Mechanism design for public projects via three machine learning based approaches
Autonomous Agents and Multi-Agent Systems(2024)
摘要
We study mechanism design for nonexcludable and excludable binary public project problems. Our aim is to maximize the expected number of consumers and the expected agents’ welfare. We first show that for the nonexcludable public project model, there is no need for machine learning based mechanism design. We identify a sufficient condition on the prior distribution for the existing conservative equal costs mechanism to be the optimal strategy-proof and individually rational mechanism. For general distributions, we propose a dynamic program that solves for the optimal mechanism. For the excludable public project model, we identify a similar sufficient condition for the existing serial cost sharing mechanism to be optimal for 2 and 3 agents. We derive a numerical upper bound and use it to show that for several common distributions, the serial cost sharing mechanism is close to optimality. The serial cost sharing mechanism is not optimal in general. We propose three machine learning based approaches for designing better performing mechanisms. We focus on the family of largest unanimous mechanisms, which characterizes all strategy-proof and individually rational mechanisms for the excludable public project model. A largest unanimous mechanism describes an iterative mechanism, which is defined by an exponential number of mechanism parameters. Our first approach describes the largest unanimous mechanism family using a neural network and training is carried out by minimizing a cost function that combines the mechanism design objective and the constraint violation penalty. We interpret the largest unanimous mechanisms as price-oriented rationing-free (PORF) mechanisms, which enables us to move the mechanisms’ iterative decision making off the neural network, to a separate simulation process, therefore avoiding the vanishing gradient problem. We also feed the prior distribution’s analytical form into the cost function to achieve high-quality gradients for efficient training. Our second approach treats the mechanism design task as a Markov Decision Process with an exponential number of states. During the Markov decision process, the non-consumers are gradually removed from the system. We train multiple neural networks, each for a different number of remaining agents, to learn the optimal value function on the states. Training is carried out by supervised learning toward a set of manually prepared base cases and the Bellman equation. Our third approach is based on reinforcement learning for a Partially Observable Markov Decision Process. Each RL episode randomly draws a type profile, which is hidden from the RL agent (mechanism designer). The RL agent only observes which cost share offers have been accepted under the largest unanimous mechanism under discussion. We use a continuous action space reinforcement learning approach to adjust the offer policy (i.e., adjust mechanism parameters). Lastly, our first two approaches use “supervision to manual mechanisms” as a systematic way for network initialization, which is potentially valuable for machine learning based mechanism design in general.
更多查看译文
关键词
Public Project,Cost Sharing,Automated Mechanism Design,Mechanism Design via Neural Networks,Mechanism Design via Reinforcement Learning
AI 理解论文
溯源树
样例
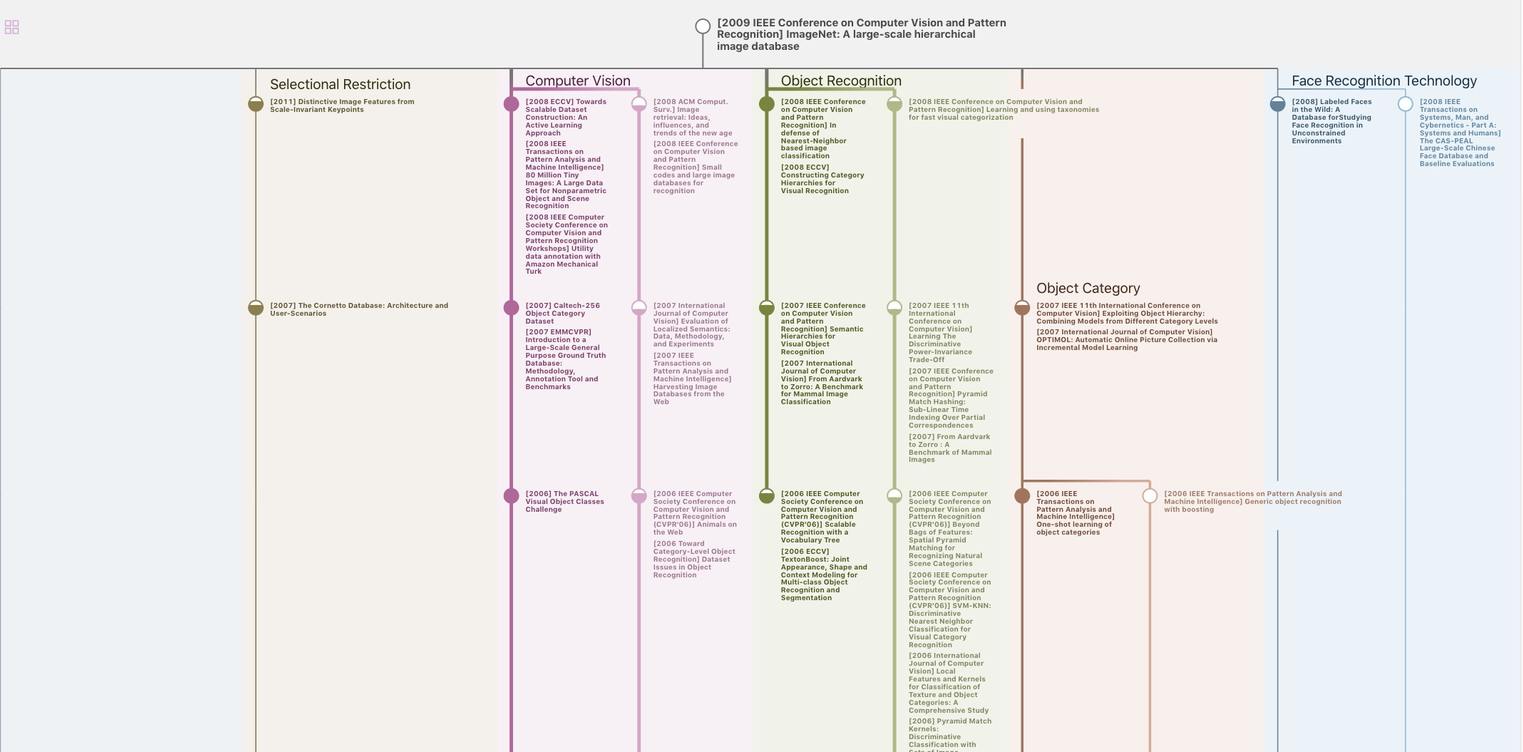
生成溯源树,研究论文发展脉络
Chat Paper
正在生成论文摘要