FED-CD: Federated Causal Discovery from Interventional and Observational Data
arXiv (Cornell University)(2022)
摘要
Existing causal discovery methods typically require the data to be available in a centralized location. However, many practical domains, such as healthcare, limit access to the data gathered by local entities, primarily for privacy and regulatory constraints. To address this, we propose FED-CD, a federated framework for inferring causal structures from distributed datasets containing observational and interventional data. By exchanging updates instead of data samples, FED-CD ensures privacy while enabling decentralized discovery of the underlying directed acyclic graph (DAG). We accommodate scenarios with shared or disjoint intervened covariates, and mitigate the adverse effects of interventional data heterogeneity. We provide empirical evidence for the performance and scalability of FED-CD for decentralized causal discovery using synthetic and real-world DAGs.
更多查看译文
关键词
federated causal discovery,observational
data,interventional
AI 理解论文
溯源树
样例
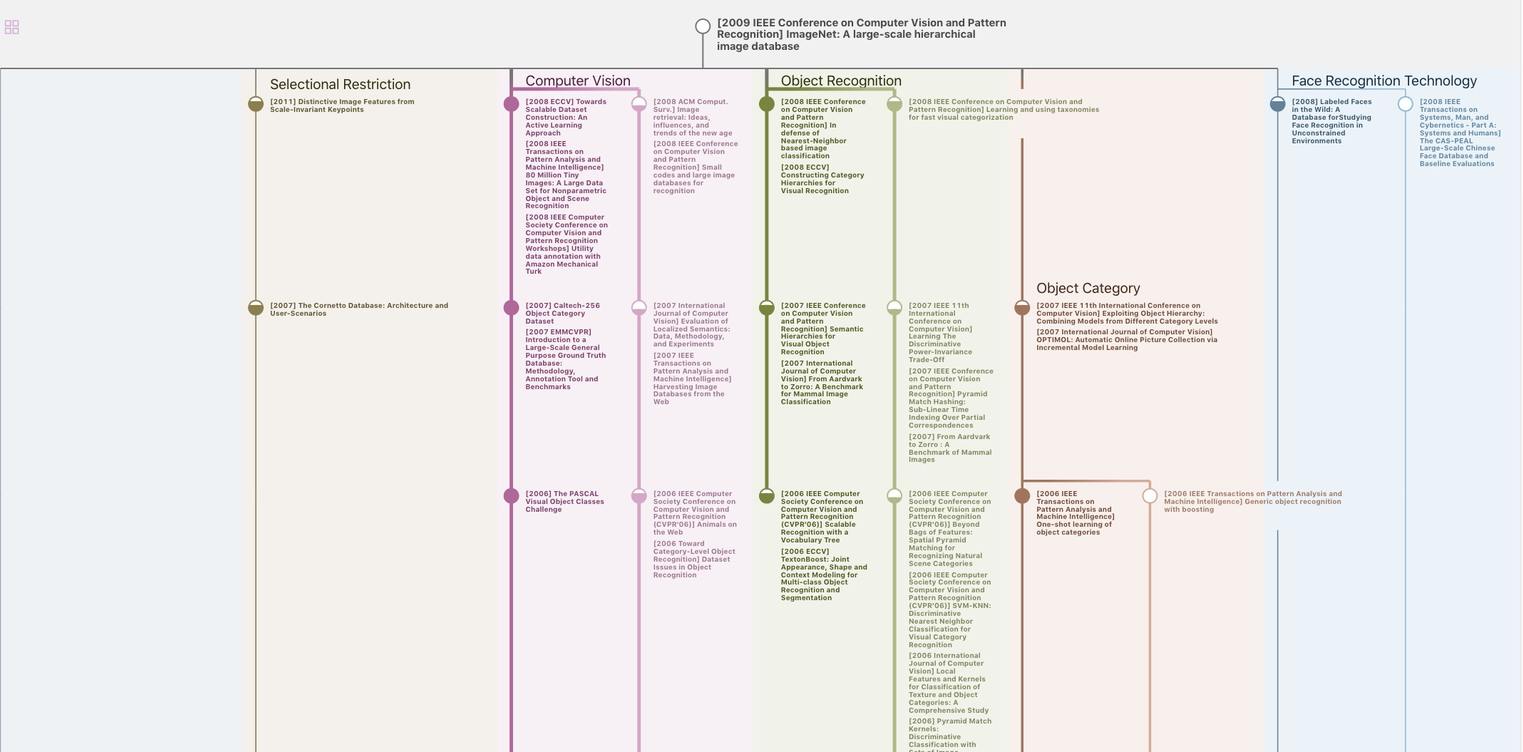
生成溯源树,研究论文发展脉络
Chat Paper
正在生成论文摘要