paper
正在生成论文摘要