Boosting Sensitivity of Large-scale Online Experimentation via Dropout Buyer Imputation
arXiv (Cornell University)(2022)
摘要
In online experimentation, appropriate metrics (e.g., purchase) provide strong evidence to support hypotheses and enhance the decision-making process. However, incomplete metrics are frequently occurred in the online experimentation, making the available data to be much fewer than the planned online experiments (e.g., A/B testing). In this work, we introduce the concept of dropout buyers and categorize users with incomplete metric values into two groups: visitors and dropout buyers. For the analysis of incomplete metrics, we propose a clustering-based imputation method using $k$-nearest neighbors. Our proposed imputation method considers both the experiment-specific features and users' activities along their shopping paths, allowing different imputation values for different users. To facilitate efficient imputation of large-scale data sets in online experimentation, the proposed method uses a combination of stratification and clustering. The performance of the proposed method is compared to several conventional methods in both simulation studies and a real online experiment at eBay.
更多查看译文
关键词
buyer imputation,dropout
AI 理解论文
溯源树
样例
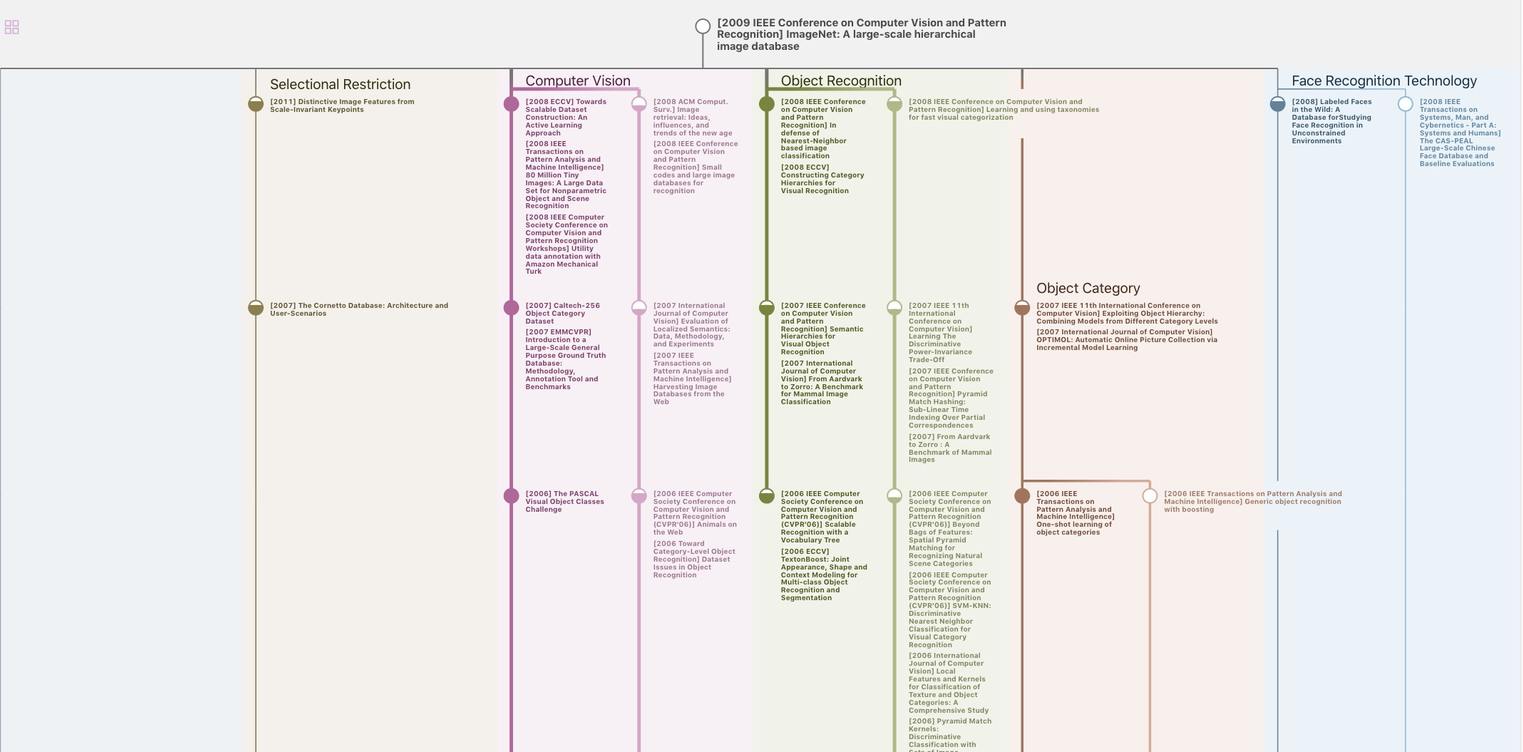
生成溯源树,研究论文发展脉络
Chat Paper
正在生成论文摘要