Robust Fine-Tuning of Deep Neural Networks with Hessian-based Generalization Guarantees
arXiv (Cornell University)(2022)
摘要
We consider fine-tuning a pretrained deep neural network on a target task. We study the generalization properties of fine-tuning to understand the problem of overfitting, which has often been observed (e.g., when the target dataset is small or when the training labels are noisy). Existing generalization measures for deep networks depend on notions such as distance from the initialization (i.e., the pretrained network) of the fine-tuned model and noise stability properties of deep networks. This paper identifies a Hessian-based distance measure through PAC-Bayesian analysis, which is shown to correlate well with observed generalization gaps of fine-tuned models. Theoretically, we prove Hessian distance-based generalization bounds for fine-tuned models. We also describe an extended study of fine-tuning against label noise, where overfitting is against a critical problem; We present an algorithm and a generalization error guarantee for this algorithm under a class conditional independent noise model. Empirically, we observe that the Hessian-based distance measure can match the scale of the observed generalization gap of fine-tuned models in practice. We also test our algorithm on several image classification tasks with noisy training labels, showing notable gains over prior methods, and the Hessian distance measure of the fine-tuned model decreases substantially.
更多查看译文
关键词
deep neural networks,neural networks,robust,fine-tuning,hessian-based
AI 理解论文
溯源树
样例
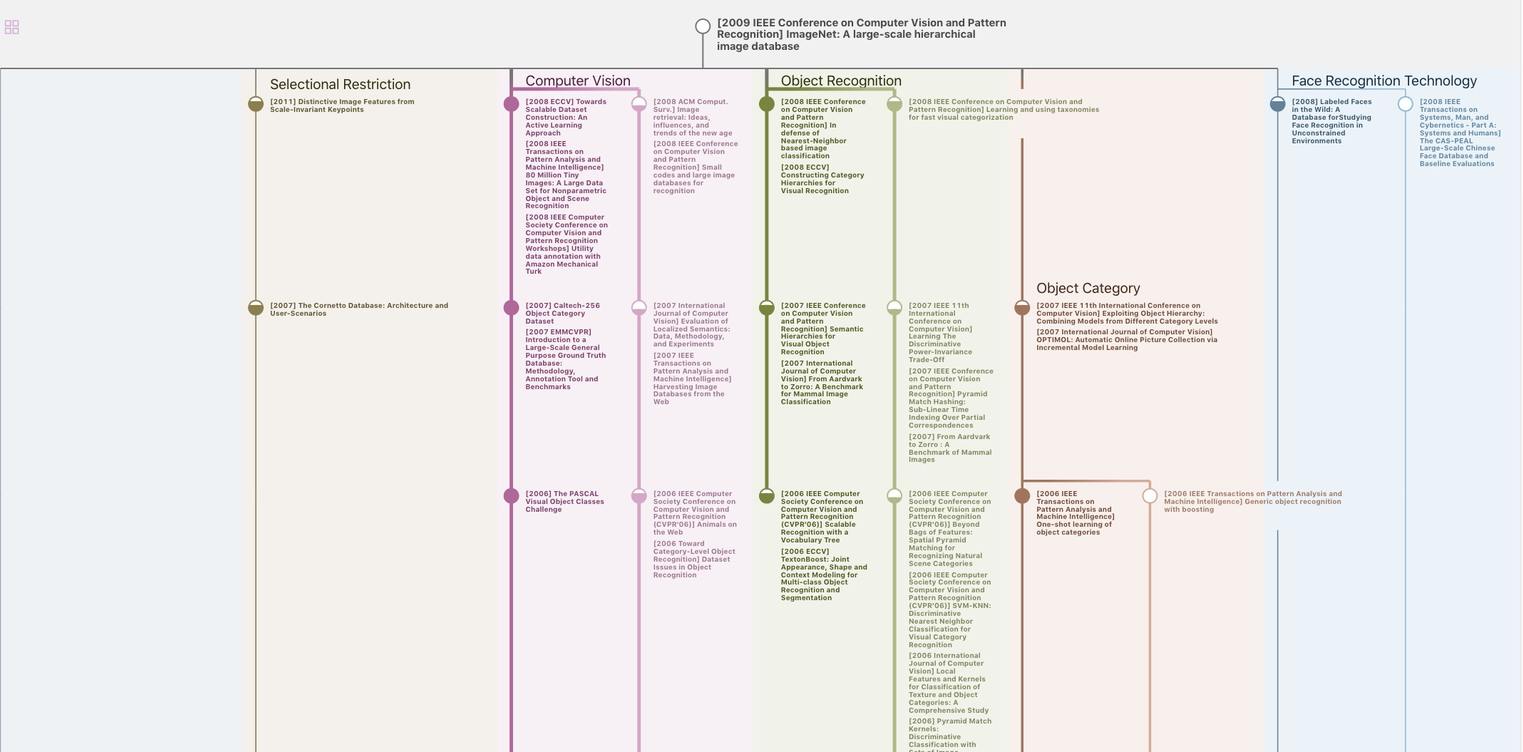
生成溯源树,研究论文发展脉络
Chat Paper
正在生成论文摘要