GLite
Proceedings of the 59th ACM/IEEE Design Automation Conference(2022)
摘要
We propose a scalable graph-level optimizer named GLite to speed up search-based optimizations on large neural networks. GLite leverages a potential-based partitioning strategy to partition large computation graphs into small subgraphs without losing profitable substitution patterns. To avoid redundant subgraph matching, we propose a dynamic programming algorithm to reuse explored matching patterns. The experimental results show that GLite reduces the running time of search-based optimizations from hours to milliseconds, without compromising in inference performance.
更多查看译文
AI 理解论文
溯源树
样例
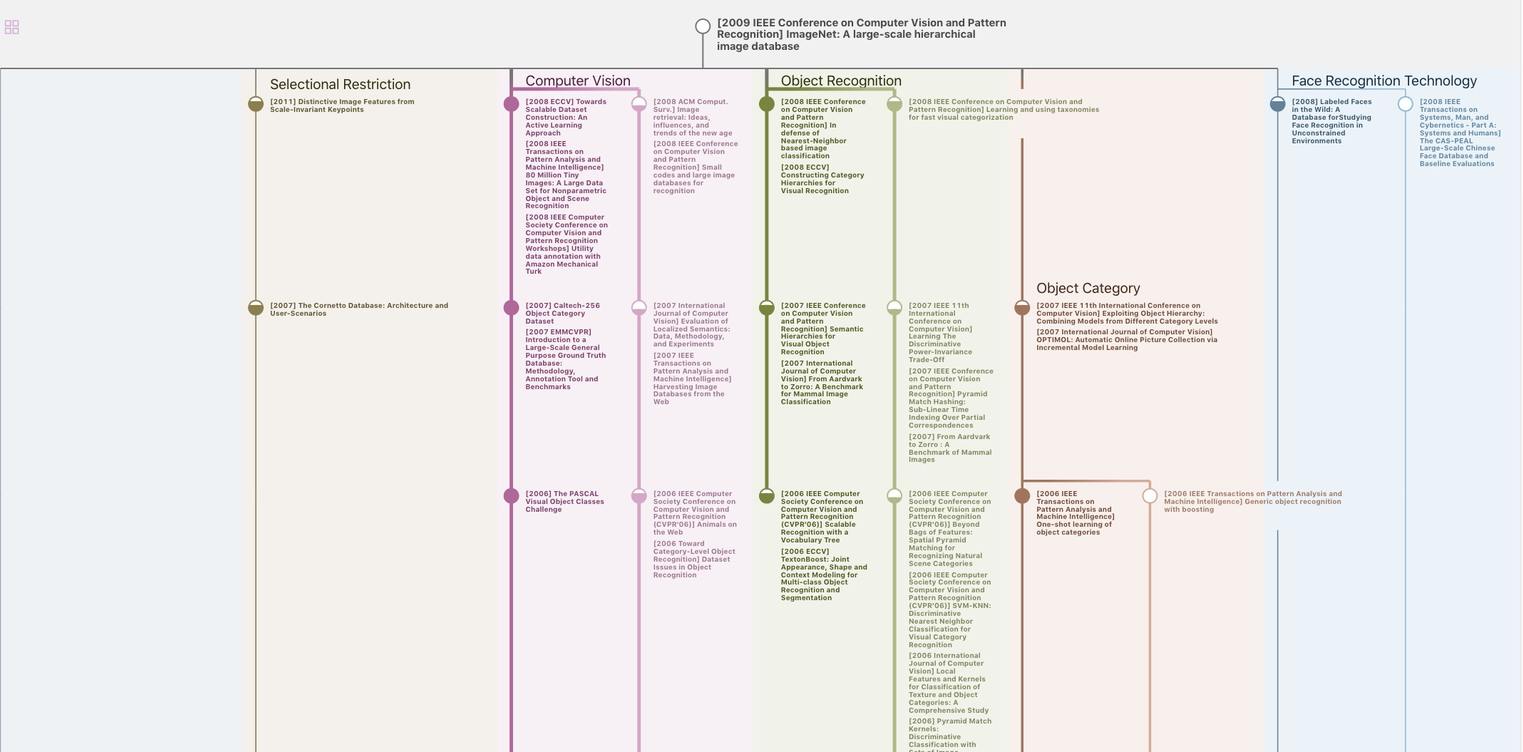
生成溯源树,研究论文发展脉络
Chat Paper
正在生成论文摘要