Multi-Agent Deep Reinforcement Learning for Mix-mode Runway Sequencing
2022 IEEE 25th International Conference on Intelligent Transportation Systems (ITSC)(2022)
摘要
In mixed-mode operation, arrivals and departures are allowed to land and depart on the same runway. An appropriate strategy from air traffic controllers for arrivals and departures sequencing would boost the runway through-put significantly. On the other hand, safety is still the most crucial feature in the operation. Therefore, to assist air traffic controllers to make decisions on departures and arrivals with efficient utilization of runway capacity and safe operations, this paper proposed a Multi-agent Deep Reinforcement Learning approach using Multi-agent Deep Deterministic Policy Gradient to train two agents simultaneously: departure agent, and arrival agent. The departure agent makes departure slotting decisions for departures while the arrival agent determines the time delay or spacing decision on the arrival stream. A data-driven simulation environment is developed using Singapore Changi Airport data to support the learning process. Besides, a random sampling technique is also introduced to reduce redundant samples and increase off-policy sample efficiency. Moreover, the impact of different reward functions on runway throughput is also investigated and two specific models, e.g., ‘arrival priority’ and ‘departure priority’, are selected for further analysis in this study. As the result, by comparing the trained models with the ad-hoc model, the proposed approach increases the runway throughput significantly, with the highest 12.8% additional departure releases and overall 5.3% additional departure releases in identical environments while safety separations are maintained.
更多查看译文
关键词
deep reinforcement learning,reinforcement learning,multi-agent,mix-mode
AI 理解论文
溯源树
样例
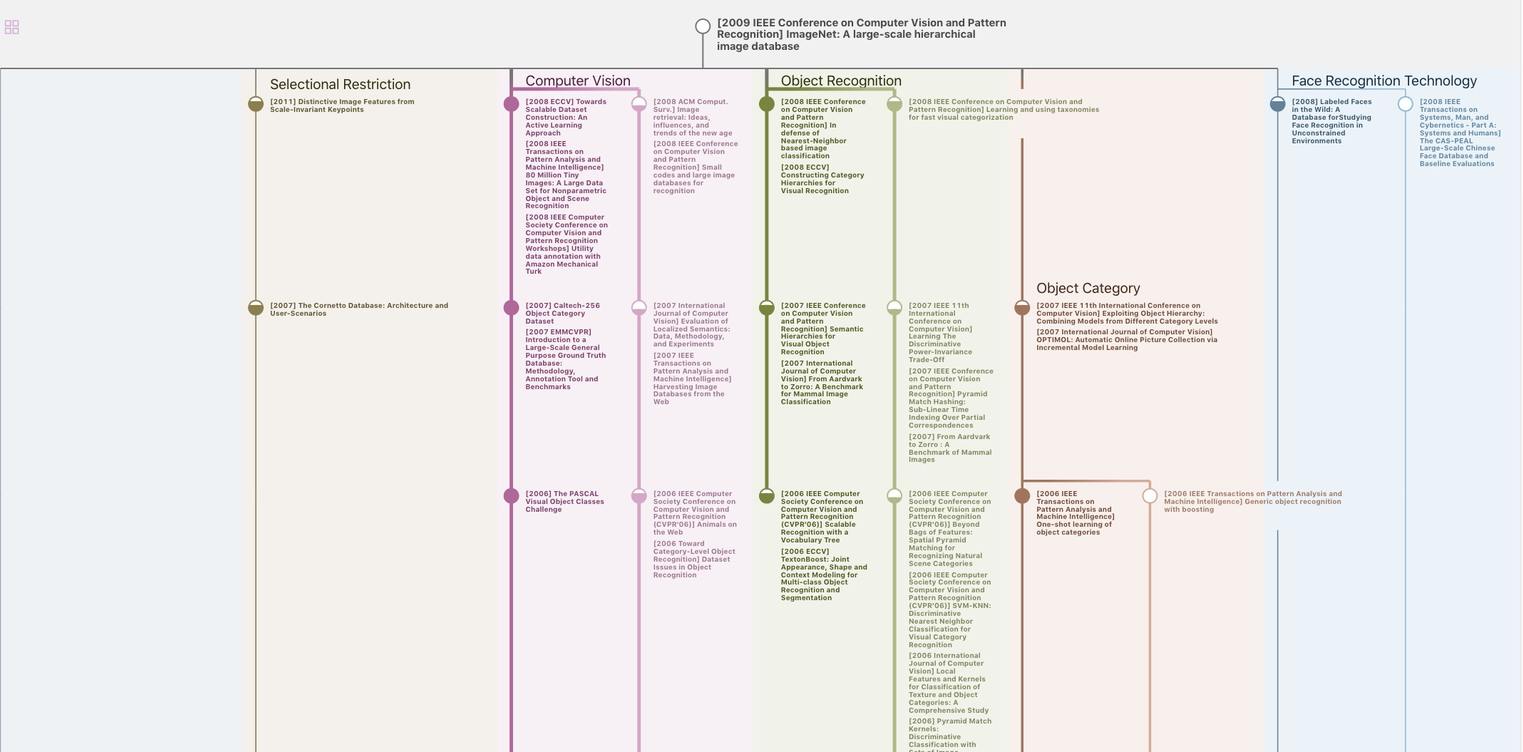
生成溯源树,研究论文发展脉络
Chat Paper
正在生成论文摘要