Stacking Designs: Designing Multifidelity Computer Experiments with Target Predictive Accuracy
arXiv (Cornell University)(2024)
摘要
In an era where scientific experiments can be very costly, multi-fidelity emulators provide a useful tool for cost-efficient predictive scientific computing. For scientific applications, the experimenter is often limited by a tight computational budget, and thus wishes to (i) maximize predictive power of the multi-fidelity emulator via a careful design of experiments, and (ii) ensure this model achieves a desired error tolerance with some notion of confidence. Existing design methods, however, do not jointly tackle objectives (i) and (ii). We propose a novel stacking design approach that addresses both goals. A multi-level reproducing kernel Hilbert space (RKHS) interpolator is first introduced to build the emulator, under which our stacking design provides a sequential approach for designing multi-fidelity runs such that a desired prediction error of $\epsilon > 0$ is met under regularity assumptions. We then prove a novel cost complexity theorem that, under this multi-level interpolator, establishes a bound on the computation cost (for training data simulation) needed to achieve a prediction bound of $\epsilon$. This result provides novel insights on conditions under which the proposed multi-fidelity approach improves upon a conventional RKHS interpolator which relies on a single fidelity level. Finally, we demonstrate the effectiveness of stacking designs in a suite of simulation experiments and an application to finite element analysis.
更多查看译文
关键词
experiments,designs,accuracy,predictive,target
AI 理解论文
溯源树
样例
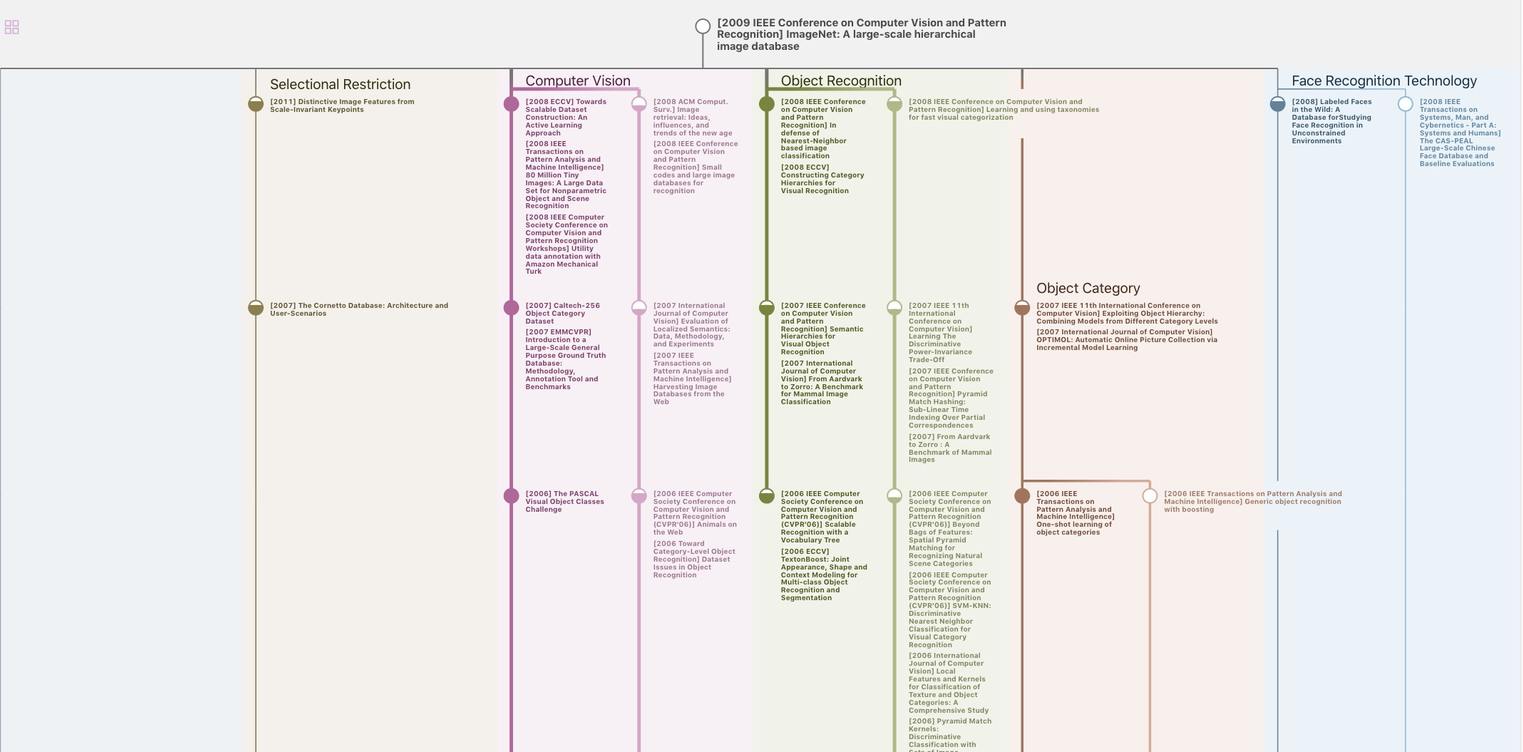
生成溯源树,研究论文发展脉络
Chat Paper
正在生成论文摘要