InFi
Proceedings of the 28th Annual International Conference on Mobile Computing And Networking(2022)
摘要
Mobile-centric AI applications put forward high requirements for resource-efficiency of model inference. Input filtering is a promising approach to eliminate the redundancy in the input so as to reduce the cost of inference. Previous efforts have tailored effective solutions for many applications, but left two essential questions unanswered: (1) theoretical filterability of an inference workload to guide the application of input filtering techniques, thereby avoiding the trial-and-error cost for resource-constrained mobile applications; (2) robust discriminability of feature embedding to allow input filtering to be widely effective for diverse inference tasks and input content. To answer these questions, we first provide a generic formalization of the input filtering problem and theoretically compare the hypothesis complexity of inference models and their input filters to understand the optimization potential of applying input filtering. Then we propose the first end-to-end learnable input filtering framework that covers most state-of-the-art methods and surpasses them in feature embedding with robust discriminability. Based on our framework, we design and implement an input filtering system InFi supporting six input modalities. InFi is the first to support text and sensor signal inputs and model partitioning deployments widely adopted by under-resourced mobile systems. Comprehensive evaluations confirm our theoretical results and show that InFi outperforms strong baselines in applicability, accuracy, and efficiency, owing to its generality and end-to-end learnability. InFi can achieve 8.5X throughput and save 95% bandwidth, while keeping over 90% accuracy, for a video analytics app on mobile platforms.
更多查看译文
关键词
infi
AI 理解论文
溯源树
样例
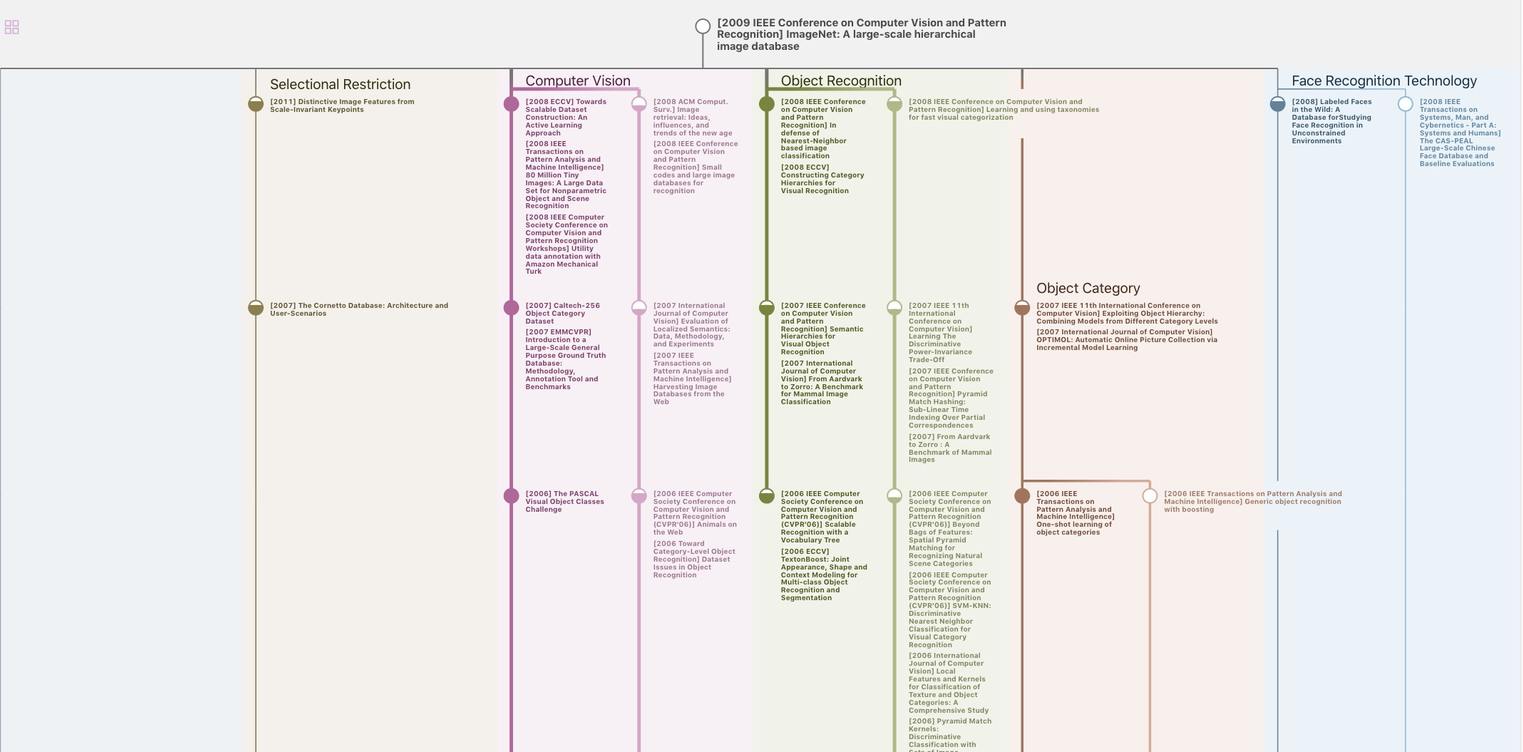
生成溯源树,研究论文发展脉络
Chat Paper
正在生成论文摘要